DeepDFA: Dataflow Analysis-Guided Efficient Graph Learning for Vulnerability Detection
arxiv(2022)
摘要
Deep learning-based vulnerability detection models have recently been shown to be effective and, in some cases, outperform static analysis tools. However, the highest-performing approaches use token-based transformer models, which do not leverage domain knowledge. Classical program analysis techniques such as dataflow analysis can detect many types of bugs and are the most commonly used methods in practice. Motivated by the causal relationship between bugs and dataflow analysis, we present DeepDFA, a dataflow analysis-guided graph learning framework and embedding that uses program semantic features for vulnerability detection. We show that DeepDFA is performant and efficient. DeepDFA ranked first in recall, first in generalizing over unseen projects, and second in F1 among all the state-of-the-art models we experimented with. It is also the smallest model in terms of the number of parameters, and was trained in 9 minutes, 69x faster than the highest-performing baseline. DeepDFA can be used with other models. By integrating LineVul and DeepDFA, we achieved the best vulnerability detection performance of 96.4 F1 score, 98.69 precision, and 94.22 recall.
更多查看译文
关键词
deep learning,vulnerability detection,dataflow analysis,program analysis
AI 理解论文
溯源树
样例
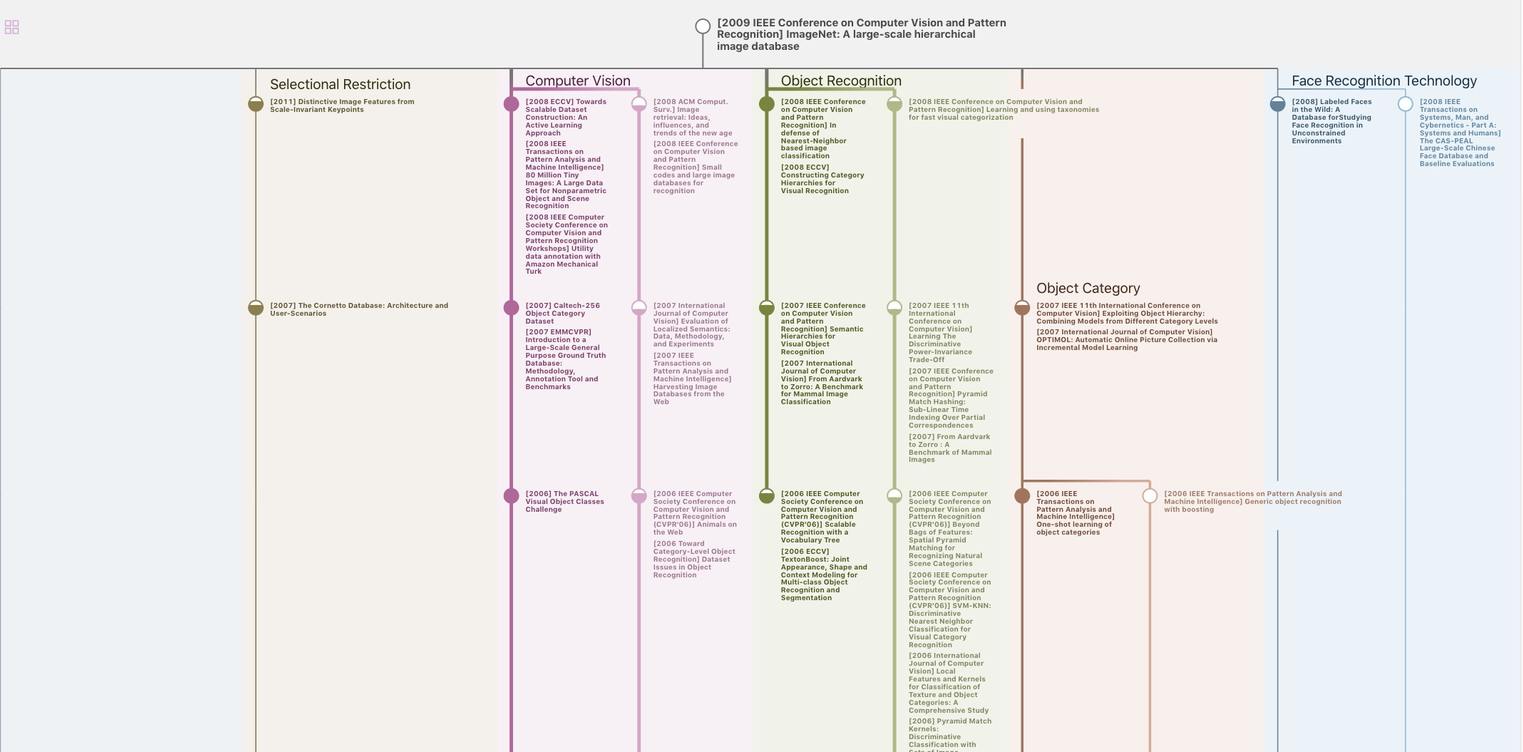
生成溯源树,研究论文发展脉络
Chat Paper
正在生成论文摘要