Excavation of Fragmented Rocks with Multi-modal Model-based Reinforcement Learning
2022 IEEE/RSJ International Conference on Intelligent Robots and Systems (IROS)(2022)
摘要
This paper presents a multi-modal model-based reinforcement learning (MBRL) approach to the excavation of fragmented rocks, which are very challenging to model due to their highly variable sizes and geometries, and visual occlusions. A multi-modal recurrent neural network (RNN) learns the dynamics of bucket-terrain interaction from a small physical dataset, with a discrete set of motion primitives encoded with domain knowledge as the action space. Then a model predictive controller (MPC) tracks a global reference path using multimodal feedback. We show that our RNN-based dynamics function achieves lower prediction errors compared to a feed-forward neural network baseline, and the MPC is able to significantly outperform manually designed strategies on such a challenging task.
更多查看译文
关键词
bucket-terrain interaction,excavation,feed-forward neural network baseline,fragmented rocks,geometries,highly variable sizes,model predictive controller,multimodal feedback,multimodal model-based reinforcement learning approach,multimodal recurrent neural network,RNN-based dynamics
AI 理解论文
溯源树
样例
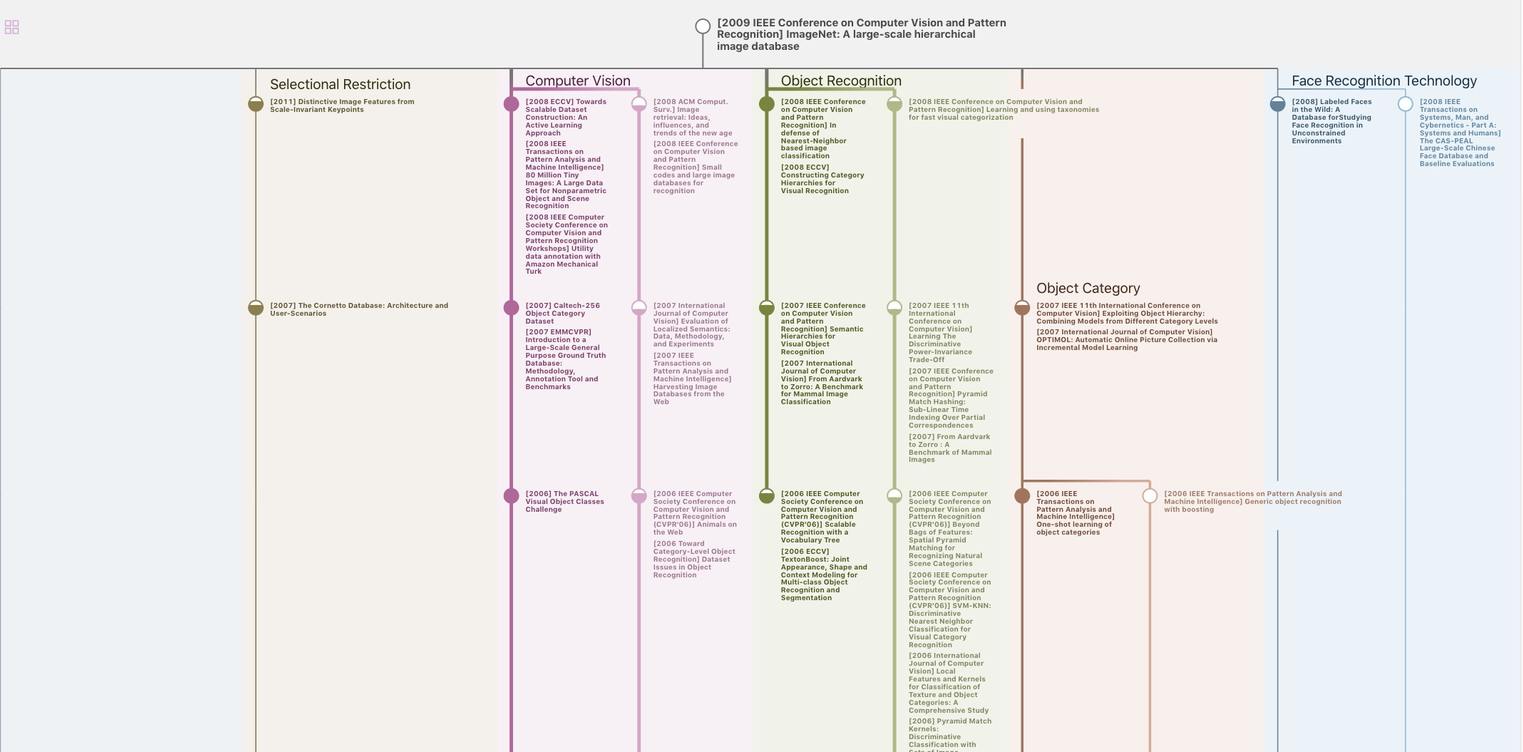
生成溯源树,研究论文发展脉络
Chat Paper
正在生成论文摘要