Locality Aware Temporal FMs for Crime Prediction
Conference on Information and Knowledge Management(2022)
摘要
ABSTRACTCrime forecasting techniques can play a leading role in hindering crime occurrences, especially in areas under possible threat. In this paper, we propose Locality Aware Temporal Factorization Machines (LTFMs) for crime prediction. Its locality representation module deploys a spatial encoder to estimate the regional dependencies using Graph Convolutional Networks (GCNs). Then, the Point of Interest (POI) encoder computes the weighted attentive aggregation of location, crime, and POI latent representations. The dynamic crime representation module utilizes the transformer-based positional encodings to capture the dependencies among space, time, and crime categories. The encodings learnt from locality representation and crime category encoders, are projected into a factorization machine-based architecture via a shared feed-forward network. An extensive comparison with state-of-art techniques, using Chicago and New York's criminal records, shows the significance of LTFMs.
更多查看译文
关键词
crime prediction
AI 理解论文
溯源树
样例
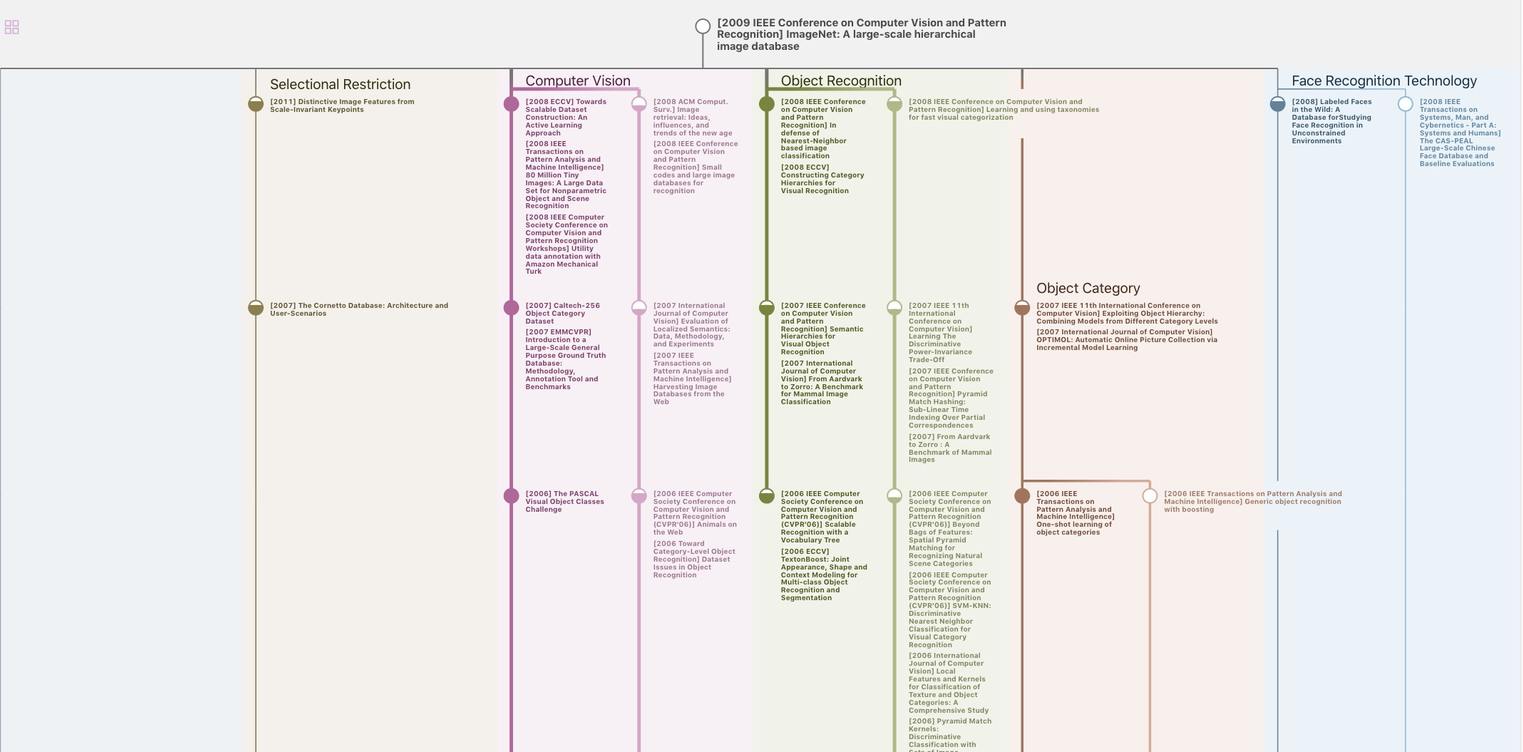
生成溯源树,研究论文发展脉络
Chat Paper
正在生成论文摘要