FETA: Flow-Enhanced Transportation for Anomaly Detection
arxiv(2022)
摘要
Resonant anomaly detection is a promising framework for model-independent searches for new particles. Weakly supervised resonant anomaly detection methods compare data with a potential signal against a template of the Standard Model (SM) background inferred from sideband regions. We propose a means to generate this background template that uses a flow-based model to create a mapping between high-fidelity SM simulations and the data. The flow is trained in sideband regions with the signal region blinded, and the flow is conditioned on the resonant feature (mass) such that it can be interpolated into the signal region. To illustrate this approach, we use simulated collisions from the Large Hadron Collider (LHC) Olympics Dataset. We find that our flow-constructed background method has competitive sensitivity with other recent proposals and can therefore provide complementary information to improve future searches.
更多查看译文
关键词
anomaly detection,transportation,flow-enhanced
AI 理解论文
溯源树
样例
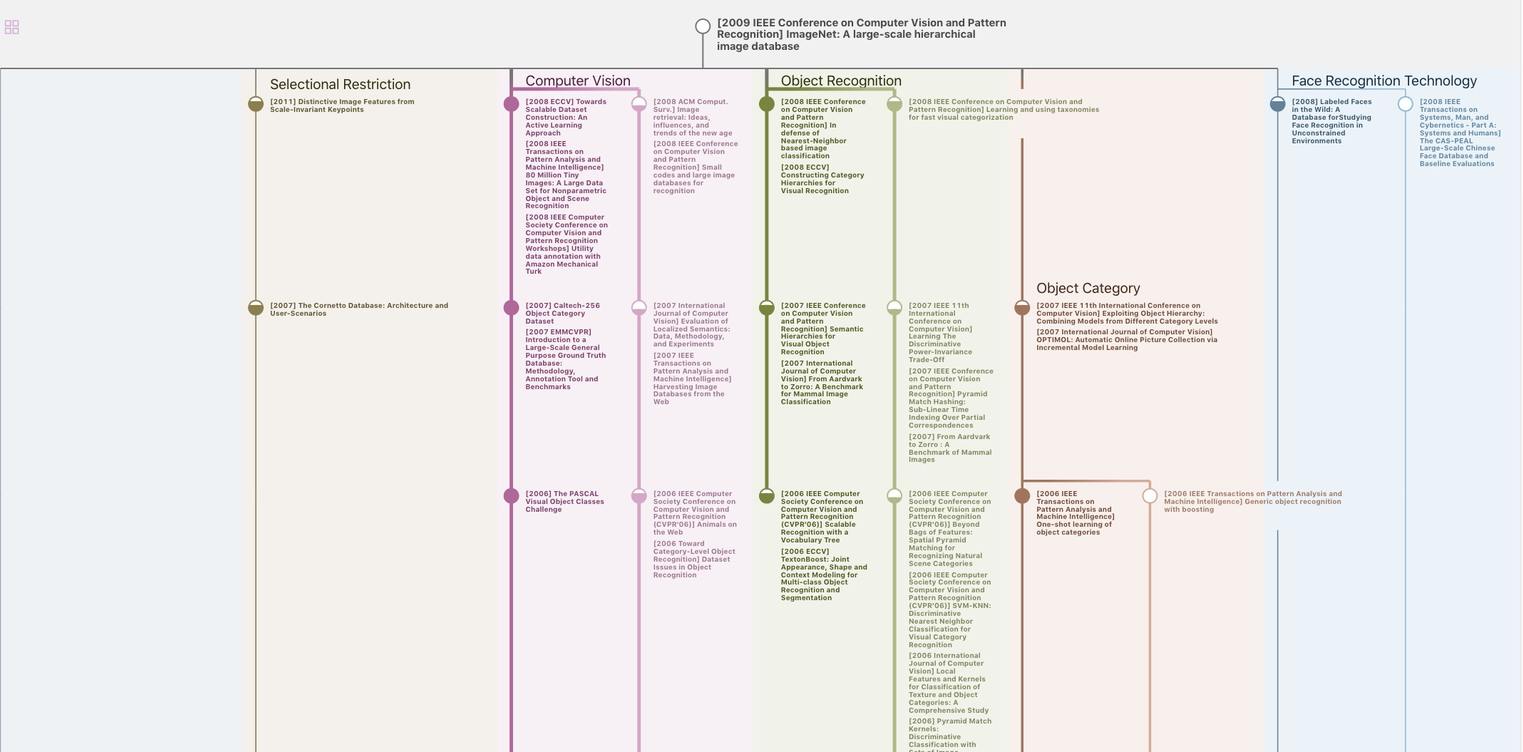
生成溯源树,研究论文发展脉络
Chat Paper
正在生成论文摘要