Learning Subgrid-scale Models with Neural Ordinary Differential Equations
arxiv(2023)
摘要
We propose a new approach to learning the subgrid-scale model when simulating partial differential equations (PDEs) solved by the method of lines and their representation in chaotic ordinary differential equations, based on neural ordinary differential equations (NODEs). Solving systems with fine temporal and spatial grid scales is an ongoing computational challenge, and closure models are generally difficult to tune. Machine learning approaches have increased the accuracy and efficiency of computational fluid dynamics solvers. In this approach neural networks are used to learn the coarse- to fine-grid map, which can be viewed as subgrid-scale parameterization. We propose a strategy that uses the NODE and partial knowledge to learn the source dynamics at a continuous level. Our method inherits the advantages of NODEs and can be used to parameterize subgrid scales, approximate coupling operators, and improve the efficiency of low-order solvers. Numerical results with the two-scale Lorenz 96 ODE, the convection-diffusion PDE, and the viscous Burgers' PDE are used to illustrate this approach.
更多查看译文
关键词
subgrid-scale subgrid-scale models,neural ordinary differential
AI 理解论文
溯源树
样例
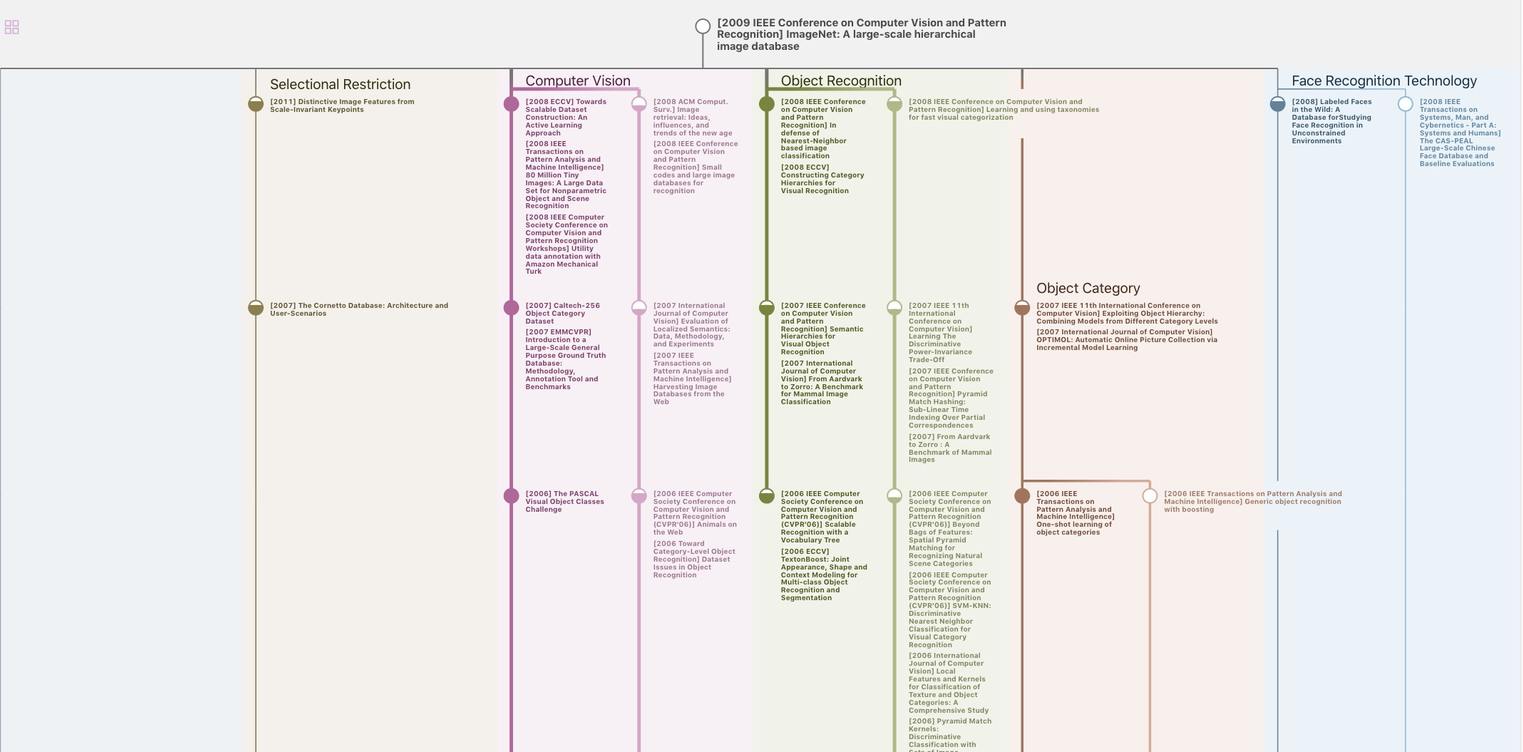
生成溯源树,研究论文发展脉络
Chat Paper
正在生成论文摘要