Synaptic Dynamics Realize First-order Adaptive Learning and Weight Symmetry
arxiv(2022)
摘要
Gradient-based first-order adaptive optimization methods such as the Adam optimizer are prevalent in training artificial networks, achieving the state-of-the-art results. This work attempts to answer the question whether it is viable for biological neural systems to adopt such optimization methods. To this end, we demonstrate a realization of the Adam optimizer using biologically-plausible mechanisms in synapses. The proposed learning rule has clear biological correspondence, runs continuously in time, and achieves performance to comparable Adam's. In addition, we present a new approach, inspired by the predisposition property of synapses observed in neuroscience, to circumvent the biological implausibility of the weight transport problem in backpropagation (BP). With only local information and no separate training phases, this method establishes and maintains weight symmetry in the forward and backward signaling paths, and is applicable to the proposed biologically plausible Adam learning rule. These mechanisms may shed light on the way in which biological synaptic dynamics facilitate learning.
更多查看译文
关键词
synapses,optimizer,biologically plausible,weight transport,Adam
AI 理解论文
溯源树
样例
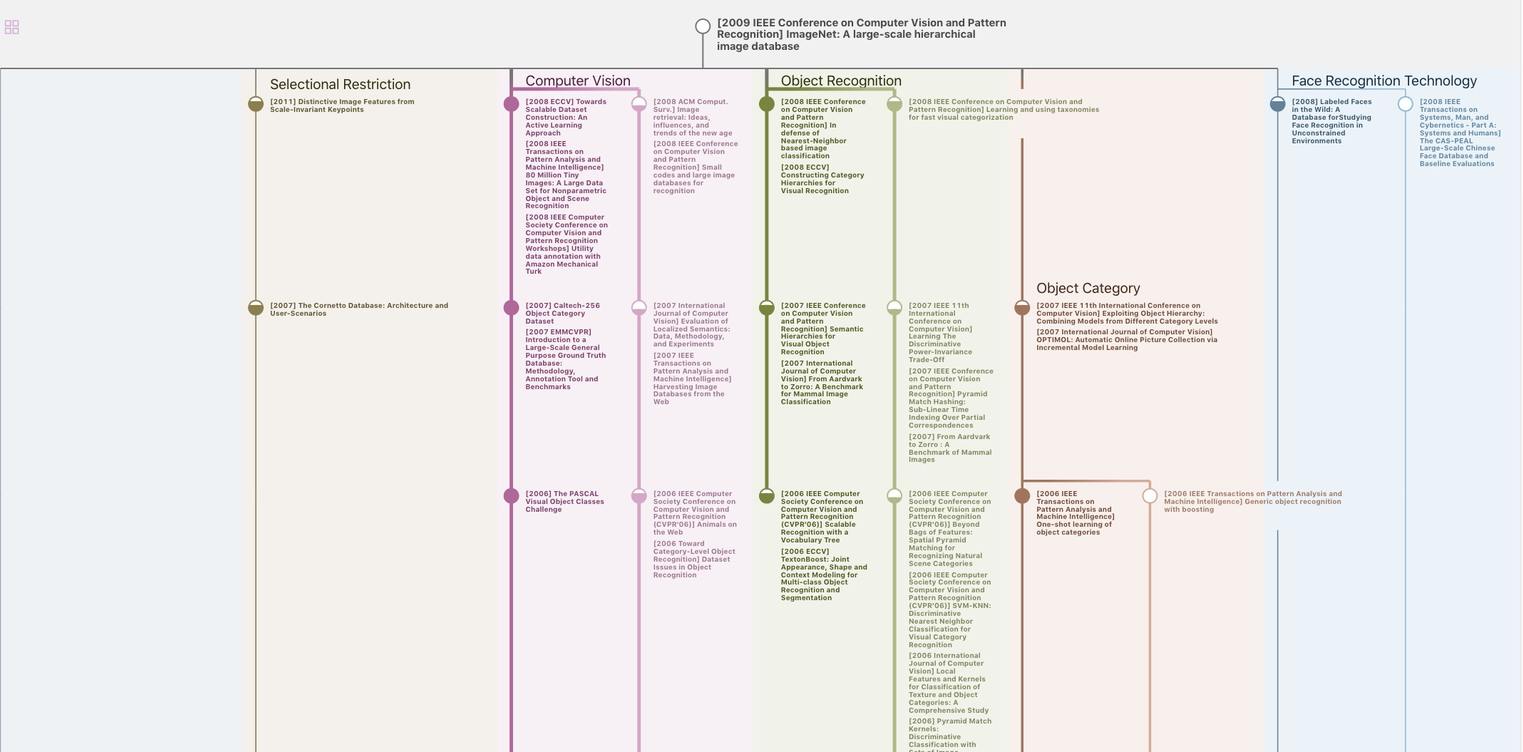
生成溯源树,研究论文发展脉络
Chat Paper
正在生成论文摘要