LODM: Large-scale Online Dense Mapping for UAV
2022 IEEE/RSJ International Conference on Intelligent Robots and Systems (IROS)(2022)
摘要
This paper proposes an online large-scale dense mapping method for UAVs with a height of 150-250 meters. We first fuse the GPS with the visual odometry to estimate the scaled poses and sparse points. In order to use the depth of sparse points for depth map, we propose Sparse Confidence Cascade View-Aggregation MVSNet (SCCVA-MVSNet), which projects the depth-converged points in the sliding window on keyframes to obtain a sparse depth map. To weigh the confidence of the depth of each sparse point, we construct sparse confidence by the photometric error. The images of all keyframes, coarse depth, and confidence as the input of CVA-MVSNet to extract features and construct 3D cost volumes with adaptive view aggregation to balance the different stereo baselines between the keyframes. Our proposed network utilizes sparse features point information, the output of the network better maintains the consistency of the scale. Our experiments show that MVSNet using sparse feature point information outperforms image-only MVSNet, and our online reconstruction results are comparable to offline reconstruction methods. To benefit the research community, we open our code at https://github.com/hjxwhy/LODM.git
更多查看译文
关键词
3D cost volumes,adaptive view aggregation,coarse depth,depth-converged points,feature extraction,GPS,keyframes,large-scale online dense mapping,online large-scale dense mapping method,online reconstruction results,photometric error,scaled pose estimation,SCCVA-MVSNet,sparse confidence cascade view-aggregation MVSNet,sparse depth map,sparse feature point information,sparse point,UAV,visual odometry
AI 理解论文
溯源树
样例
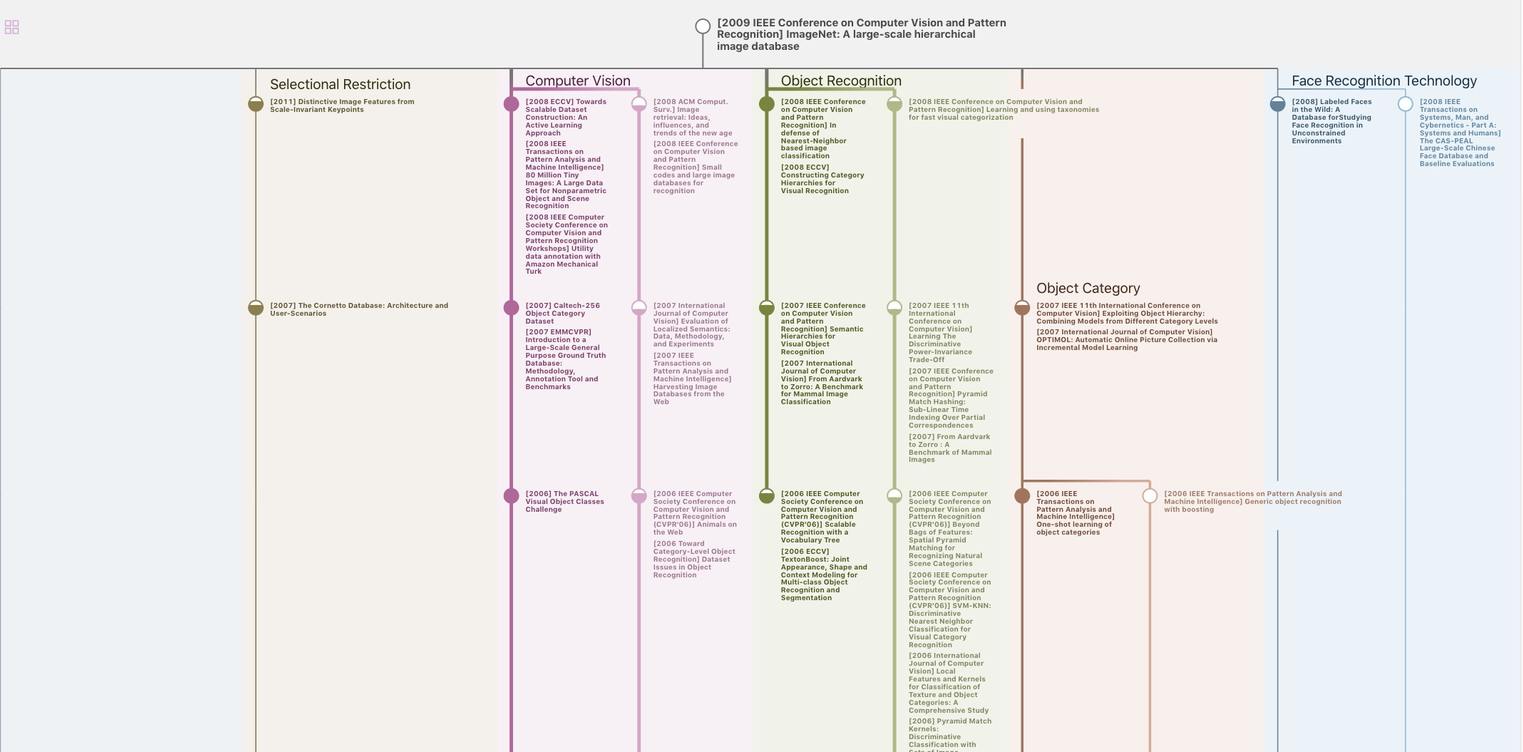
生成溯源树,研究论文发展脉络
Chat Paper
正在生成论文摘要