Jointly-Learnt Networks for Future Action Anticipation via Self-Knowledge Distillation and Cycle Consistency
IEEE Transactions on Circuits and Systems for Video Technology(2023)
摘要
Future action anticipation aims to infer future actions from the observation of a small set of past video frames. In this paper, we propose a novel Jointly-learnt Action Anticipation Network (J-AAN) via Self-Knowledge Distillation (Self-KD) and cycle consistency for future action anticipation. In contrast to the current state-of-the-art methods which anticipate the future actions either directly or recursively, our proposed J-AAN anticipates the future actions jointly in both direct and recursive ways. However, when dealing with future action anticipation, one important challenge to address is the future's uncertainty since multiple action sequences may come from or be followed by the same action. Training an action anticipation model with one-hot-encoded hard labels that assign zero probabilities to incorrect yet semantically similar actions may not handle the uncertain future. To address this challenge, we design a Self-KD mechanism to train our J-AAN, where the J-AAN gradually distills its own knowledge during the training to soften the hard labels to model the uncertainty on future action anticipation. Furthermore, we design a forward and backward action anticipation framework with our proposed J-AAN based on a cyclic consistency constraint. The forward J-AAN anticipates the future actions from the observed past actions, and the backward J-AAN verifies the anticipation of the forward J-AAN by anticipating the past actions from the anticipated future actions. The proposed method outperforms all the latest state-of-the-art action anticipation methods on the Breakfast, 50Salads, and EPIC-Kitchens-55 datasets. This project will be publicly available on https://github.com/MoniruzzamanMd/J-AAN.
更多查看译文
关键词
Future action anticipation,self-knowledge distillation,cycle consistency
AI 理解论文
溯源树
样例
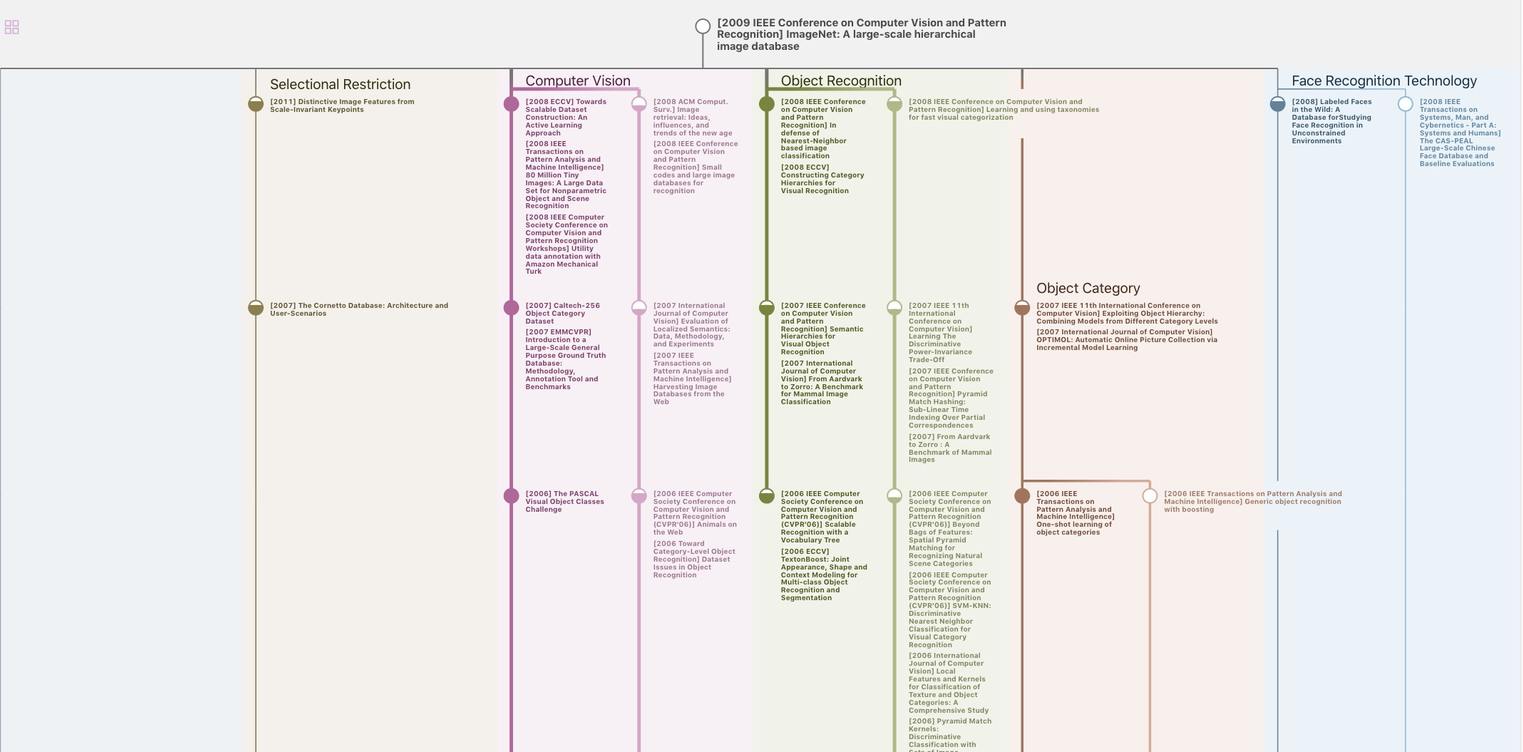
生成溯源树,研究论文发展脉络
Chat Paper
正在生成论文摘要