Accelerated multiscale mechanics modeling in a deep learning framework
arxiv(2022)
摘要
Microstructural heterogeneity affects the macro-scale behavior of materials. Conversely, load distribution at the macro-scale changes the microstructural response. These up-scaling and down-scaling relations are often modeled using multiscale finite element (FE) approaches such as FE-squared ($FE^2$). However, $FE^2$ requires numerous calculations at the micro-scale, which often renders this approach intractable. This paper reports an enormously faster machine learning (ML) based approach for multiscale mechanics modeling. The proposed ML-driven multiscale analysis approach uses an ML-model that predicts the local stress tensor fields in a linear elastic fiber-reinforced composite microstructure. This ML-model, specifically a U-Net deep convolutional neural network (CNN), is trained separately to perform the mapping between the spatial arrangement of fibers and the corresponding 2D stress tensor fields. This ML-model provides effective elastic material properties for up-scaling and local stress tensor fields for subsequent down-scaling in a multiscale analysis framework. Several numerical examples demonstrate a substantial reduction in computational cost using the proposed ML-driven approach when compared with the traditional multiscale modeling approaches such as full-scale FE analysis, and homogenization based $FE^2$ analysis. This approach has tremendous potential in efficient multiscale analysis of complex heterogeneous materials, with applications in uncertainty quantification, design, and optimization.
更多查看译文
关键词
multiscale mechanics,deep learning framework,deep learning,accelerated
AI 理解论文
溯源树
样例
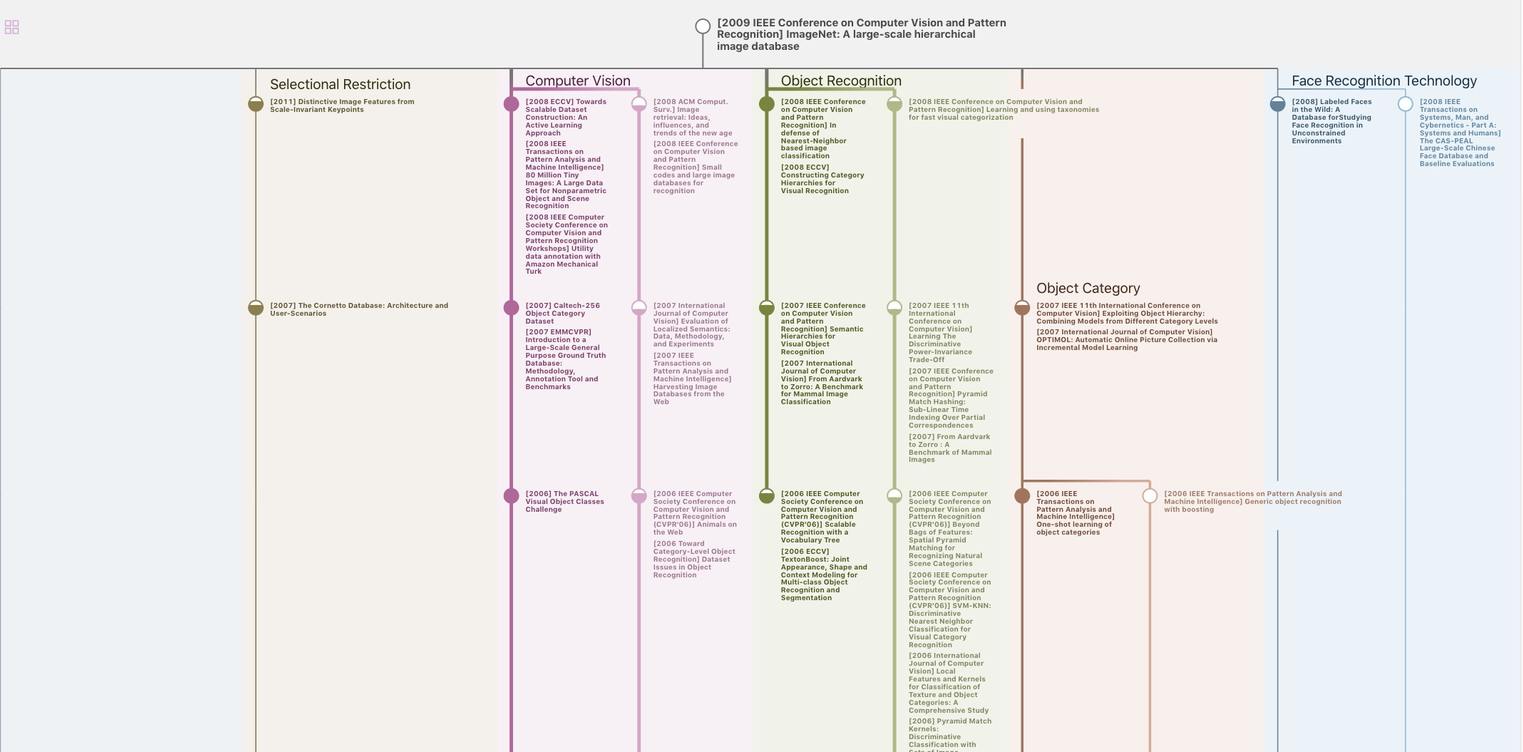
生成溯源树,研究论文发展脉络
Chat Paper
正在生成论文摘要