Integrated condition-based maintenance and multi-item lot-sizing with stochastic demand
Journal of Industrial and Management Optimization(2023)
摘要
This paper studies the problem of integrated lot-sizing and maintenance decision making in case of multiple products and stochastic demand. The problem is formulated as a Markov decision process, in which the goal is to find a joint production and maintenance policy that minimizes the long run expected total discounted cost. Therefore, the classic Q-learning algorithm is adopted, and a decomposition-based approximate Q-value heuristic is developed to obtain near-optimal solutions in a reasonable time. To accelerate the convergence of the Q-learning algorithm, a hybrid Q-learning method is proposed in which the Q-values are initiated by the output of the decomposition-based approximate Q-value heuristic. The numerical experiments reveal that the approximate Q-value heuristic is outperformed by the classic and hybrid Q-learning algorithms in terms of accuracy and that the hybrid Q-learning method converges much faster than the classic Q-learning method. However, these so-called tabular methods do not scale to larger problems with more than four products. Hence, based on the problem structure, three state aggregation schemes are developed and applied to the Q-learning algorithm to solve the large-scale problems. The numerical study demonstrates that Q-learning with the third state aggregation scheme performs nearly as good as the hybrid Q-learning method while significantly reducing the computational time and being scalable to large-scale problems.
更多查看译文
关键词
Condition-based maintenance,Markov decision process,inventory,multi-product,lot-sizing,stochastic demand,reinforcement learning
AI 理解论文
溯源树
样例
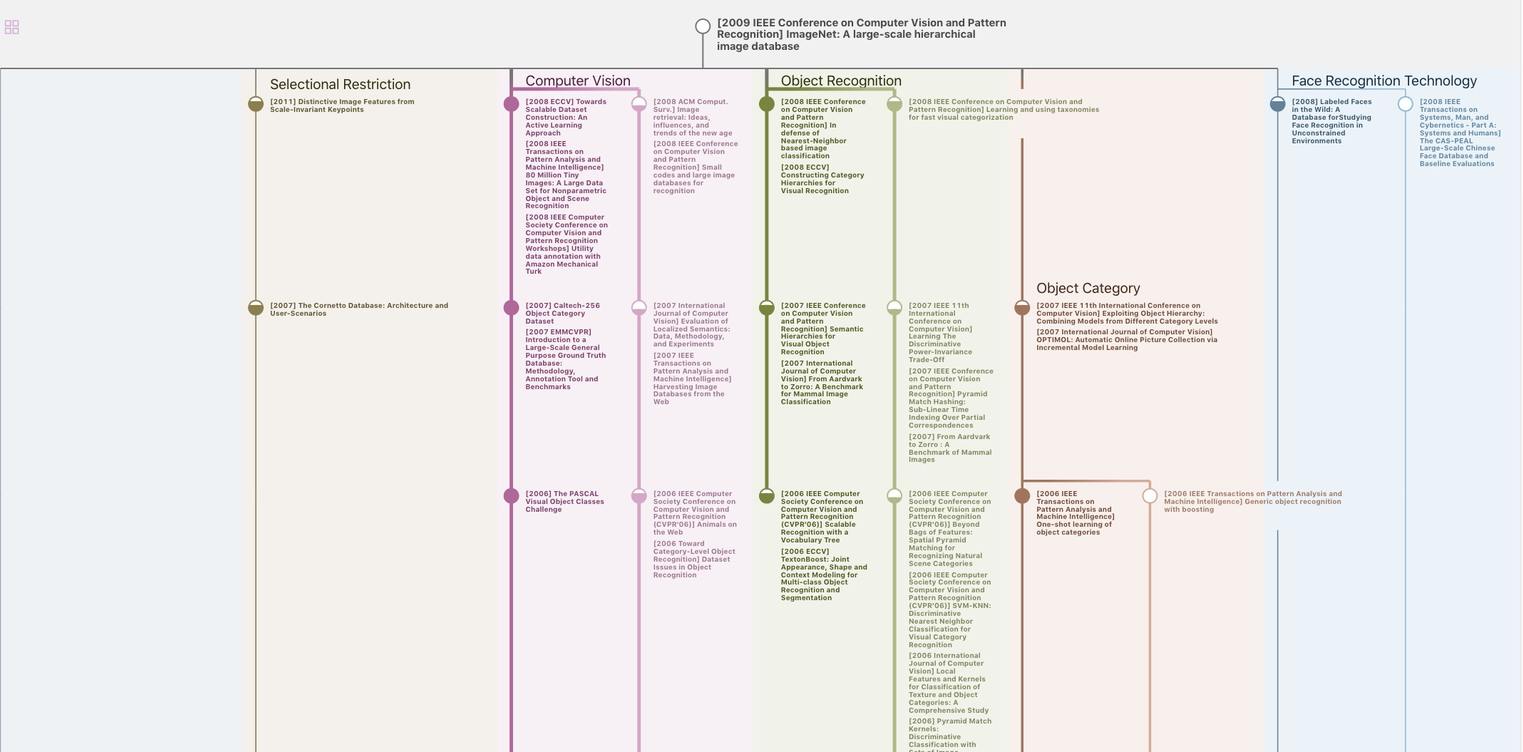
生成溯源树,研究论文发展脉络
Chat Paper
正在生成论文摘要