TDA-Clustering Strategies for the Characterization of Brain Organoids.
EPIMI/ML-CDS@MICCAI(2022)
摘要
We propose to use Topological Data Analysis (TDA) to characterize the morphological development of brain organoids. We combine TDA with clustering strategies to characterize the morphology of three developmental stages of segmented brain organoid images. We calculate a linear regression of the H1 feature diagrams as well as entropy, dispersion, and average persistence of H0 and H1 features separately for each developmental stage. We also compute pairwise average Wasserstein distances between features, and within and inter clusters for each developmental stage. To explore all feature vectors from each group, we calculate K-L divergence on the t-SNE reduction of the TDA results. Early stages are characterized by a persistence diagram with a low slope and intercept, a high entropy within features, and a high Wasserstein distance between clusters near and far from the origin. The opposite is true for later stages. K-L divergences are particularly high between mid-stages and early or late stages, and punctually between some clusters. Results highlight specific morphological patterns at 14 days, corresponding to neuroepithelial formations.
更多查看译文
关键词
brain,tda-clustering
AI 理解论文
溯源树
样例
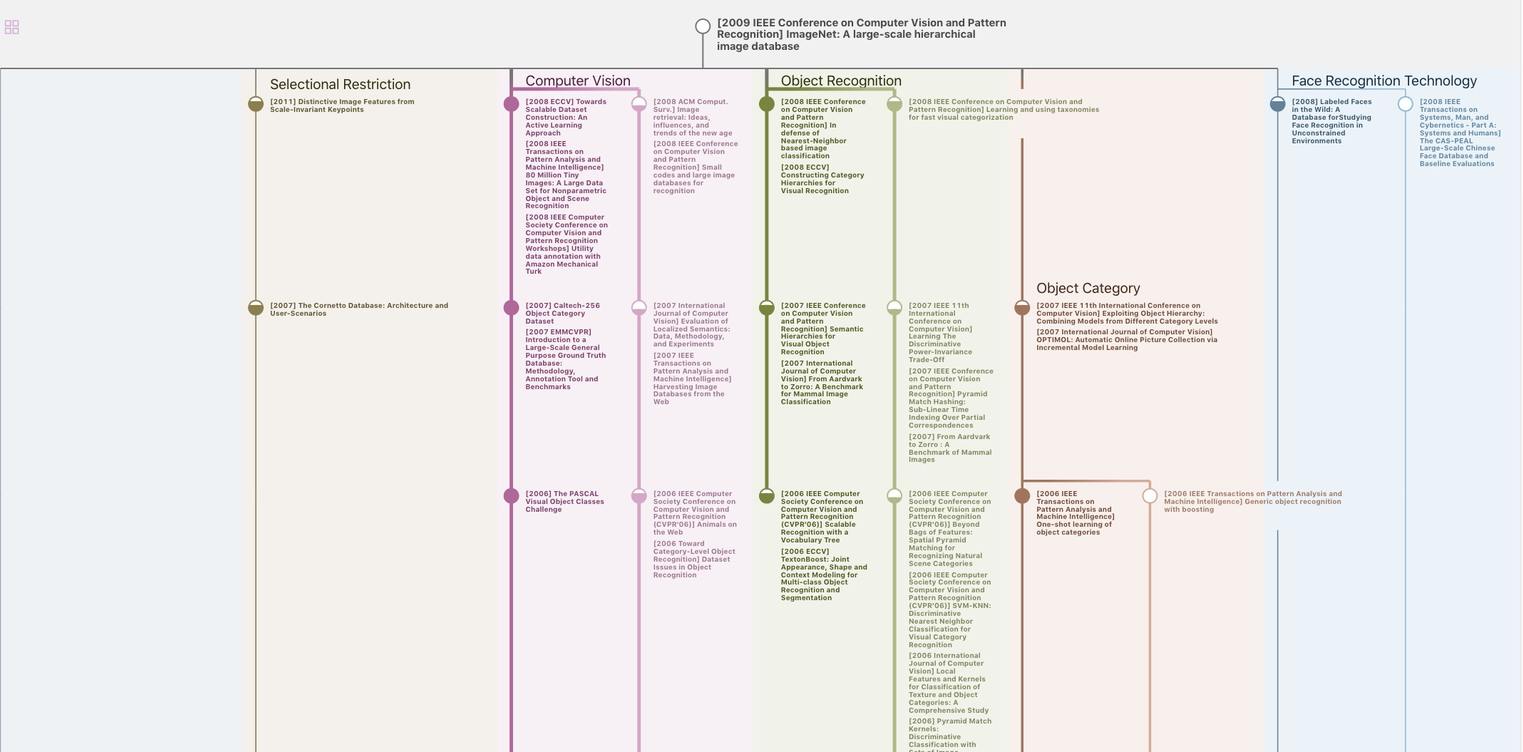
生成溯源树,研究论文发展脉络
Chat Paper
正在生成论文摘要