Integrating very-high-resolution UAS data and airborne imaging spectroscopy to map the fractional composition of Arctic plant functional types in Western Alaska
Remote Sensing of Environment(2023)
摘要
Widespread changes in vegetation cover and composition are driving strong impacts on Arctic ecosystem functioning and global climate feedbacks. An accurate characterization of tundra vegetation composition is required to understand how the Arctic will respond to future climate change. However, quantifying tundra vegetation composition over large areas is challenging as commonly-used satellite observations are too coarse, spatially and spectrally, to differentiate low-lying tundra vegetation types. Recent airborne and spaceborne imaging spectroscopy platforms provide better data to characterize vegetation composition. Yet, our ability to characterize vegetation composition with imaging spectroscopy remains largely unexplored in the Arctic, particularly due to a lack of ground observations needed to train and test classification models. To address this problem, we collected very-high-resolution (VHR, ∼5 cm) unoccupied aerial system (UAS) imagery at three low-Arctic tundra sites located on the Seward Peninsula, western Alaska. In this paper, we examine the feasibility of integrating imagery from the UAS and the hyperspectral Airborne Visible/Infrared Imaging Spectrometer, Next Generation (AVIRIS-NG) airborne instrument to map the fractional composition of 12 key Arctic plant functional types (PFTs). To this end, we first mapped the 12 PFTs from our VHR UAS imagery using random forest classification. We then used these UAS-derived PFT maps as ground truth to develop partial least squares regression (PLSR) models to predict the fractional cover (FCover) of each PFT from AVIRIS-NG imagery. We evaluated the performance of our PLSR models using reserved UAS samples, as well as by mapping PFT FCover and dominant PFT for large tundra landscapes. Our results show that 1) Arctic PFTs can be effectively mapped using VHR UAS imagery, with overall accuracy between 86% and 92%, 2) when the UAS mapped PFTs were used to inform PLSR scaling models, the FCover of the 12 PFTs could be effectively estimated from AVIRIS-NG imagery with a mean absolute error (MAE) <0.13, and 3) our PLSR models outperformed traditional, fully constrained least-squares (FCLS) linear mixture analysis and produced high-quality, spatially contiguous PFT FCover and PFT maps that captured vegetation spatial patterns with similar accuracy to those developed from UAS imagery. The developed PLSR models have the potential to be broadly applied for quantifying vegetation composition with AVIRIS-NG images to help monitor tundra vegetation dynamics and improve process-based modeling of tundra ecosystems.
更多查看译文
关键词
Arctic,PFT,PLSR,UAS,Unmixing,Vegetation cover,AVIRIS-NG
AI 理解论文
溯源树
样例
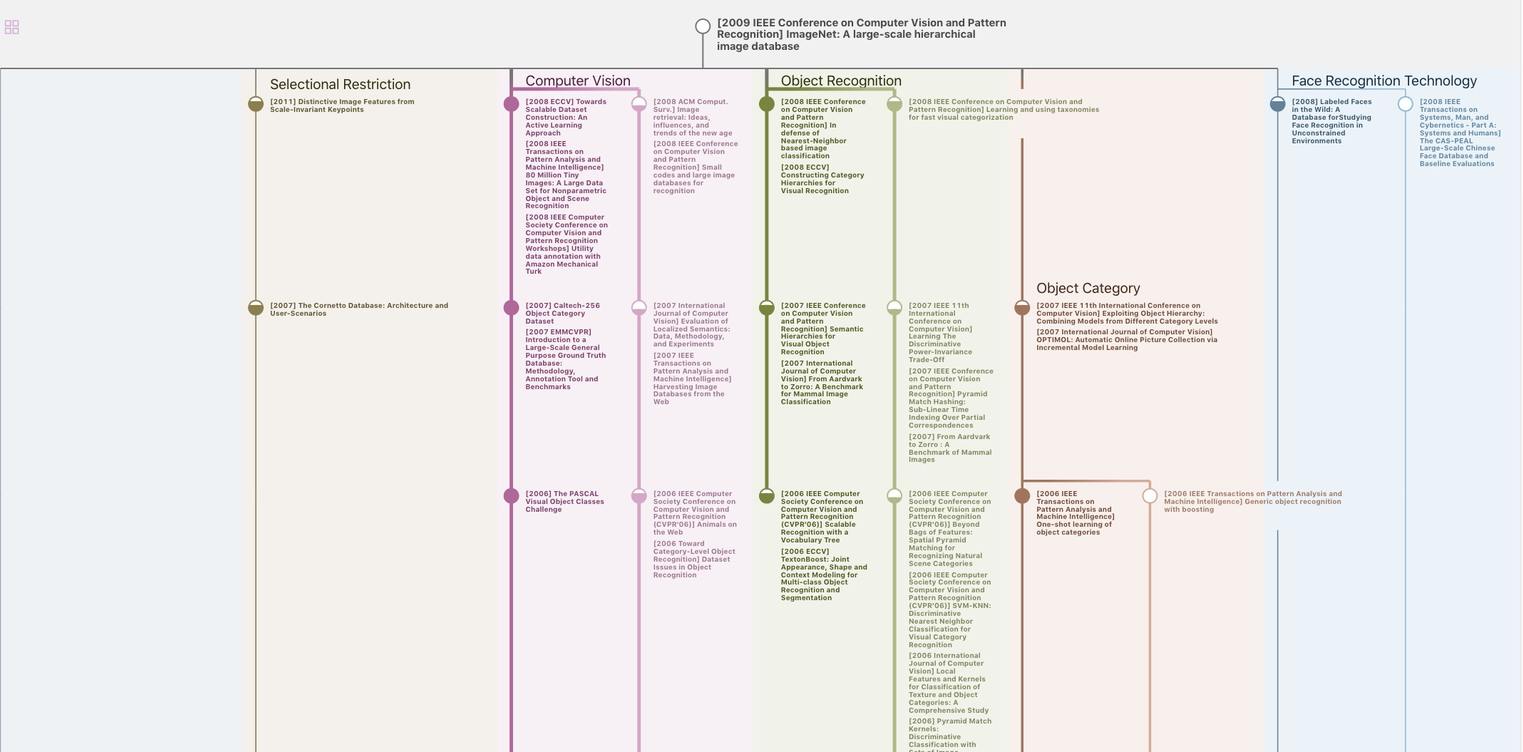
生成溯源树,研究论文发展脉络
Chat Paper
正在生成论文摘要