PROV-FL: Privacy-preserving Round Optimal Verifiable Federated Learning.
Workshop on Security and Artificial Intelligence (AISec)(2022)
摘要
Federated learning is a distributed framework where a server computes a global model by aggregating the local models trained on users' private data. However, for a stronger data privacy guarantee, the server should not access the local models except the aggregated one. One way to achieve this is to use a secure aggregation protocol that comes with the cost of several rounds of interactions between the server and users in the absence of a fully trusted third party (TTP). In this paper, we present PROV-FL, an efficient privacy-preserving federated learning training system that securely aggregates users' local models. PROV-FL requires only one round of communication between the server and users for aggregating local models without a TTP. Based on the homomorphic encryption and differential privacy techniques, we develop two PROV-FL training protocols for two different, namely single and multi-aggregator, scenarios. PROV-FL enjoys the verifiability feature in which the server can verify the authenticity of the aggregated model and efficiently handles users' dynamic joining and leaving. We evaluate and compare the performance of PROV-FL by running experiments on training CNN/DNN models with a diverse set of real-world datasets.
更多查看译文
AI 理解论文
溯源树
样例
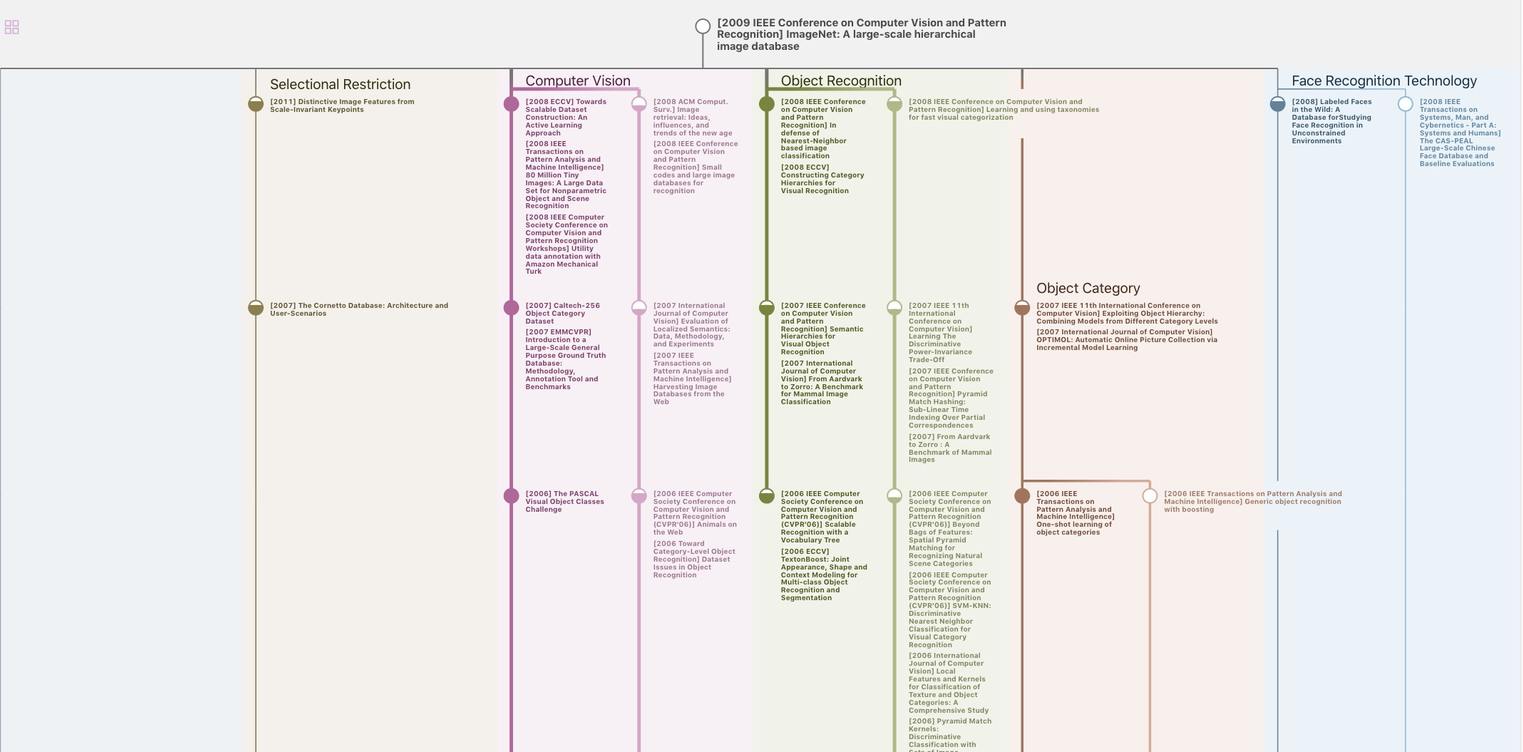
生成溯源树,研究论文发展脉络
Chat Paper
正在生成论文摘要