Audio Embedding-Aware Dialogue Policy Learning
IEEE/ACM Transactions on Audio, Speech, and Language Processing(2023)
摘要
Following the success of Natural Language Processing (NLP) transformers pretrained via self-supervised learning, similar models have been proposed recently for speech processing such as Wav2Vec2, HuBERT and UniSpeech-SAT. An interesting yet unexplored area of application of these models is Spoken Dialogue Systems, where the users' audio signals are typically just mapped to word-level features derived from an Automatic Speech Recogniser (ASR), and then processed using NLP techniques to generate system responses. This paper reports a comprehensive comparison of dialogue policies trained using ASR-based transcriptions and extended with the aforementioned audio processing transformers in the DSTC2 task. Whilst our dialogue policies are trained with supervised and policy-based deep reinforcement learning, they are assessed using both automatic task completion metrics and a human evaluation. Our results reveal that using audio embeddings is more beneficial than detrimental in most of our trained dialogue policies, and that the benefits are stronger for supervised learning than reinforcement learning.
更多查看译文
关键词
Audio embeddings,deep reinforcement learning,spoken dialogue systems,transformer neural networks
AI 理解论文
溯源树
样例
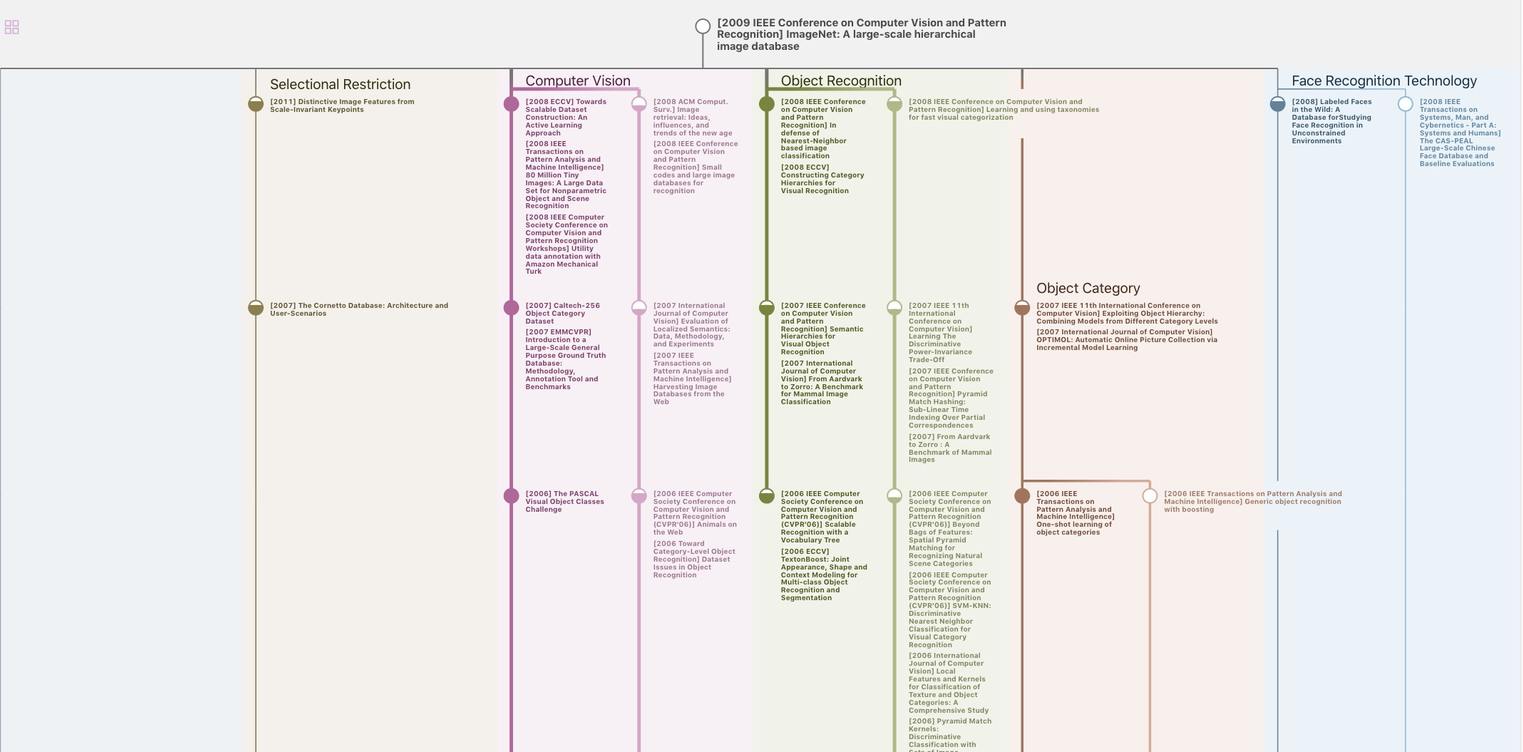
生成溯源树,研究论文发展脉络
Chat Paper
正在生成论文摘要