Cognize Yourself: Graph Pre-Training via Core Graph Cognizing and Differentiating
Conference on Information and Knowledge Management(2022)
摘要
ABSTRACTWhile Graph Neural Networks (GNNs) have become de facto criterion in graph representation learning, they still suffer from label scarcity and poor generalization. To alleviate these issues, graph pre-training has been proposed to learn universal patterns from unlabeled data via applying self-supervised tasks. Most existing graph pre-training methods only use a single self-supervised task, which will lead to insufficient knowledge mining. Recently, there are also some works that try to use multiple self-supervised tasks, however, we argue that these methods still suffer from a serious problem, which we call it graph structure impairment. That is, there actually exists structural gaps among several tasks due to the divergence of optimization objectives, which means customized graph structures should be provided for different self-supervised tasks. Graph structure impairment not only significantly hurts the generalizability of pre-trained GNNs, but also leads to suboptimal solution, and there is no study so far to address it well. Motivated by Meta-Cognitive theory, we propose a novel model named Core Graph Cognizing and Differentiating (CORE) to deal with the problem in an effective approach. Specifically, CORE consists of cognizing network and differentiating process, the former cognizes a core graph which stands for the essential structure of the graph, and the latter allows it to differentiate into several task-specific graphs for different tasks. Besides, this is also the first study to combine graph pre-training with cognitive theory to build a cognition-aware model. Several experiments have been conducted to demonstrate the effectiveness of CORE.
更多查看译文
关键词
GNN Pre-training, Graph Transfer Learning, Graph Neural Networks, Graph Representation Learning
AI 理解论文
溯源树
样例
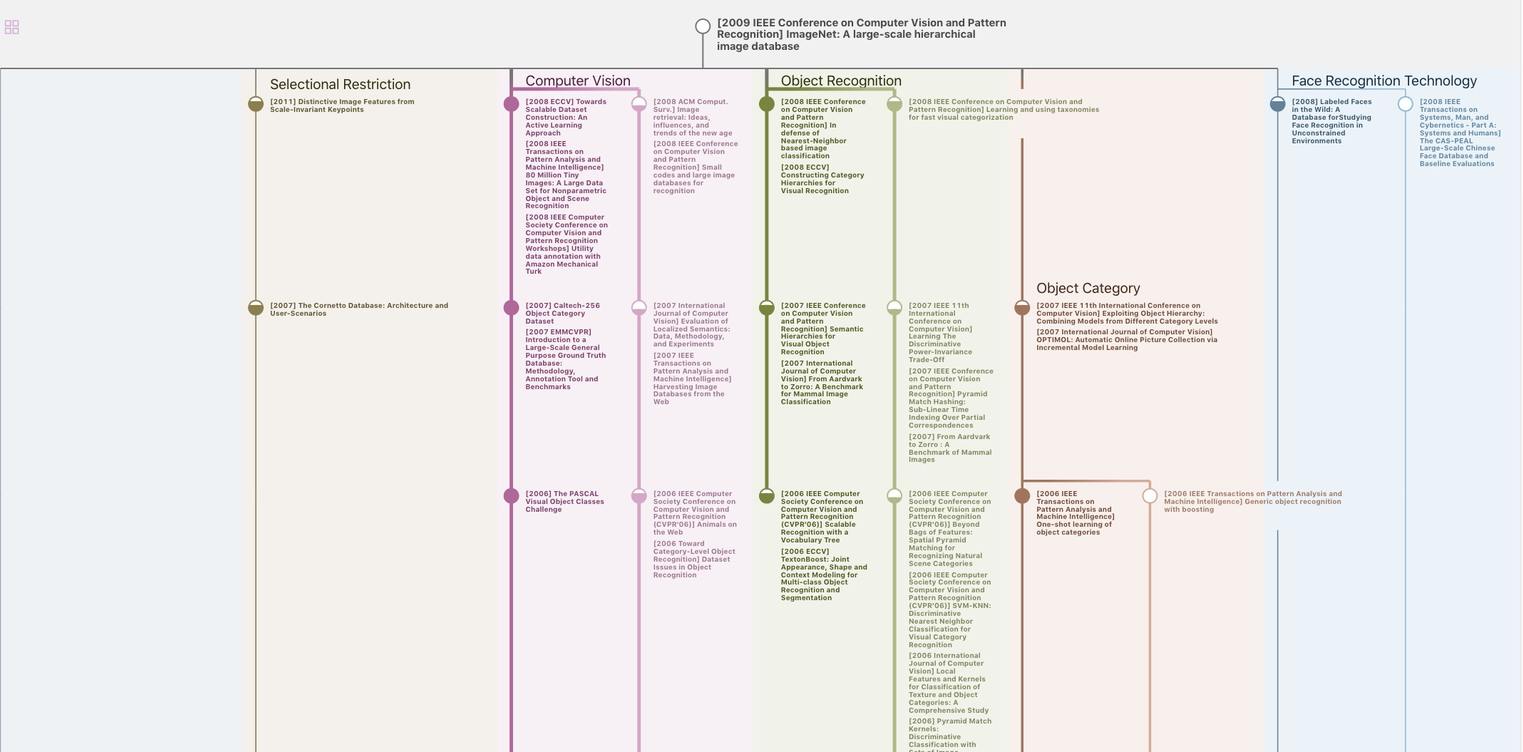
生成溯源树,研究论文发展脉络
Chat Paper
正在生成论文摘要