LaSQuE: Improved Zero-Shot Classification from Explanations Through Quantifier Modeling and Curriculum Learning
conf_acl(2022)
摘要
A hallmark of human intelligence is the ability to learn new concepts purely from language. Several recent approaches have explored training machine learning models via natural language supervision. However, these approaches fall short in leveraging linguistic quantifiers (such as 'always' or 'rarely') and mimicking humans in compositionally learning complex tasks. Here, we present LaSQuE, a method that can learn zero-shot classifiers from language explanations by using three new strategies - (1) modeling the semantics of linguistic quantifiers in explanations (including exploiting ordinal strength relationships, such as 'always' > 'likely'), (2) aggregating information from multiple explanations using an attention-based mechanism, and (3) model training via curriculum learning. With these strategies, LaSQuE outperforms prior work, showing an absolute gain of up to 7% in generalizing to unseen real-world classification tasks.
更多查看译文
关键词
curriculum learning,quantifier modeling,zero-shot
AI 理解论文
溯源树
样例
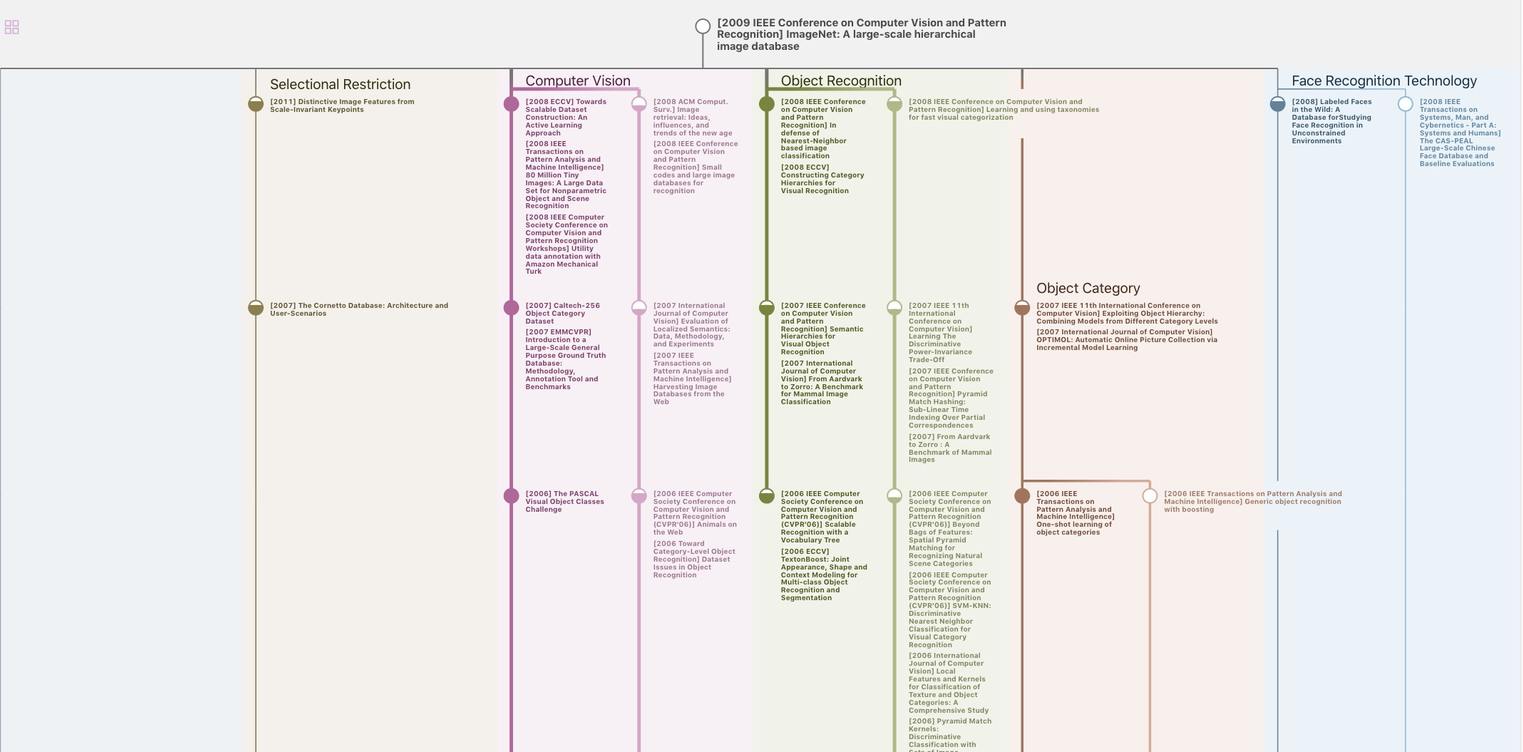
生成溯源树,研究论文发展脉络
Chat Paper
正在生成论文摘要