Charging demand prediction in Beijing based on real-world electric vehicle data
Journal of Energy Storage(2023)
摘要
The accurate estimation and prediction of charging demand play an essential role in charging infrastructure planning, power grid laying and efficient operations. In this paper, three-month real-world travel and charging records of 25,489 electric passenger vehicles in Beijing are utilized to quantitatively study the travel patterns and charging behaviors of electric vehicles and support charging demand prediction. A "multi-level & multi -dimension" travel and charging characteristic parameters extraction framework is proposed with >60 electric vehicle behavior characteristic parameters extracted from time, spatial, and energy dimensions. Then a two-step GMM and K-means-based clustering model is proposed to cluster the electric vehicle users into six categories with different usage habits, attributes, and characteristics. A trip-chain-simulation-based charging demand prediction model is further established to generate a week-period continuous activities simulation for specific category vehicles, in which the interdependences of travel and charging characteristic parameters are considered to make the trip chain simulation closer to reality. The city charging demands are aggregated from individual charging prediction results at a spatial resolution of 0.46 km and time resolution of 15 min quantitatively; the spatio-temporal aggregation results show that the proposed model can realize high accuracy prediction for real-world charging demands. The results are expected to provide the foundation for research on charging infrastructure planning and the impact of charging behaviors on the grid load.
更多查看译文
关键词
Electric vehicle,Trip chain,User clustering,Charging demand
AI 理解论文
溯源树
样例
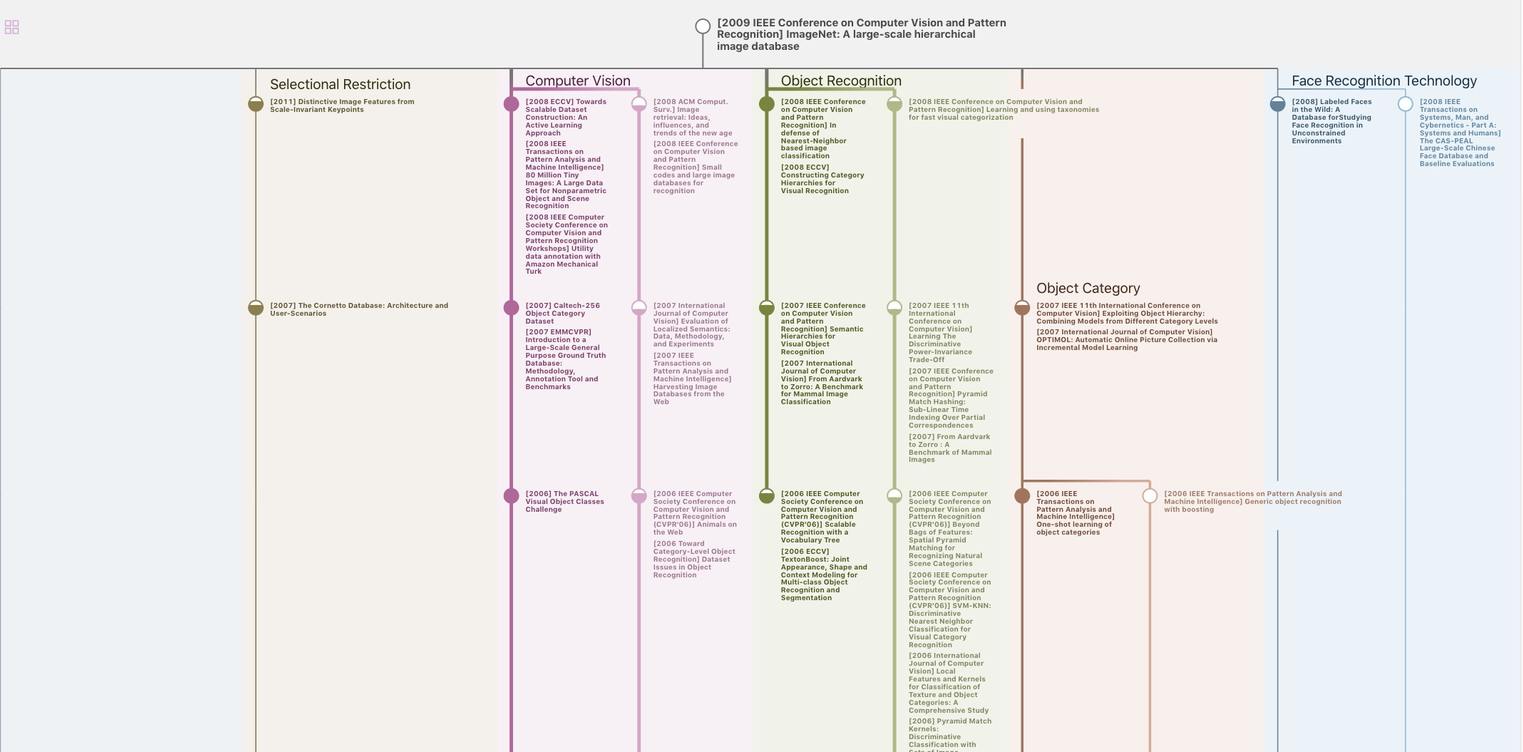
生成溯源树,研究论文发展脉络
Chat Paper
正在生成论文摘要