Marker Free Gait Analysis using Pose Estimation Model
2022 IEEE 20th Student Conference on Research and Development (SCOReD)(2022)
摘要
In recent years, gait analysis has gained prominence among scholars. Gait analysis is used extensively in medical diagnoses, rehabilitation, and biometric identification. Clinical gait analysis is often conducted in a gait lab employing a 3D motion capture system and a pressure sensing walkway. Using wearable inertial sensors, the gait assessment can also be conducted outside the gait lab. However, these methods are limited by expensive equipment and the need for specialized knowledge to conduct a reliable gait evaluation. Thus, a marker-free deep learning based pose estimation method is suggested to assess the lower limb joint kinematics robustly and accurately during gait analysis. This study seeks to determine the pose estimation model that provide reliable and accurate lower limb joint kinematics evaluation in real-world applications. In the conclusion, the average inference speeds for OpenPose, MediaPipe Pose, and MMPose are 17.00, 30.19, and 2.82 frames per second, respectively, with average correlations of 0.896, 0.944, and 0.942 between the calculated lower limb joint kinematics and baseline. Therefore, MediaPipe Pose is the best pose estimation model for assessing the kinematics of the lower limb joints in real-world applications.
更多查看译文
关键词
Markerless gait analysis,gait kinematics,OpenPose,MediaPipe Pose,MMPose
AI 理解论文
溯源树
样例
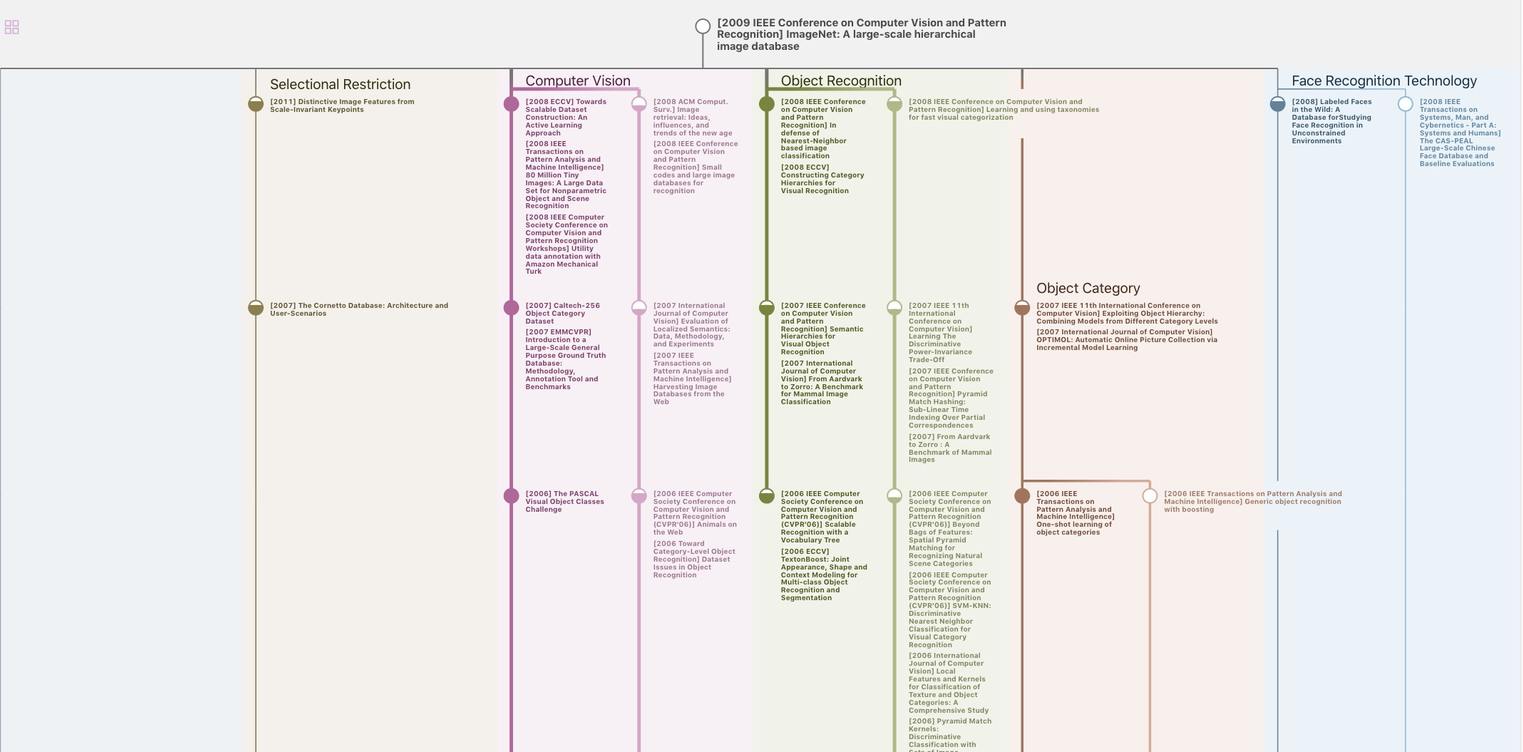
生成溯源树,研究论文发展脉络
Chat Paper
正在生成论文摘要