Quaternion Scalar and Vector Norm Decomposition: Quaternion PCA for Color Face Recognition
IEEE Transactions on Image Processing(2023)
摘要
This paper proposes a decomposition called quaternion scalar and vector norm decomposition (QSVND) for approximation problems in color image processing. Different from traditional quaternion norm approximations that are always the single objective models (SOM), QSVND is adopted to transform the SOM into the bi-objective model (BOM). Furthermore, regularization is used to solve the BOM problem as a common scalarization method, which converts the BOM into a more reasonable SOM. This can handle over-fitting or under-fitting problems neglected in this kind of research for quaternion representation (QR) in color image processing. That is how to treat redundancy caused by the extra scalar part when the vector part of a quaternion is used to represent a color pixel. We apply QSVND to quaternion principal component analysis (QPCA) for color face recognition (FR), which can deal with the phenomenon of under-fitting of vector part norm approximation. Comparisons with the competing approaches on AR, FERET, FEI, and KDEF & AKDEF databases consistently show the superiority of the proposed approach for color FR.
更多查看译文
关键词
Quaternion scalar and vector norm decomposition,regularization,principal component analysis,color face recognition
AI 理解论文
溯源树
样例
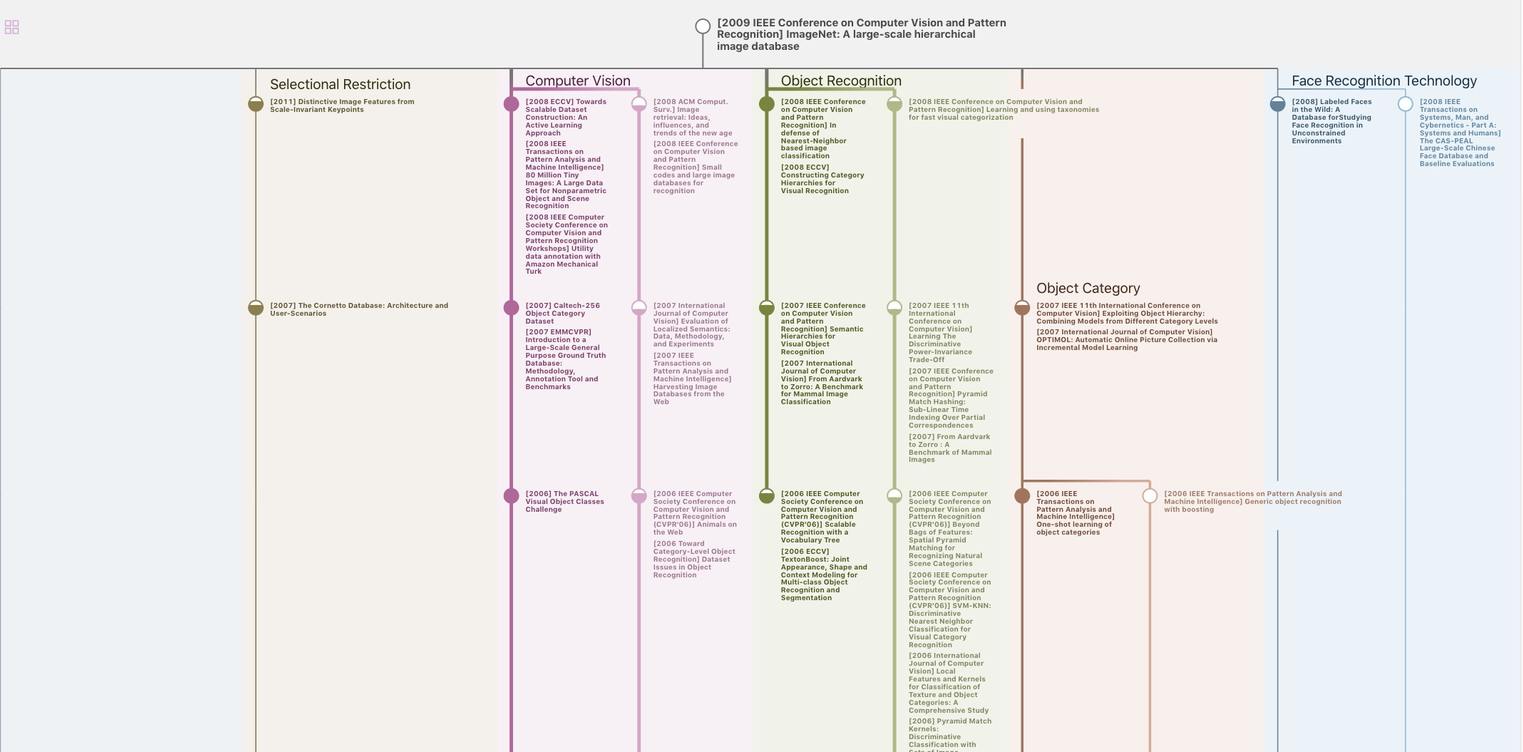
生成溯源树,研究论文发展脉络
Chat Paper
正在生成论文摘要