A DRL-Based Decentralized Computation Offloading Method: An Example of an Intelligent Manufacturing Scenario
IEEE Transactions on Industrial Informatics(2022)
摘要
With the development of edge computing and 5G, the demand for resource-limited devices to execute computation-intensive tasks can be effectively alleviated. The research on computation offloading lays an essential foundation for realizing mobile edge computing, and deep reinforcement learning (DRL) has become an emerging technique to address the computation offloading problem. This article utilizes a DRL-based algorithm to design a decentralized computation offloading framework aimed at minimizing the computational cost. We employ a multiuser system model with a single-edge server suitable for industrial scenarios. Then, we propose a dual-critic deep deterministic policy gradient (DC-DDPG) algorithm based on the deep deterministic policy gradient (DDPG) algorithm to tackle computation offloading and resource allocation problems for all users. DC-DDPG adopts two critic nets in both the primary and target nets to fit the action value of two different optimization objectives, which expedites the convergence during the training process and reduces the computational cost of the edge computation system during operation. Compared with other DRL methods, such as deep Q-network and DDPG, numerical results demonstrate that the proposed DC-DDPG algorithm has a faster convergence speed and performs significantly better than other DRL-based algorithms in terms of system computational cost in computing-intensive tasks, which makes it more suitable for industrial intelligent manufacturing scenarios with large data volume.
更多查看译文
关键词
decentralized computation offloading method,manufacturing,drl-based
AI 理解论文
溯源树
样例
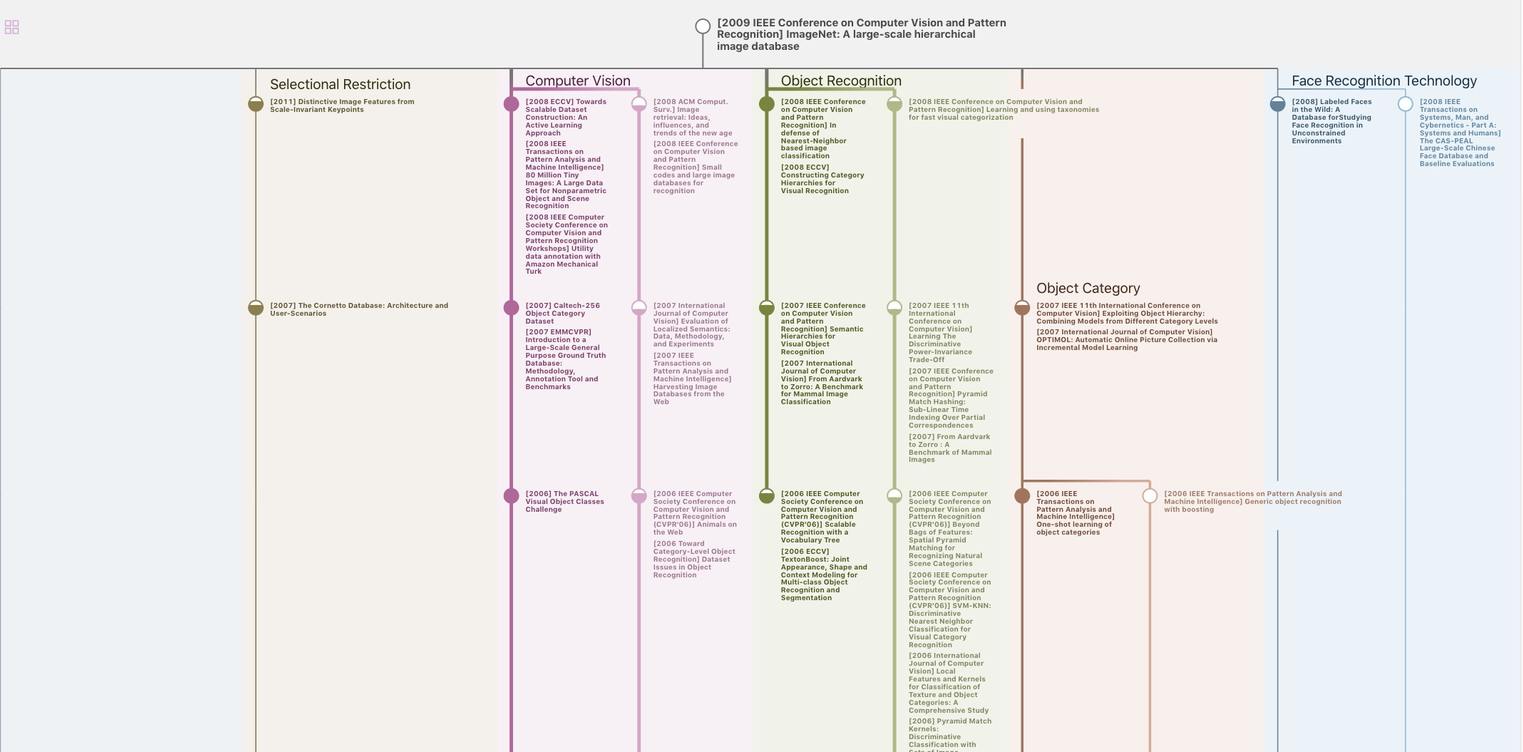
生成溯源树,研究论文发展脉络
Chat Paper
正在生成论文摘要