Situation-Aware Deep Reinforcement Learning for Autonomous Nonlinear Mobility Control in Cyber-Physical Loitering Munition Systems
arxiv(2022)
摘要
According to the rapid development of drone technologies, drones are widely used in many applications including military domains. In this paper, a novel situation-aware DRL- based autonomous nonlinear drone mobility control algorithm in cyber-physical loitering munition applications. On the battlefield, the design of DRL-based autonomous control algorithm is not straightforward because real-world data gathering is generally not available. Therefore, the approach in this paper is that cyber-physical virtual environment is constructed with Unity environment. Based on the virtual cyber-physical battlefield scenarios, a DRL-based automated nonlinear drone mobility control algorithm can be designed, evaluated, and visualized. Moreover, many obstacles exist which is harmful for linear trajectory control in real-world battlefield scenarios. Thus, our proposed autonomous nonlinear drone mobility control algorithm utilizes situation-aware components those are implemented with a Raycast function in Unity virtual scenarios. Based on the gathered situation-aware information, the drone can autonomously and nonlinearly adjust its trajectory during flight. Therefore, this approach is obviously beneficial for avoiding obstacles in obstacle-deployed battlefields. Our visualization-based performance evaluation shows that the proposed algorithm is superior from the other linear mobility control algorithms.
更多查看译文
关键词
mobility control,autonomous nonlinear
AI 理解论文
溯源树
样例
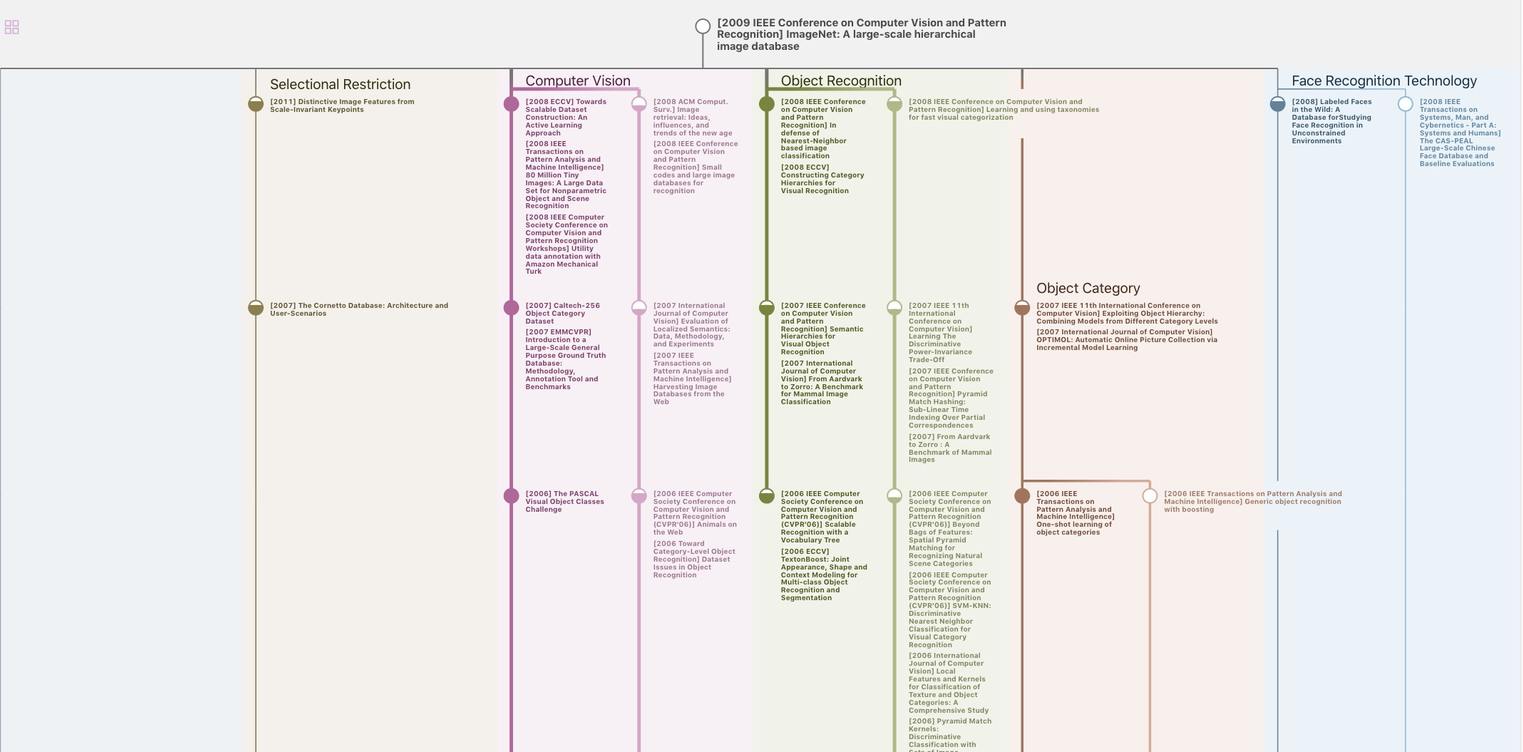
生成溯源树,研究论文发展脉络
Chat Paper
正在生成论文摘要