LSTM Molecular Descriptor-Free QSAR Application Research Based on Genetic Algorithm Optimization
2022 3rd International Conference on Big Data, Artificial Intelligence and Internet of Things Engineering (ICBAIE)(2022)
摘要
Traditional machine learning methods, especially random forests and support vector machines, can achieve good prediction accuracy in activity prediction, but the workload of screening molecular descriptors is too large and complicated. Therefore, this paper constructs and uses the QSAR model directly from the SMILES linear text representation of the compound, and uses the genetic algorithm-optimized long-short-term memory neural network model (GA-LSTM) to conduct quantitative structure-activity relationship analysis to predict human protein activity. The experimental results show that the accuracy and efficiency of the QSAR model without molecular descriptors based on GA-LSTM is higher than those of traditional machine learning methods, which provides a new idea and method for the pre-validation of drug development.
更多查看译文
关键词
lstm,descriptor-free
AI 理解论文
溯源树
样例
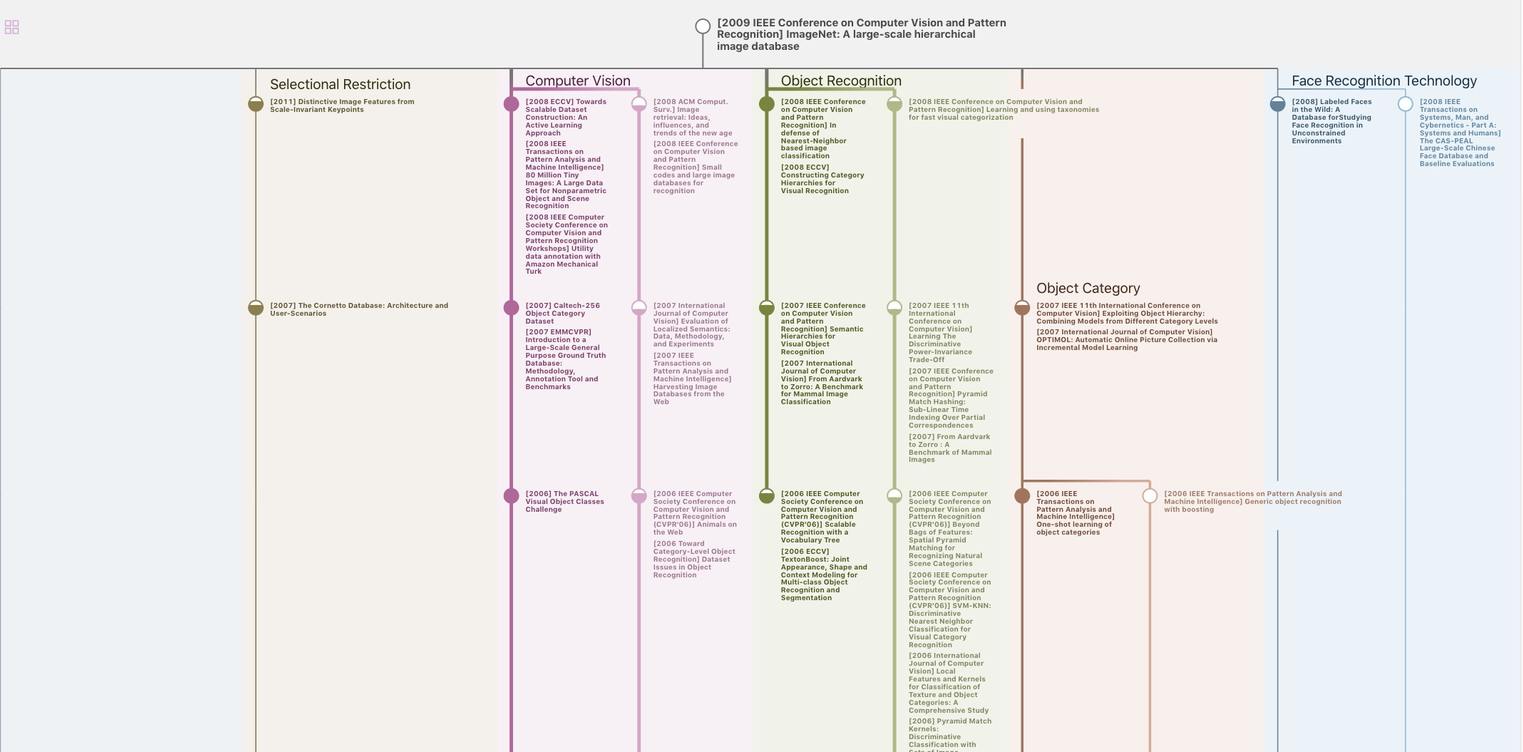
生成溯源树,研究论文发展脉络
Chat Paper
正在生成论文摘要