Robust Consensus Clustering and its Applications for Advertising Forecasting
arxiv(2022)
摘要
Consensus clustering aggregates partitions in order to find a better fit by reconciling clustering results from different sources/executions. In practice, there exist noise and outliers in clustering task, which, however, may significantly degrade the performance. To address this issue, we propose a novel algorithm -- robust consensus clustering that can find common ground truth among experts' opinions, which tends to be minimally affected by the bias caused by the outliers. In particular, we formalize the robust consensus clustering problem as a constraint optimization problem, and then derive an effective algorithm upon alternating direction method of multipliers (ADMM) with rigorous convergence guarantee. Our method outperforms the baselines on benchmarks. We apply the proposed method to the real-world advertising campaign segmentation and forecasting tasks using the proposed consensus clustering results based on the similarity computed via Kolmogorov-Smirnov Statistics. The accurate clustering result is helpful for building the advertiser profiles so as to perform the forecasting.
更多查看译文
关键词
robust consensus clustering,advertising
AI 理解论文
溯源树
样例
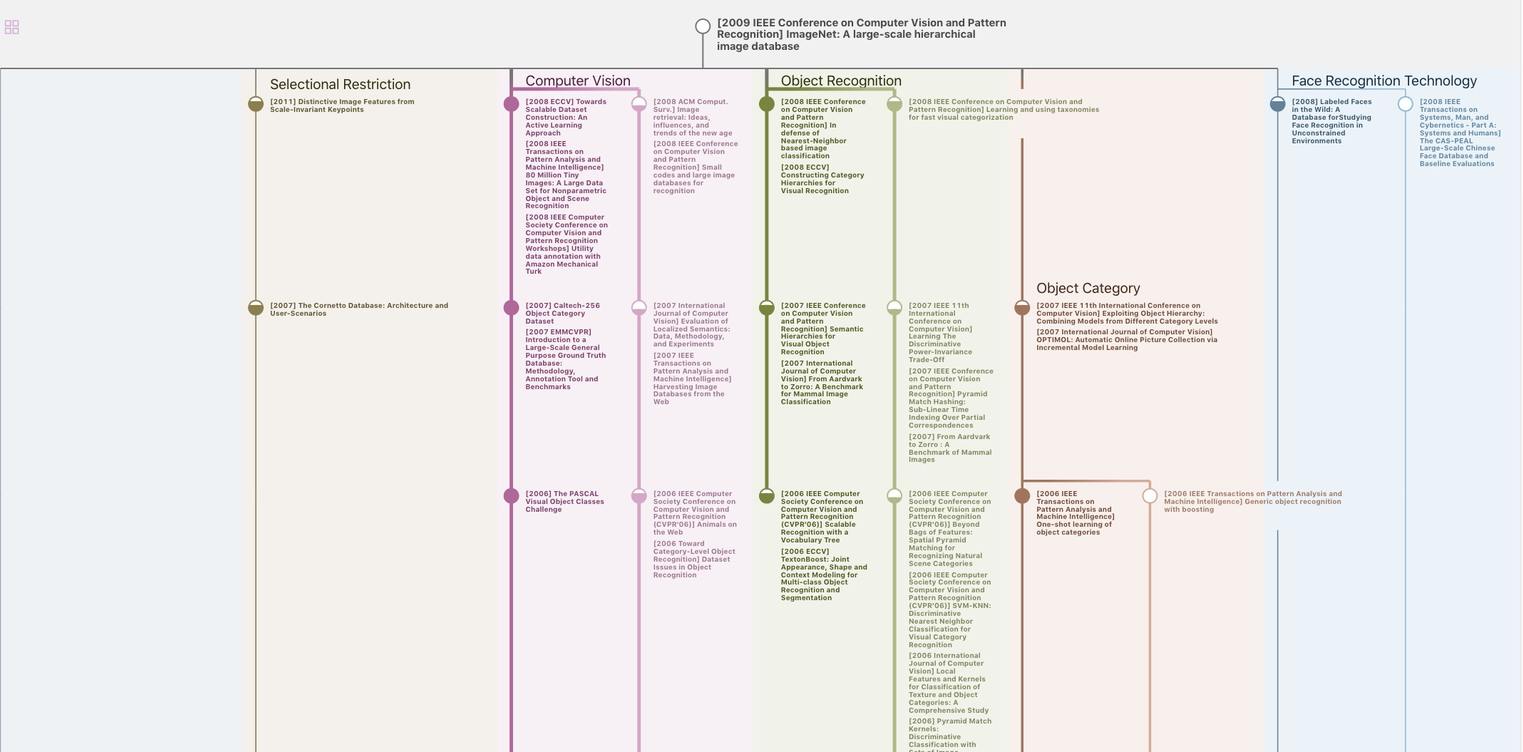
生成溯源树,研究论文发展脉络
Chat Paper
正在生成论文摘要