Deep Multi-Task Learning for an Autoencoder-Regularized Semantic Segmentation of Fundus Retina Images
Mathematics(2022)
摘要
Automated segmentation of retinal blood vessels is necessary for the diagnosis, monitoring, and treatment planning of the disease. Although current U-shaped structure models have achieved outstanding performance, some challenges still emerge due to the nature of this problem and mainstream models. (1) There does not exist an effective framework to obtain and incorporate features with different spatial and semantic information at multiple levels. (2) The fundus retina images coupled with high-quality blood vessel segmentation are relatively rare. (3) The information on edge regions, which are the most difficult parts to segment, has not received adequate attention. In this work, we propose a novel encoder–decoder architecture based on the multi-task learning paradigm to tackle these challenges. The shared image encoder is regularized by conducting the reconstruction task in the VQ-VAE (Vector Quantized Variational AutoEncoder) module branch to improve the generalization ability. Meanwhile, hierarchical representations are generated and integrated to complement the input image. The edge attention module is designed to make the model capture edge-focused feature representations via deep supervision, focusing on the target edge regions that are most difficult to recognize. Extensive evaluations of three publicly accessible datasets demonstrate that the proposed model outperforms the current state-of-the-art methods.
更多查看译文
关键词
retinal vessel segmentation,VQ-VAE,edge attention
AI 理解论文
溯源树
样例
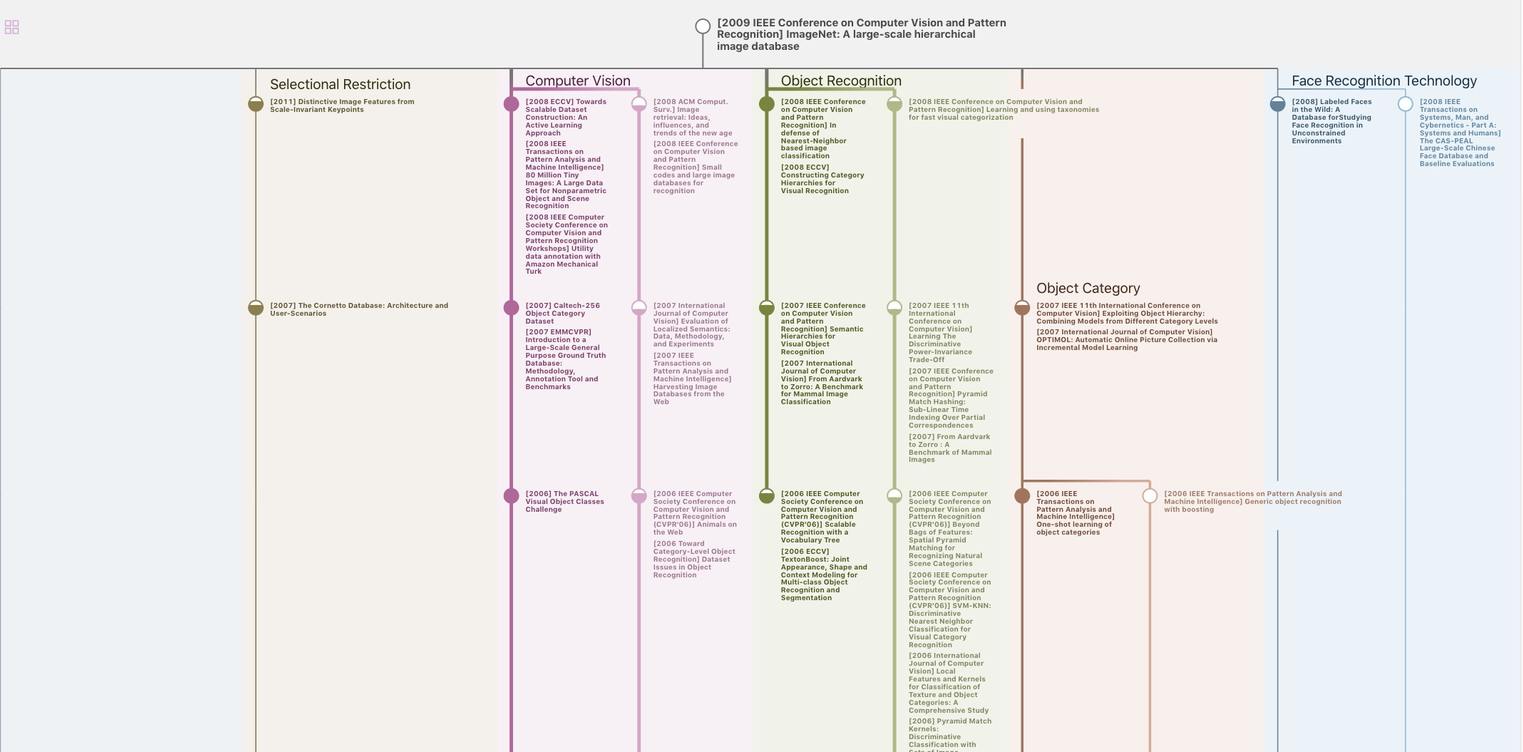
生成溯源树,研究论文发展脉络
Chat Paper
正在生成论文摘要