Knowledge discovery with computational fluid dynamics: Supercritical airfoil database and drag divergence prediction
Physics of Fluids(2023)
摘要
Aerodynamic rules and knowledge are often obtained through theoretical research and experiments, which have contributed greatly to aircraft design. For example, Korn's equation predicts the airfoil drag divergence Mach number using the airfoil maximum thickness and the lift coefficient. It is very helpful in the aircraft initial design. However, it neither reveals the key factors of fluid features on the drag divergence nor contributes to the detailed design. This paper designs a supercritical airfoil database that covers the typical free stream Mach number, angle of attack, lift coefficient, and geometry of modern transonic commercial aircraft. Correlation screening and multivariate regression are carried out to discover knowledge about the airfoil drag divergence Mach number and pressure distribution features. A new linear correlation is discovered and validated by existed airfoil databases. Compared with Korn's equation, the discovered correlation reduces the maximum prediction error by approximately 40%. It indicates that the drag divergence Mach number can be increased by obtaining a shock wave that is further upstream in the detailed design. Furthermore, it enables the cruise performance and drag divergence Mach number to be predicted with only one simulation of the cruise point, which will greatly save the computational cost of optimizations.
更多查看译文
关键词
supercritical airfoil database,computational fluid dynamics,knowledge discovery,divergence prediction
AI 理解论文
溯源树
样例
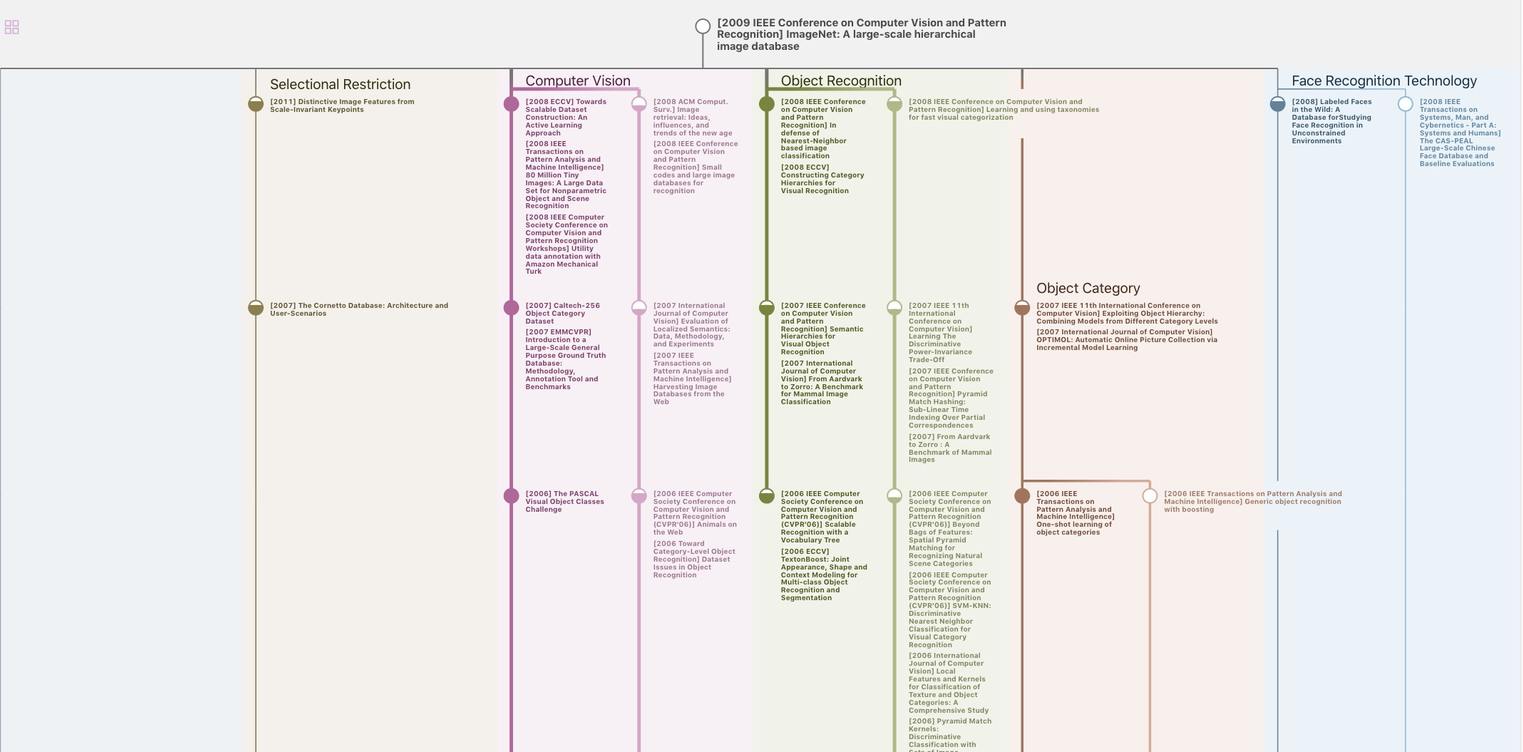
生成溯源树,研究论文发展脉络
Chat Paper
正在生成论文摘要