Inconsistent Partitioning and Unproductive Feature Associations Yield Idealized Radiomic Models
Radiology(2023)
摘要
Background: Radiomics is the extraction of predefined mathematic features from medical images for the prediction of variables of clinical interest. While some studies report superlative accuracy of radiomic machine learning (ML) models, the published methodology is often incomplete, and the results are rarely validated in external testing data sets.Purpose: To characterize the type, prevalence, and statistical impact of methodologic errors present in radiomic ML studies.Materials and Methods: Radiomic ML publications were reviewed for the presence of performance-inflating methodologic flaws. Common flaws were subsequently reproduced with randomly generated features interpolated from publicly available radiomic data sets to demonstrate the precarious nature of reported findings.Results: In an assessment of radiomic ML publications, the authors uncovered two general categories of data analysis errors: incon-sistent partitioning and unproductive feature associations. In simulations, the authors demonstrated that inconsistent partitioning augments radiomic ML accuracy by 1.4 times from unbiased performance and that correcting for flawed methodologic results in areas under the receiver operating characteristic curve approaching a value of 0.5 (random chance). With use of randomly generated features, the authors illustrated that unproductive associations between radiomic features and gene sets can imply false causality for biologic phenomenon.Conclusion: Radiomic machine learning studies may contain methodologic flaws that undermine their validity. This study provides a review template to avoid such flaws.
更多查看译文
AI 理解论文
溯源树
样例
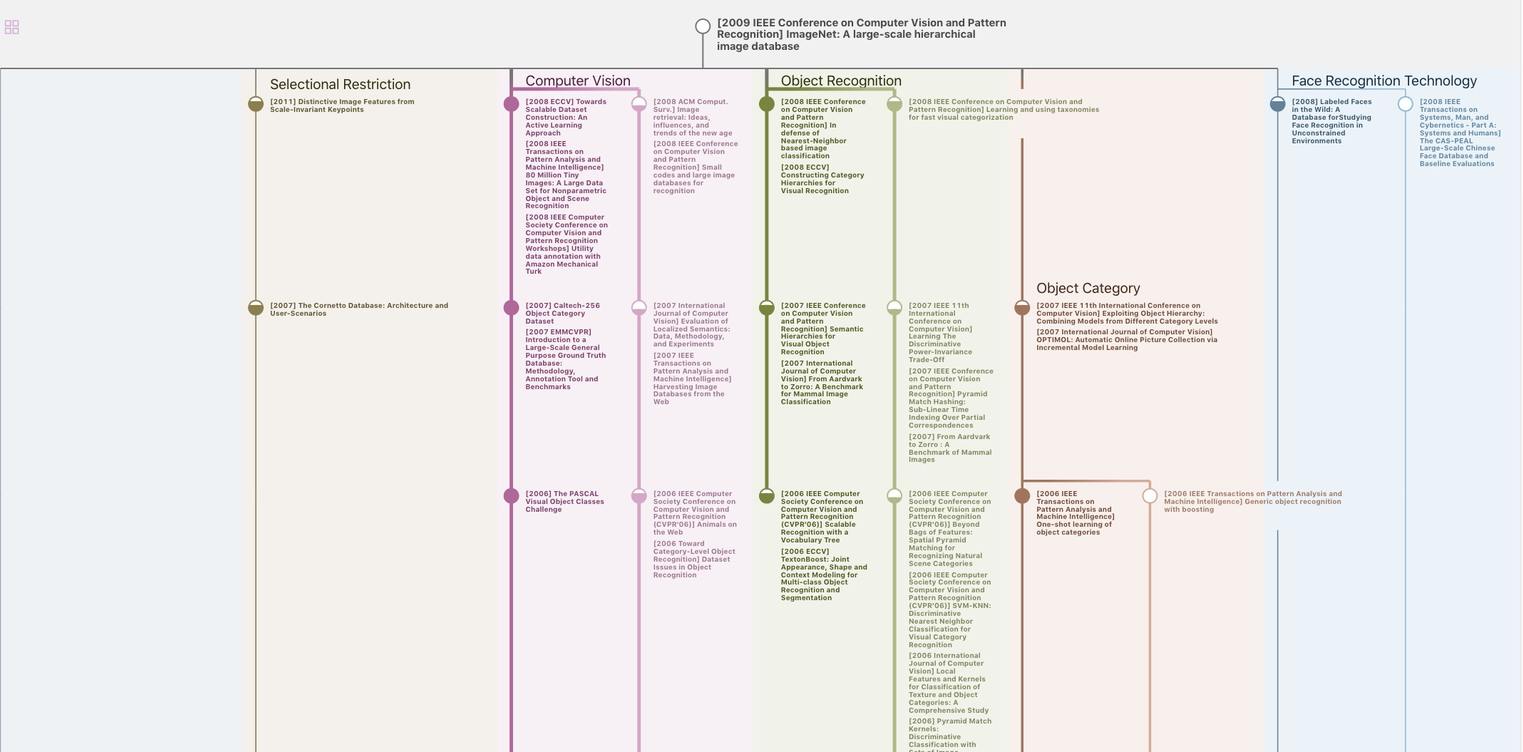
生成溯源树,研究论文发展脉络
Chat Paper
正在生成论文摘要