TV‐L1 Ordinal Logistic Regression Reveals New Morphometric Patterns Related to Parkinsonian Symptom Severity: An ENIGMA‐PD study
Alzheimer's & Dementia(2022)
摘要
Abstract Background Parkinson’s disease (PD) is heterogeneous, both phenotypically and in terms of temporal progression. The Hoehn and Yahr (HY) scale is a well‐established PD staging approach, and identifies 5 stages of the disease. Morphometric effects in deep gray matter regions of the brain associated with HY stages are complex; a recent large‐scale ENIGMA‐PD study showed higher local subcortical volumes in early HY stages relative to controls, followed by a precipitous decrease after stage 2 [1]. This finding motivates a closer look at fine‐level morphometry beyond gross volume measures. Here, we developed and applied a novel machine learning algorithm to reveal the subcortical shape signatures of HY staging. Method We computed shape features in 7 bilateral subcortical regions [2] based on T1‐weighted MRI data from 2,322 PD subjects and 1,207 controls from 20 ENIGMA‐PD cohorts (HY stages in Table 1) . We developed a sparse, spatially coherent (total variation/TV‐L1) ordinal linear logistic classifier [3] to predict HY stages with a single linear model. We applied the model to vertex‐wise medial thickness features. We optimized regularization parameters for balanced recall (sensitivity) and precision using a 4‐fold cross‐validation grid search. Very low numbers of HY4 and HY5 samples necessitated merging stages 3‐5 into one category. For comparison, we also trained 4 binary TV‐L1 logit models on the same features [4], discriminating (1) PD‐Control; (2) HY1‐HY2; (3) HY1‐HY345; (4) HY2‐HY345, using ROC area‐under‐the‐curve (AUC) evaluation. Result Across‐stage mean out‐of‐sample precision and recall were 0.43, and 0.393, respectively (chance=0.33). Table 2 shows the confusion matrix and precision/recall for each HY stage. All models’ linear coefficient maps are displayed in Figures 1,2 . Binary classification ROC‐AUC was 0.66 for PD‐Control, and ranged from 0.62 to 0.73 for HY prediction ( Figure 2 ). Conclusion We developed an ordit machine learning model for morphometric shape‐based ordinal classification of disease stages, training it for Parkinson’s Disease Hoehn and Yahr stage prediction on a large MRI collection. Performance was substantially above chance. Model weight maps indicate early increased thalamic thickness, followed by a complex thinning pattern associated with later HY stages.
更多查看译文
关键词
parkinsonian symptom severity,ordinal logistic regression
AI 理解论文
溯源树
样例
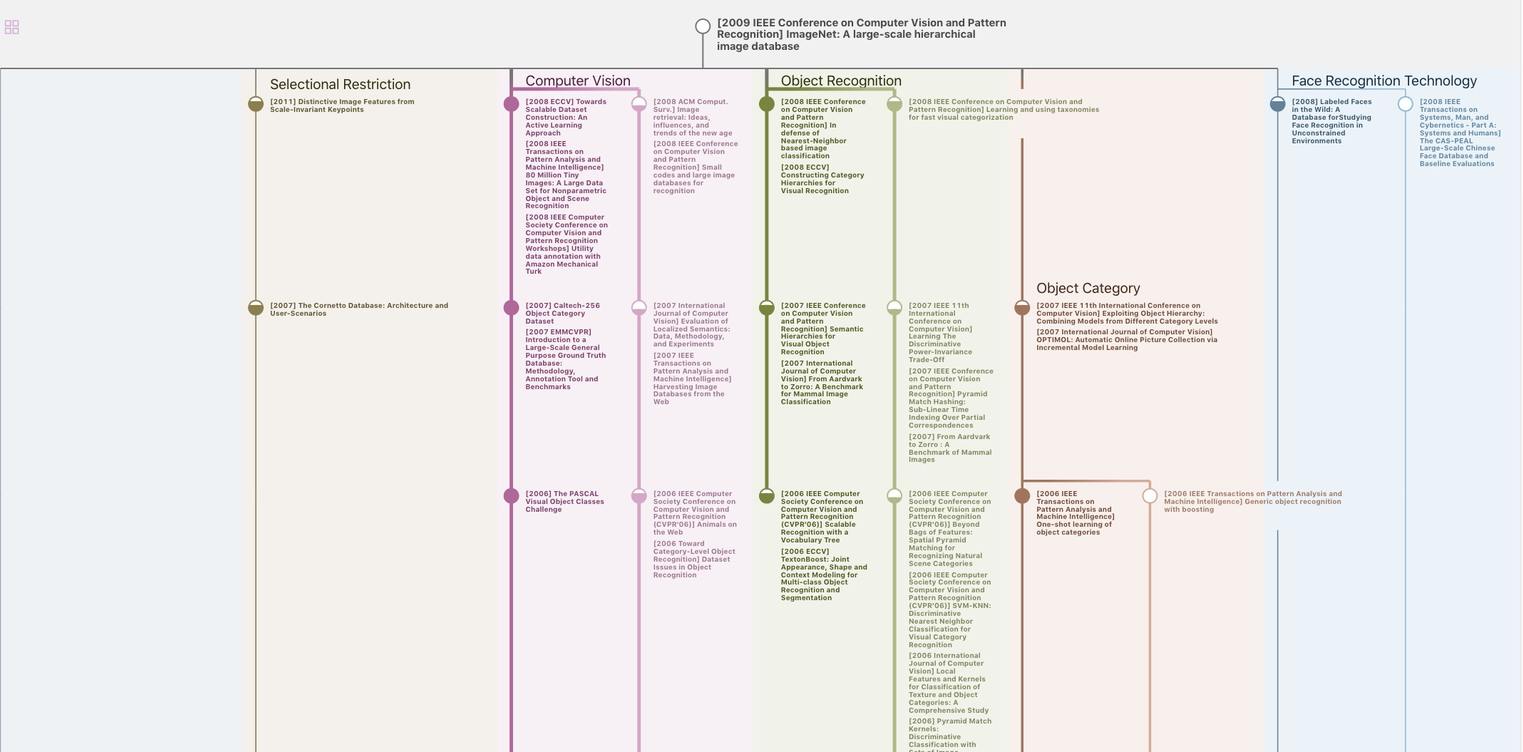
生成溯源树,研究论文发展脉络
Chat Paper
正在生成论文摘要