Neural-Symbolic Inference for Robust Autoregressive Graph Parsing via Compositional Uncertainty Quantification
emnlp 2022(2022)
摘要
Pre-trained seq2seq models excel at graph semantic parsing with rich annotated data, but generalize worse to out-of-distribution (OOD) and long-tail examples. In comparison, symbolic parsers under-perform on population-level metrics, but exhibit unique strength in OOD and tail generalization. In this work, we study compositionality-aware approach to neural-symbolic inference informed by model confidence, performing fine-grained neural-symbolic reasoning at subgraph level (i.e., nodes and edges) and precisely targeting subgraph components with high uncertainty in the neural parser. As a result, the method combines the distinct strength of the neural and symbolic approaches in capturing different aspects of the graph prediction, leading to well-rounded generalization performance both across domains and in the tail. We empirically investigate the approach in the English Resource Grammar (ERG) parsing problem on a diverse suite of standard in-domain and seven OOD corpora. Our approach leads to 35.26% and 35.60% error reduction in aggregated SMATCH score over neural and symbolic approaches respectively, and 14% absolute accuracy gain in key tail linguistic categories over the neural model, outperforming prior state-of-art methods that do not account for compositionality or uncertainty.
更多查看译文
关键词
robust autoregressive graph parsing,inference,uncertainty,neural-symbolic
AI 理解论文
溯源树
样例
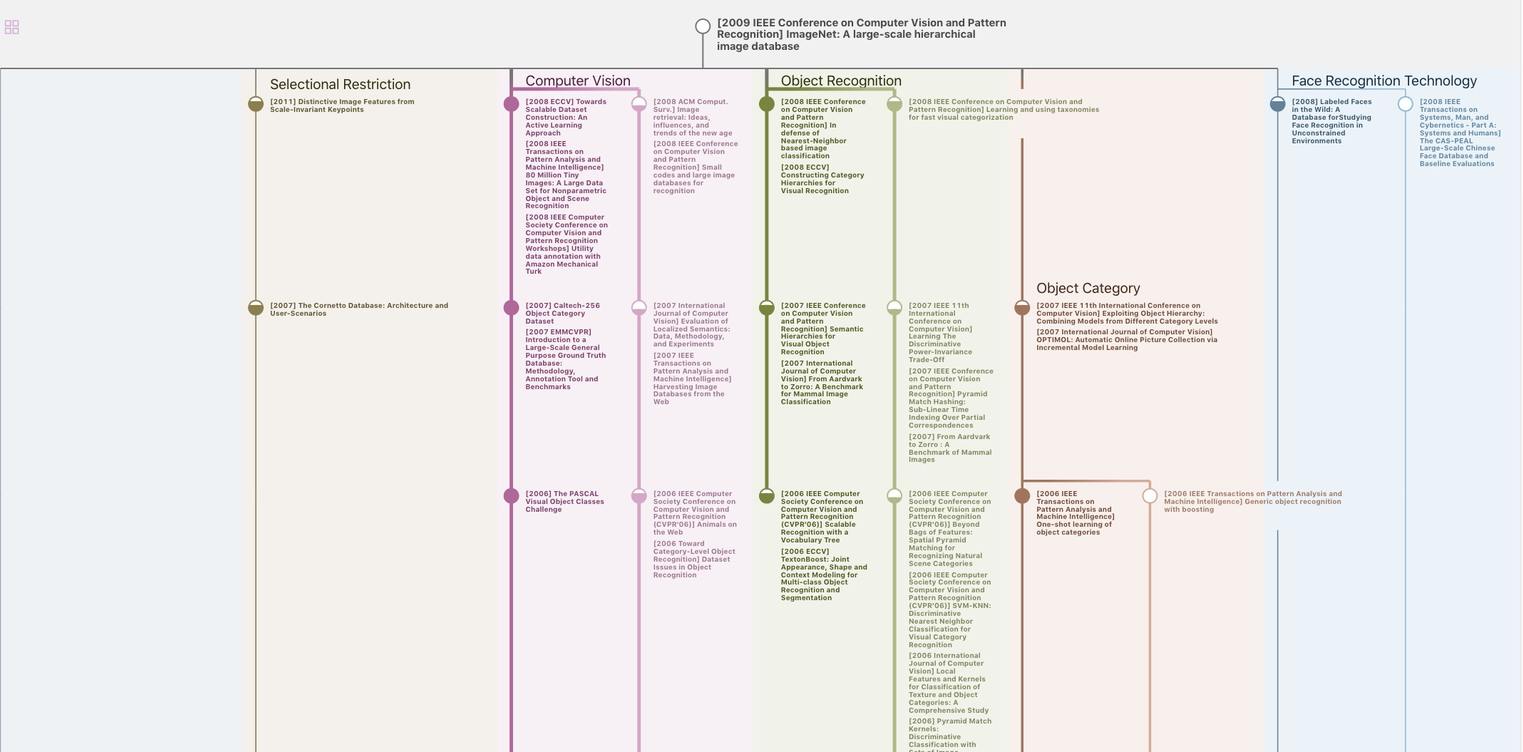
生成溯源树,研究论文发展脉络
Chat Paper
正在生成论文摘要