Learning for Vehicle-to-Vehicle Cooperative Perception under Lossy Communication
arxiv(2023)
摘要
Deep learning has been widely used in the perception (e.g., 3D object detection) of intelligent vehicle driving. Due to the beneficial Vehicle-to-Vehicle (V2V) communication, the deep learning based features from other agents can be shared to the ego vehicle so as to improve the perception of the ego vehicle. It is named as Cooperative Perception in the V2V research, whose algorithms have been dramatically advanced recently. However, all the existing cooperative perception algorithms assume the ideal V2V communication without considering the possible lossy shared features because of the Lossy Communication (LC) which is common in the complex real-world driving scenarios. In this paper, we first study the side effect (e.g., detection performance drop) by the lossy communication in the V2V Cooperative Perception, and then we propose a novel intermediate LC-aware feature fusion method to relieve the side effect of lossy communication by a LC-aware Repair Network (LCRN) and enhance the interaction between the ego vehicle and other vehicles by a specially designed V2V Attention Module (V2VAM) including intra-vehicle attention of ego vehicle and uncertainty-aware inter-vehicle attention. The extensive experiment on the public cooperative perception dataset OPV2V (based on digital-twin CARLA simulator) demonstrates that the proposed method is quite effective for the cooperative point cloud based 3D object detection under lossy V2V communication.
更多查看译文
关键词
Three-dimensional displays, Vehicular ad hoc networks, Object detection, Feature extraction, Point cloud compression, Maintenance engineering, Laser radar, Deep learning, vehicle-to-vehicle cooperative perception, 3D object detection, lossy communication, digital twin
AI 理解论文
溯源树
样例
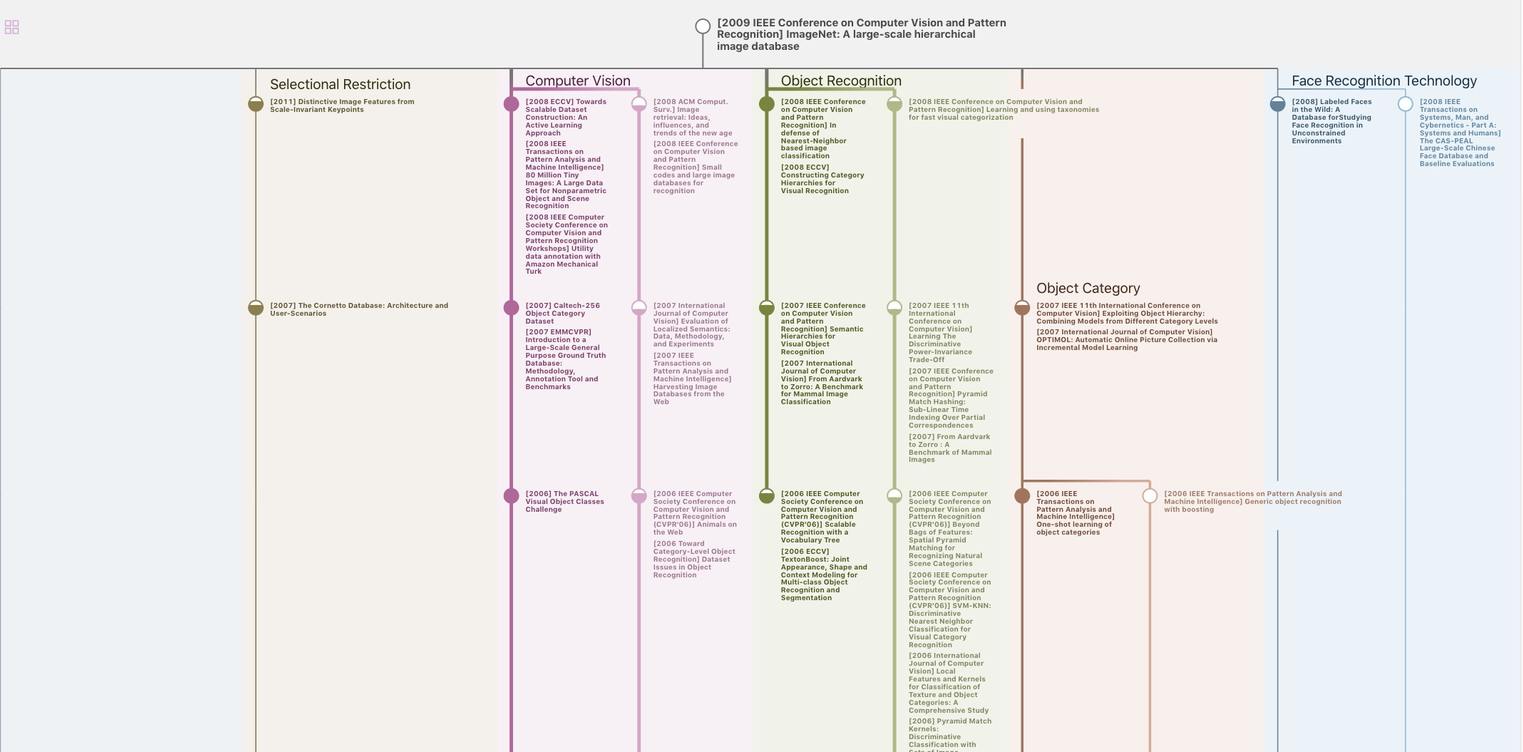
生成溯源树,研究论文发展脉络
Chat Paper
正在生成论文摘要