Avoiding Overconfidence in Predictions of Residential Energy Demand Through Identification of the Persistence Forecast Effect
IEEE Transactions on Smart Grid(2023)
摘要
Forecasting domestic electricity consumption is important for a wide range of modern power system solutions and smart applications that support network operation, grid stability, and demand-side management, most of which depend on robust and accurate predictions. The methods producing these predictions infer future load from statistical regularity in historical data. If such regularity is lacking, predictions then regress towards the most recently observed consumption value used in the input set. Predictions then follow the actual load data one step behind in time, potentially affecting the robustness of predictions and functionality of applications. Current evaluation methods do not detect this behaviour which may result in overconfidence in prediction results. In this study, we I) define and systematically analyse this behaviour, which we label the Persistence Forecast Effect and illustrate its impacts, II) propose a novel method, called 1-Step-Shifting, to detect its presence, and III) analyse and establish the relationship between irregularity in data and the effect. Further, we provide a case study applying state-of-the-art forecasting techniques to a real-world dataset of electricity consumption data from 69 households in order to demonstrate the Persistence Forecast Effect, its implications, and its relationship to statistical regularity in historical data.
更多查看译文
关键词
Demand forecasting,demand-side management,energy consumption,energy efficiency,time-series analysis
AI 理解论文
溯源树
样例
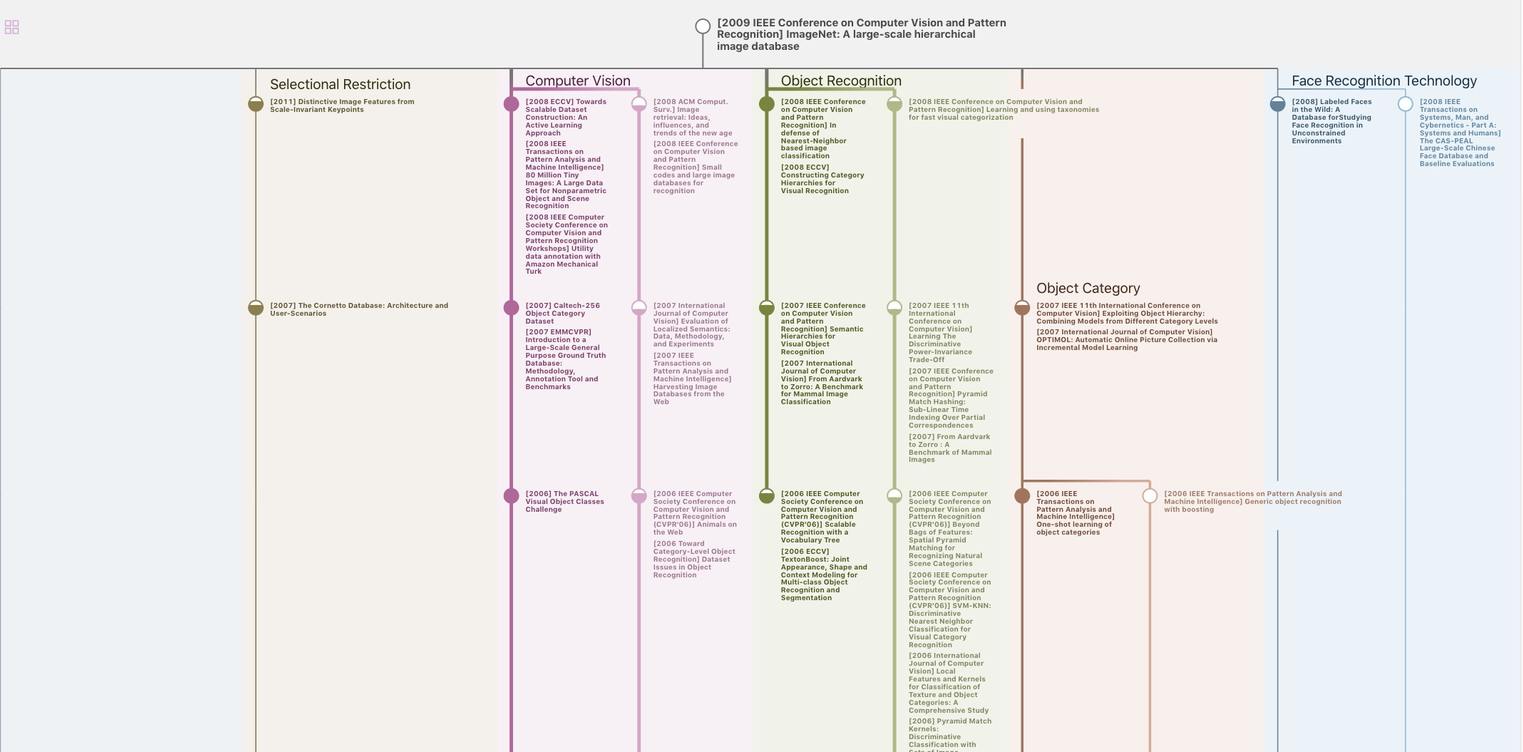
生成溯源树,研究论文发展脉络
Chat Paper
正在生成论文摘要