Understanding Spatio-Temporal Relations in Human-Object Interaction using Pyramid Graph Convolutional Network
2022 IEEE/RSJ International Conference on Intelligent Robots and Systems (IROS)(2022)
摘要
Human activities recognition is an important task for an intelligent robot, especially in the field of human-robot collaboration, it requires not only the label of subactivities but also the temporal structure of the activity. In order to automatically recognize both the label and the temporal structure in sequence of human-object interaction, we propose a novel Pyramid Graph Convolutional Network (PGCN), which employs a pyramidal encoder-decoder architecture consisting of an attention based graph convolution network and a temporal pyramid pooling module for downsampling and upsampling interaction sequence on the temporal axis, respectively. The system represents the 2D or 3D spatial relation of human and objects from the detection results in video data as a graph. To learn the human-object relations, a new attention graph convolutional network is trained to extract condensed information from the graph representation. To segment action into sub-actions, a novel temporal pyramid pooling module is proposed, which upsamples compressed features back to the original time scale and classifies actions per frame. We explore various attention layers, namely spatial attention, temporal attention and channel attention, and combine different upsampling decoders to test the performance on action recognition and segmentation. We evaluate our model on two challenging datasets in the field of human-object interaction recognition, i.e. Bimanual Actions and IKEA Assembly datasets. We demonstrate that our classifier significantly improves both framewise action recognition and segmentation, e.g., F1 micro and F1@50 scores on Bimanual Actions dataset are improved by 4.3% and 8.5% respectively.
更多查看译文
关键词
3D spatial relation,action recognition,attention based graph convolution network,attention graph convolutional network,attention layers,bimanual actions dataset,channel attention,classifies actions,graph representation,human activities recognition,human-object interaction recognition,human-object relations,human-robot collaboration,intelligent robot,novel temporal pyramid pooling module,original time scale,PGCN,pyramid graph convolutional network,pyramidal encoder-decoder architecture,segment action,segmentation,spatial attention,spatio-temporal relations,temporal attention,temporal axis,temporal structure,upsampling decoders,upsampling interaction sequence
AI 理解论文
溯源树
样例
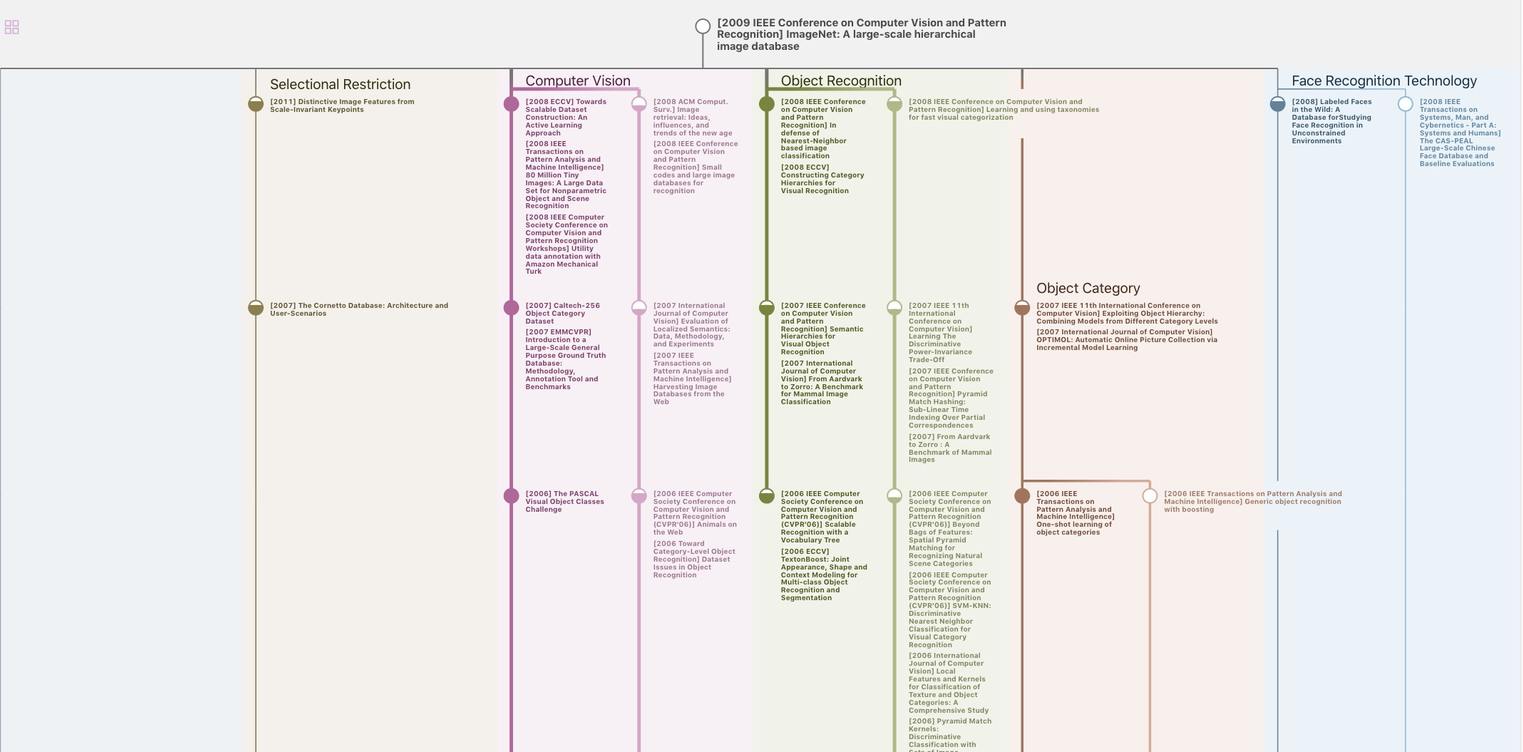
生成溯源树,研究论文发展脉络
Chat Paper
正在生成论文摘要