Depth, Breadth, and Complexity: Ways to Attack and Defend Deep Learning Models.
ACM Asia Conference on Computer and Communications Security (AsiaCCS)(2022)
摘要
Deep Learning is rapidly evolving to the point that it can be used in crucial safety and security applications, including self-driving vehicles, surveillance, drones, and robots. However, these deep learning models are vulnerable to attacks based on adversarial samples that are undetectable to the human eye but cause the model to misbehave. There is an increasing demand for comprehensive and in-depth analysis of behaviors of various attacks and the possible defenses against common deep learning models under several adversarial scenarios. In this study, we conducted four separate investigations. First, we examine the relationship between the model's complexity and its robustness against the studied attacks. Second, the connection between the performance and diversity of models is examined. Third, the first and second experiments were tested across different datasets to explore the impact of the dataset on the performance of the model. Four, throughout the defense strategies, the model behavior is extensively investigated. The code, trained models, and detailed settings and results are available at: https://github.com/InfoLab-SKKU/ML-Adversarial-Attacks-Analysis.
更多查看译文
关键词
Deep Learning, Adversarial Attacks, Defenses, Computer Vision
AI 理解论文
溯源树
样例
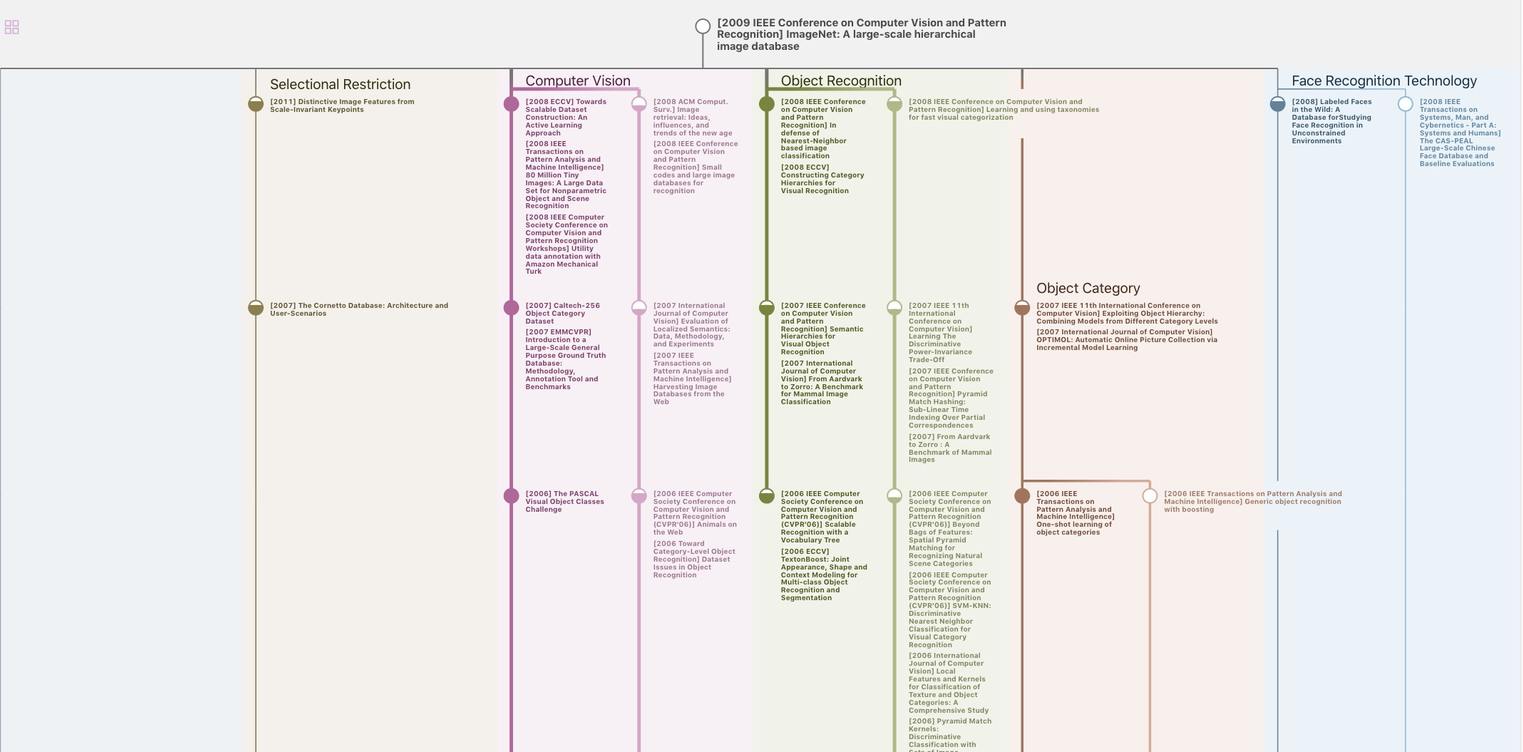
生成溯源树,研究论文发展脉络
Chat Paper
正在生成论文摘要