Correction: Global and local representation collaborative learning for few-shot learning
Journal of Intelligent Manufacturing(2024)
摘要
The objective of few-shot learning (FSL) is to learn a model that can quickly adapt to novel classes with only few examples. Recent works have shown that a powerful representation with a base learner trained in supervised and self-supervised manners has significant advantages over the existing sophisticated FSL algorithms. In this paper, we build on this insight and propose a new framework called global and local representation collaborative learning (GLCL), which combines the complementary advantages of global equivariance and local aggregation. Global equivariance learns the internal structure of data to improve class discrimination, and the local aggregation retains important semantic information to enrich feature representations. In addition, we design a cross-view contrastive learning to promote the consistent learning and implicit exploration of useful knowledge from one another. A simultaneous optimization of these contrasting objectives allows the model to encode informative features while maintaining strong generalization capabilities for new tasks. We demonstrate consistent and substantial performance gains for FSL classification tasks on multiple datasets. Our code is available at https://github.com/zjgans/GLCL .
更多查看译文
关键词
Few-shot learning,Global equivariance,Local aggregation,Contrastive learning,Meta-learning
AI 理解论文
溯源树
样例
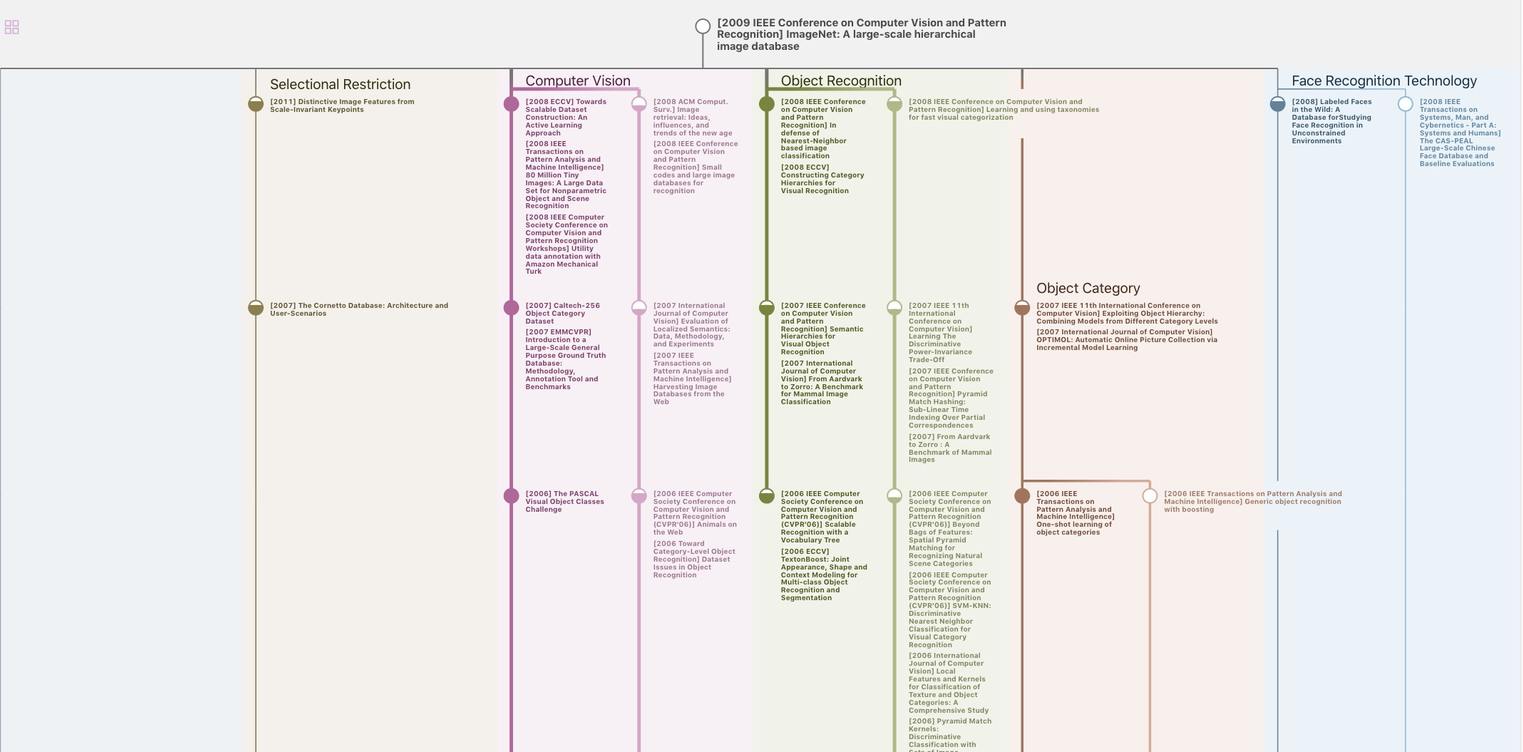
生成溯源树,研究论文发展脉络
Chat Paper
正在生成论文摘要