Leveraging Spectral Representations of Control Flow Graphs for Efficient Analysis of Windows Malware.
ACM Asia Conference on Computer and Communications Security (AsiaCCS)(2022)
摘要
The rapid pace of malware development and the widespread use of code obfuscation, polymorphism, and morphing techniques pose a considerable challenge to detecting and analyzing malware. Today, it is difficult for antivirus applications to use traditional signature-based detection methods to detect morphing malware. Thus, the emergence of structure graph-based detection methods has become a hope to solve this challenge. In this work, we propose a method for detecting malware using graphs' spectral heat and wave signatures, which are efficient and size- and permutation-invariant. We extracted 250 and 1,000 heat and wave representations, and we trained and tested heat and wave representations on eight machine learning classifiers. We used a dataset of 37,537 unpacked Windows malware executables and extracted the control flow graph (CFG) of each windows malware to obtain the spectral representations. Our experimental results showed that by using heat and wave spectral graph theory, the best malware analysis accuracy reached 95.9%.
更多查看译文
关键词
Malware Detection, Spectral Representation, Windows Executable Binaries, Size-invariant Representations
AI 理解论文
溯源树
样例
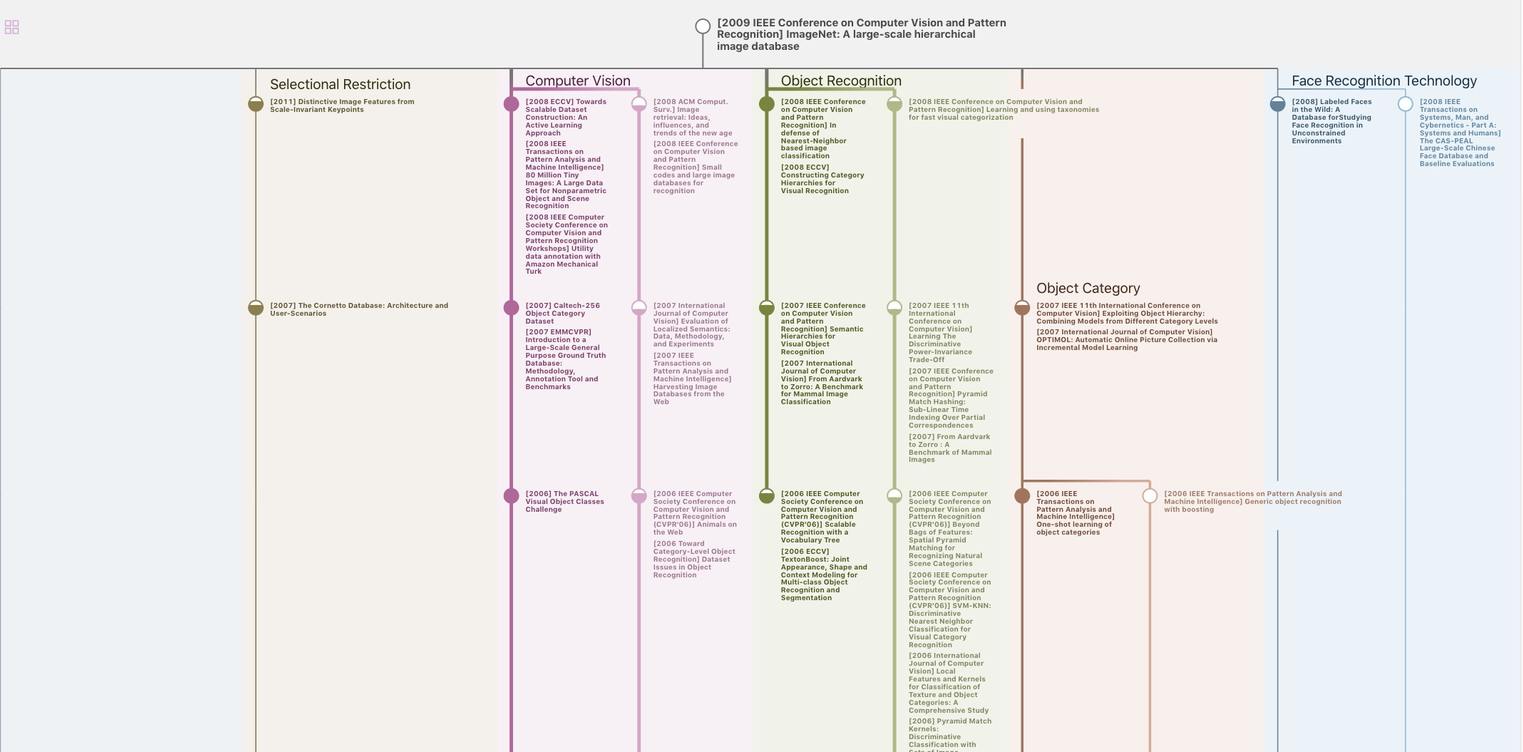
生成溯源树,研究论文发展脉络
Chat Paper
正在生成论文摘要