Forecasting the Geomagnetic Activity Several Days in Advance Using Neural Networks Driven by Solar EUV Imaging
JOURNAL OF GEOPHYSICAL RESEARCH-SPACE PHYSICS(2022)
摘要
Many models of the near-Earth's space environment (radiation belts, ionosphere, upper atmosphere, etc.) are driven by geomagnetic indices, representing the state of disturbance of the Earth's magnetosphere. Over the past decade, machine learning-based methods for forecasting geomagnetic indices from near-Earth solar wind parameters have become popular in the space weather community. These methods often prove to be very accurate and skilled. However, these approaches have the notable drawback of being effective in an operational context only for limited forecasting horizons (often up to a couple of hours ahead at best). In order to increase this prediction horizon, we introduce SERENADE, a novel deep learning-based proof-of-concept model using images delivered by the Atmospheric Imaging Assembly instrument onboard the Solar Dynamics Observatory spacecraft to directly provide probabilistic forecasts of the daily maximum of the geomagnetic index Kp up to a few days ahead. We show in particular that SERENADE is able to capture information on the geomagnetic dynamics from solar imaging alone. In addition, despite it being a prototypical model, our model is more accurate in most situations than three empirical baseline models. However, the model still shows some strong limitations inherent to its structure and the used data set, which could be the focus of future works. This opens the way to a better mid-to-long term data-driven magnetospheric modeling within space weather and geophysical pipelines.
更多查看译文
关键词
deep learning, solar imaging, geomagnetic activity, probabilistic forecast
AI 理解论文
溯源树
样例
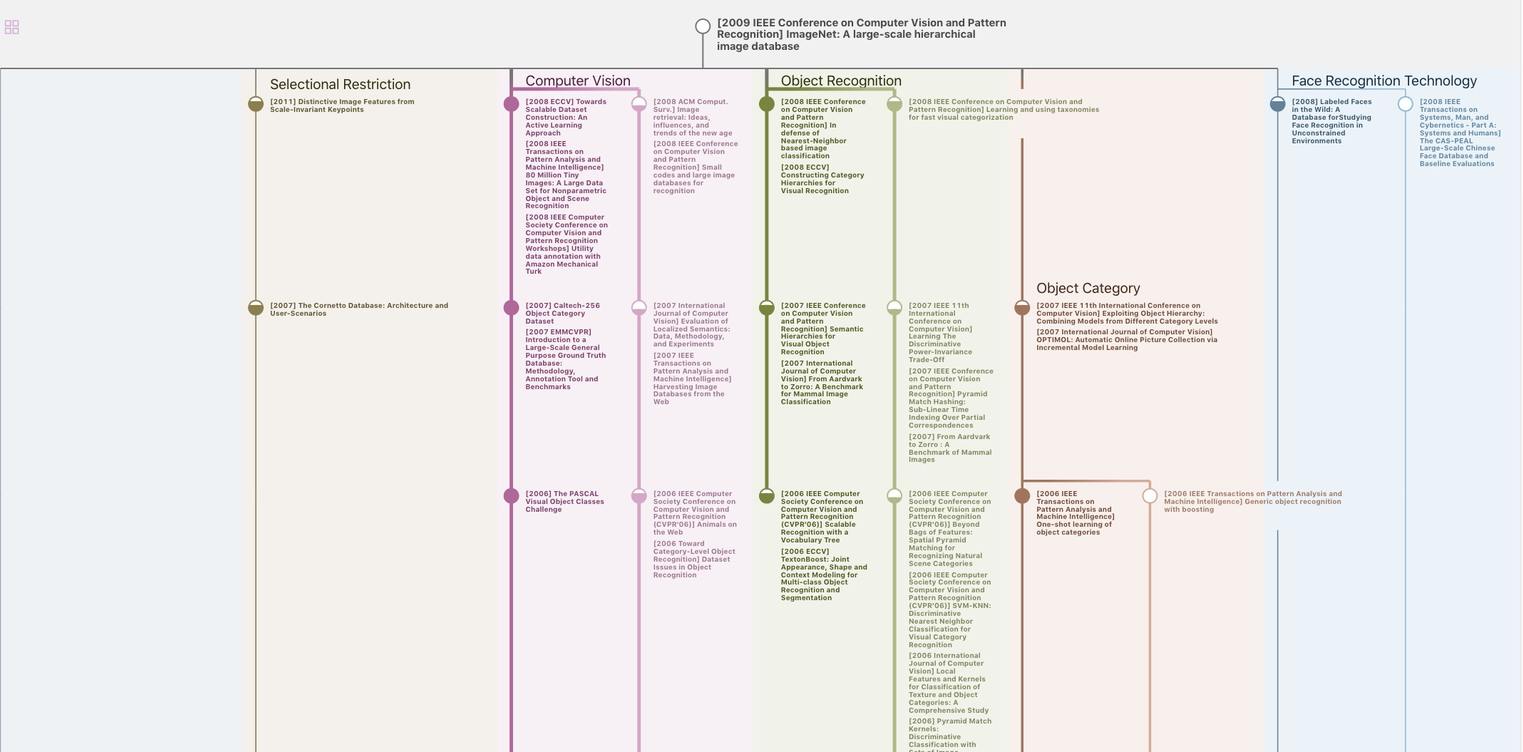
生成溯源树,研究论文发展脉络
Chat Paper
正在生成论文摘要