Towards Energy-Preserving Natural Language Understanding With Spiking Neural Networks
IEEE/ACM Transactions on Audio, Speech, and Language Processing(2023)
摘要
Artificial neural networks have shown promising results in a variety of natural language understanding (NLU) tasks. Despite their successes, conventional neural-based NLU models are criticized for high energy consumption, making them laborious to be widely applied in low-power electronics, such as smartphones and intelligent terminals. In this paper, we introduce a potential direction to alleviate this bottleneck by proposing a spiking encoder. The core of our model is bi-directional spiking neural network (SNN) which transforms numeric values into discrete spiking signals and replaces massive multiplications with much cheaper additive operations. We examine our model on sentiment classification and machine translation tasks. Experimental results reveal that our model achieves comparable classification and translation accuracy to advanced
Transformer
baseline, whereas significantly reduces the required computational energy to 0.82%.
更多查看译文
关键词
Natural language processing,language model,natural language understanding,spiking neural network
AI 理解论文
溯源树
样例
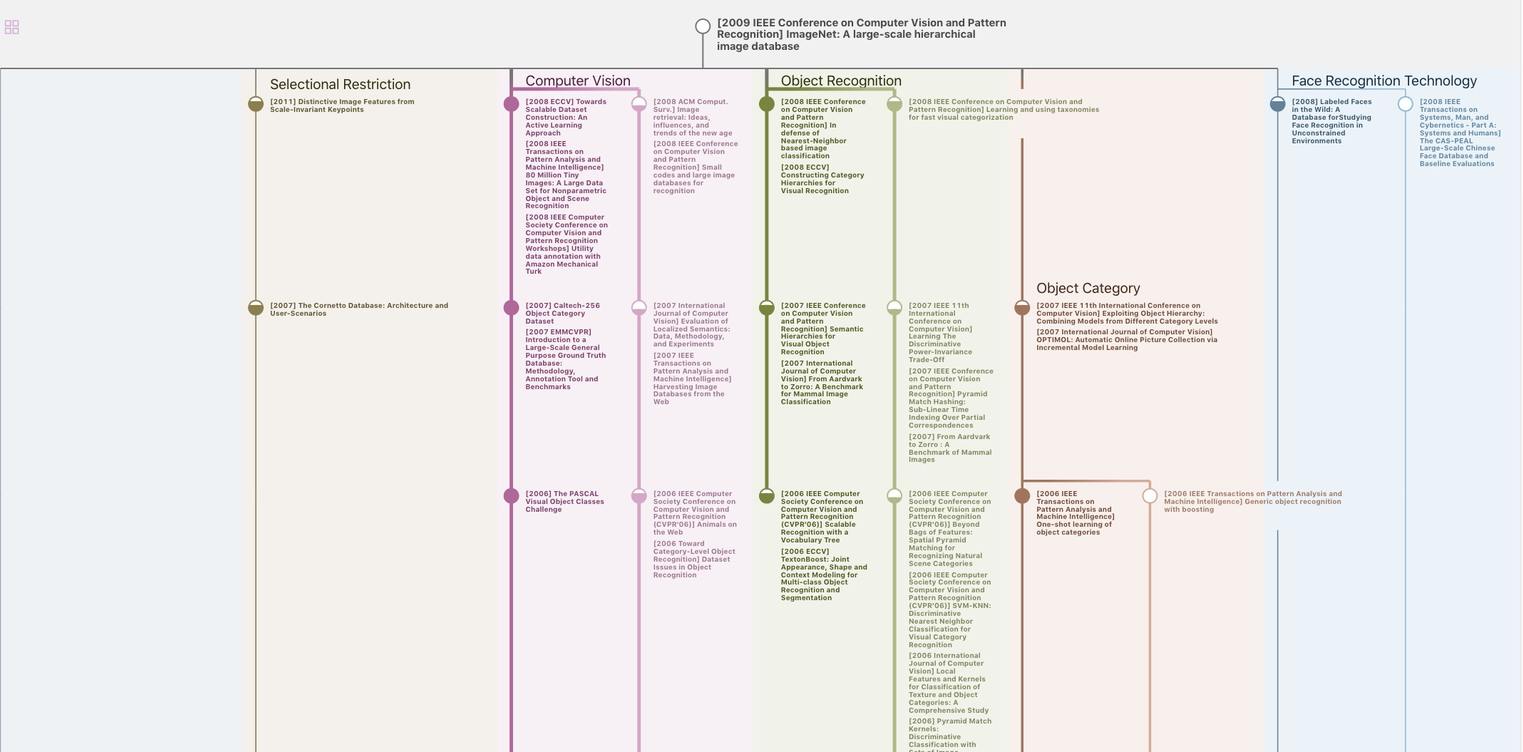
生成溯源树,研究论文发展脉络
Chat Paper
正在生成论文摘要