Debiasing Pretrained Text Encoders by Paying Attention to Paying Attention
emnlp 2022(2022)
摘要
Natural Language Processing (NLP) models are found to exhibit discriminatory stereotypes across many social constructs, e.g. gender and race. In comparison to the progress made in reducing bias from static word embeddings, fairness in sentence-level text encoders received little consideration despite their wider applicability in contemporary NLP tasks. In this paper, we propose a debiasing method for pre-trained text encoders that both reduces social stereotypes, and inflicts next to no semantic damage. Unlike previous studies that directly manipulate the embeddings, we suggest to dive deeper into the operation of these encoders, and pay more attention to the way they pay attention to different social groups. We find that stereotypes are also encoded in the attention layer. Then, we work on model debiasing by redistributing the attention scores of a text encoder such that it forgets any preference to historically advantaged groups, and attends to all social classes with the same intensity. Our experiments confirm that reducing bias from attention effectively mitigates it from the model’s text representations.
更多查看译文
关键词
pretrained text encoders,attention
AI 理解论文
溯源树
样例
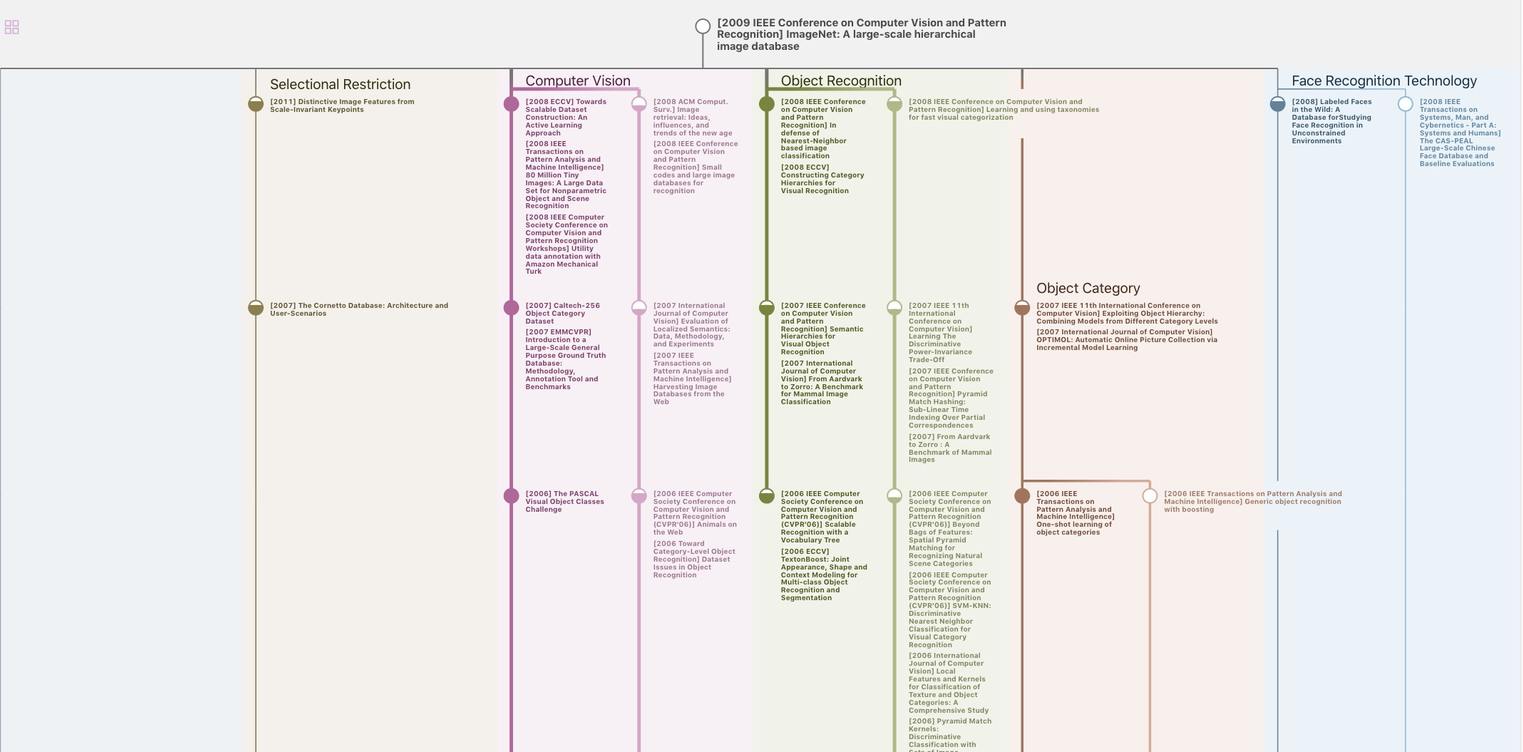
生成溯源树,研究论文发展脉络
Chat Paper
正在生成论文摘要