Exploring the combination of self and mutual teaching for tabular-data-related semi-supervised regression
EXPERT SYSTEMS WITH APPLICATIONS(2023)
摘要
Semi-supervised learning (SSL) has drawn much attention since it can alleviate the predicament in which only limited labels can be accessed, with the help of numerous unlabeled data. Many deep neural networks (NNs) based SSL methods have been proposed recently, which mostly focus on image classification tasks, while fewer efforts have been taken to tabular data related regression scenarios. In this paper, to handle the semi-supervised regression problem for tabular data with NNs based methods, we empirically observe the demerits of previous methods and present a novel framework, to explore the combination of self teaching and mutual teaching. For one thing, self teaching is employed to each base learner by using consistency regularization so that the model is driven to be more stable and also robust to local perturbations. For another, the knowledge of other base learners is extracted and filtered to perform mutual teaching to avoid the confirmation bias problem and boost the training of each base learner. Here, we employ an uncertainty-based strategy for the filtering of knowledge in mutual teaching. Extensive experiments are performed on multiple real-world datasets to demonstrate the effectiveness of previous methods and the proposed framework, and further analyses are conducted to understand the influence of each factor in the proposed framework.
更多查看译文
关键词
Semi-supervised regression, Self teaching, Mutual teaching, Tabular data
AI 理解论文
溯源树
样例
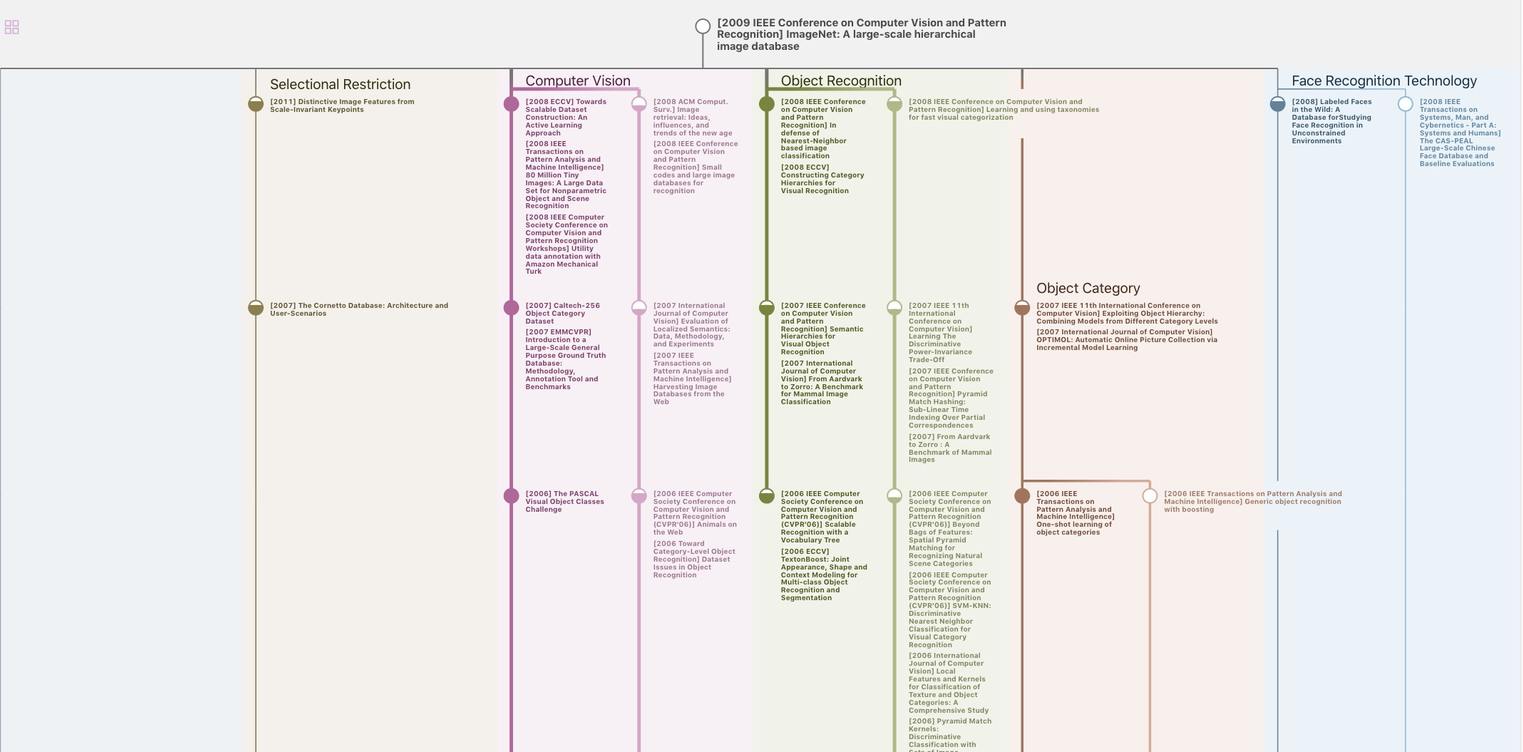
生成溯源树,研究论文发展脉络
Chat Paper
正在生成论文摘要