A Topic Modeling Approach to Classifying Open Street Map Health Clinics and Schools in Sub-Saharan Africa
arxiv(2022)
摘要
Data deprivation, or the lack of easily available and actionable information on the well-being of individuals, is a significant challenge for the developing world and an impediment to the design and operationalization of policies intended to alleviate poverty. In this paper we explore the suitability of data derived from OpenStreetMap to proxy for the location of two crucial public services: schools and health clinics. Thanks to the efforts of thousands of digital humanitarians, online mapping repositories such as OpenStreetMap contain millions of records on buildings and other structures, delineating both their location and often their use. Unfortunately much of this data is locked in complex, unstructured text rendering it seemingly unsuitable for classifying schools or clinics. We apply a scalable, unsupervised learning method to unlabeled OpenStreetMap building data to extract the location of schools and health clinics in ten countries in Africa. We find the topic modeling approach greatly improves performance versus reliance on structured keys alone. We validate our results by comparing schools and clinics identified by our OSM method versus those identified by the WHO, and describe OSM coverage gaps more broadly.
更多查看译文
关键词
topic modeling approach,map,africa,street,sub-saharan
AI 理解论文
溯源树
样例
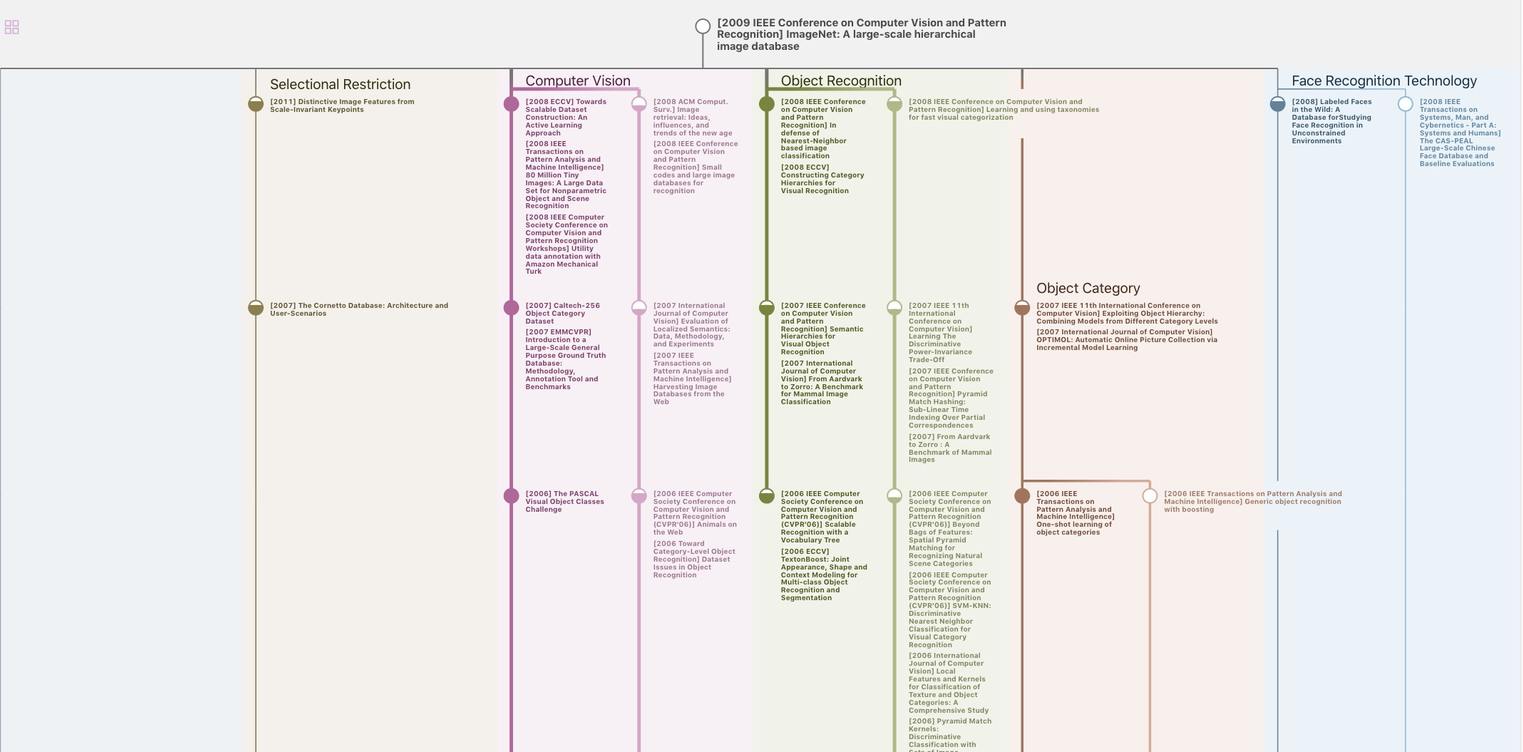
生成溯源树,研究论文发展脉络
Chat Paper
正在生成论文摘要