Attention-guided RGB-D Fusion Network for Category-level 6D Object Pose Estimation
2022 IEEE/RSJ International Conference on Intelligent Robots and Systems (IROS)(2022)
摘要
This work focuses on estimating 6D poses and sizes of category-level objects from a single RGB-D image. How to exploit the complementary RGB and depth features plays an important role in this task yet remains an open question. Due to the large intra-category texture and shape variations, an object instance in test may have different RGB and depth features from those of the object instances in training, which poses challenges to previous RGB-D fusion methods. To deal with such problem, an Attention-guided RGB-D Fusion Network (ARF-Net) is proposed in this work. Our key design is an ARF module that learns to adaptively fuse RGB and depth features with guidance from both structure-aware attention and relation-aware attention. Specifically, the structure-aware attention captures spatial relationship among object parts and the relation-aware attention captures the RGB-to-depth correlations between the appearance and geometric features. Our ARF -Net directly establishes canonical correspondences with a compact decoder based on the multi-modal features from our ARF module. Extensive experiments show that our method can effectively fuse RGB features to various popular point cloud encoders and provide consistent performance improvement. In particular, without reconstructing instance 3D models, our method with its relatively compact architecture outperforms all state-of-the-art models on CAMERA25 and REAL275 benchmarks by a large margin.
更多查看译文
关键词
ARF -Net,ARF module,ARF-Net,Attention-guided RGB-D Fusion Network,category-level 6D object pose estimation,category-level objects,depth features,geometric features,intra-category texture,multimodal features,object instance,previous RGB-D fusion methods,relation-aware attention,RGB features,RGB-to-depth correlations,shape variations,single RGB-D image,structure-aware attention captures spatial relationship
AI 理解论文
溯源树
样例
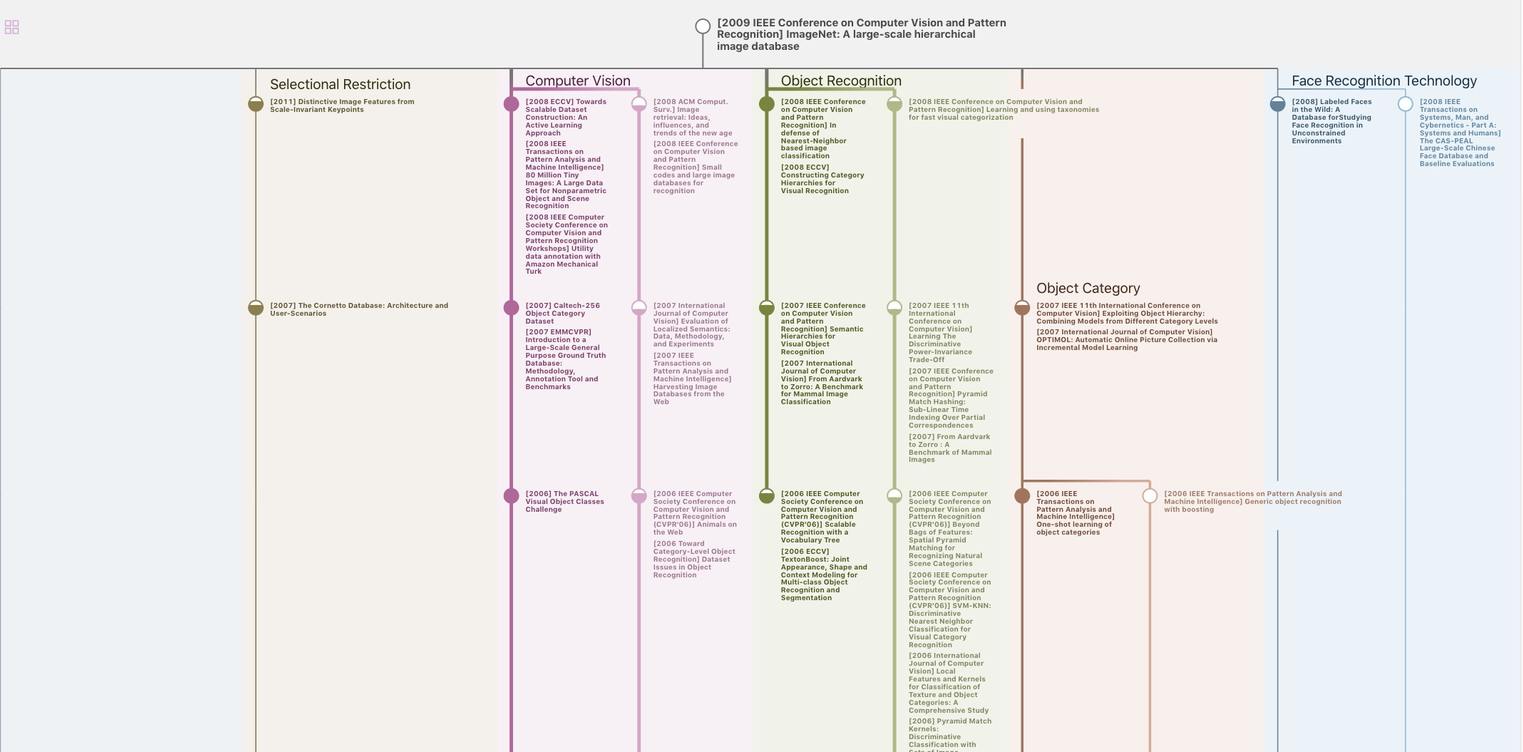
生成溯源树,研究论文发展脉络
Chat Paper
正在生成论文摘要