Bayesian Physics-Informed Neural Networks for Robust System Identification of Power Systems
arxiv(2023)
摘要
This paper introduces for the first time, to the best of our knowledge, the Bayesian Physics-Informed Neural Networks for applications in power systems. Bayesian Physics-Informed Neural Networks (BPINNs) combine the advantages of Physics-Informed Neural Networks (PINNs), being robust to noise and missing data, with Bayesian modeling, delivering a confidence measure for their output. Such a confidence measure can be very valuable for the operation of safety critical systems, such as power systems, as it offers a degree of 'trustworthiness' for the neural network output. This paper applies the BPINNs for robust identification of the system inertia and damping, using a single machine infinite bus system as the guiding example. The goal of this paper is to introduce the concept and explore the strengths and weaknesses of BPINNs compared to existing methods. We compare BPINNs with the PINNs and the recently popular method for system identification, SINDy. We find that BPINNs and PINNs are robust against all noise levels, delivering estimates of the system inertia and damping with significantly lower error compared to SINDy, especially as the noise levels increases.
更多查看译文
关键词
Bayesian Physics-Informed Neural Networks,System Identification,Swing Equation,Power System Dynamics
AI 理解论文
溯源树
样例
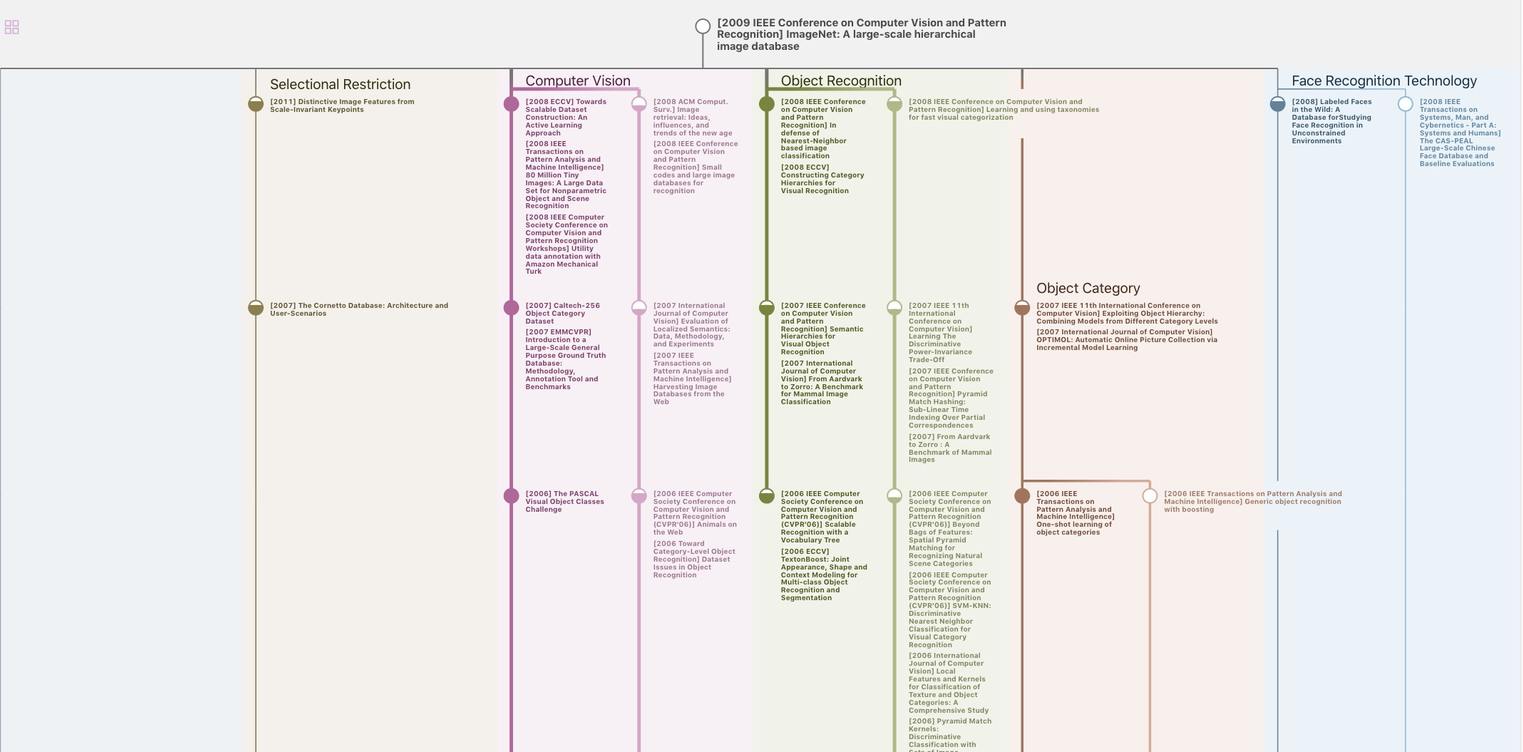
生成溯源树,研究论文发展脉络
Chat Paper
正在生成论文摘要