Event-triggered-based online integral reinforcement learning for optimal control of unknown constrained nonlinear systems
INTERNATIONAL JOURNAL OF CONTROL(2024)
摘要
For unknown nonlinear systems with actuator saturation, an online policy iteration-based algorithm is employed to solve the optimal event-triggered control problem. To learn the system dynamics, a novel identifier is proposed to make the estimation error converge quickly and the experience replay technique is employed to release the persistence of the excitation condition. To approximate the cost function and the event-triggered control law, we present event-triggered-based critic and actor networks, whose weights are updated only at triggered instants. During the policy iteration process, an event-triggered-based integral reinforcement learning method is proposed to solve the Hamilton-Jacobi-Bellman equation. By utilising the integral reinforcement learning, the network resource is saved and learning efficiency is improved. Based on the Lyapunov method, stability for the closed-loop system and estimation errors for the three networks are analysed. At last, simulation results of two numerical examples are used to show the effectiveness of the proposed method.
更多查看译文
关键词
Optimal event-triggered control,event-triggered-based integral reinforcement learning,unknown nonlinear systems,constrained control input
AI 理解论文
溯源树
样例
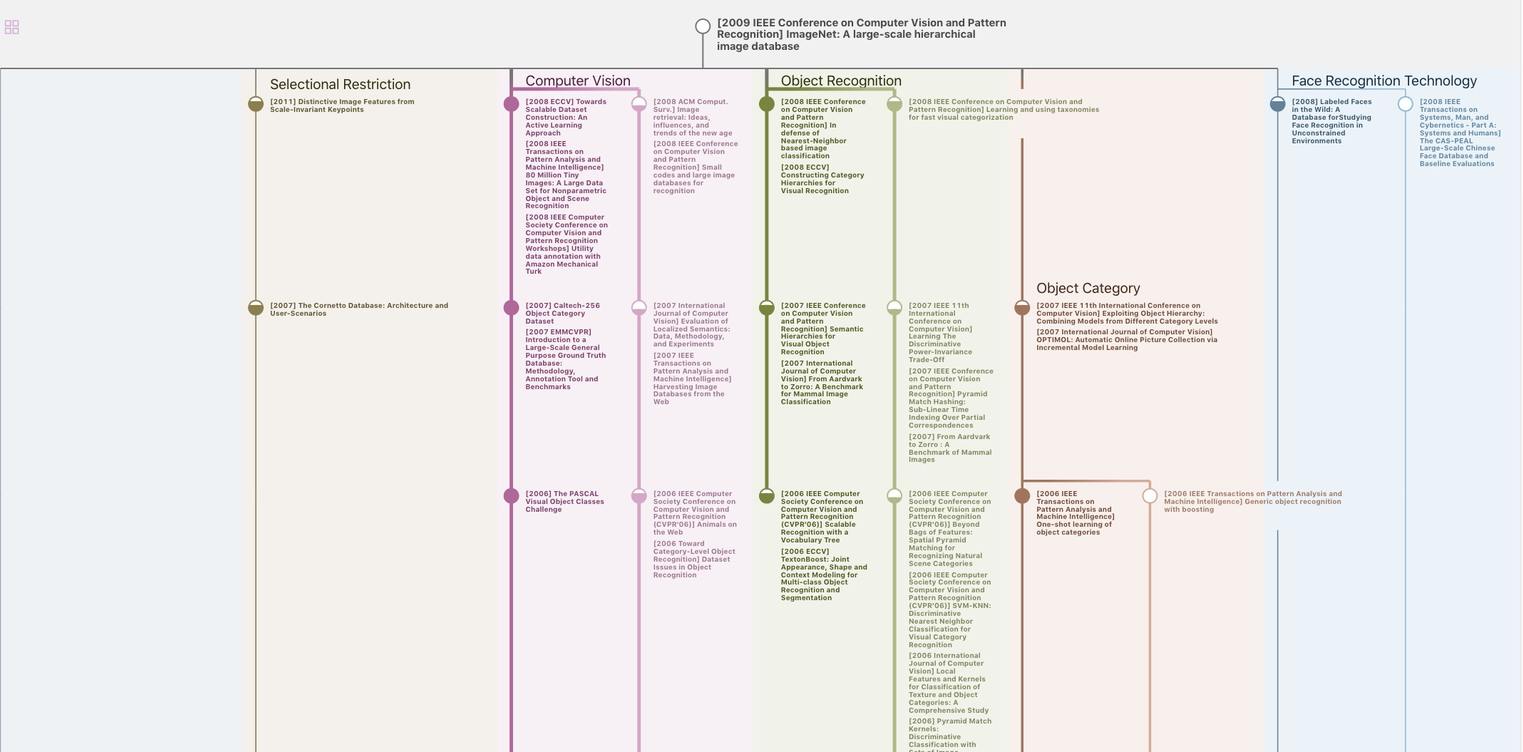
生成溯源树,研究论文发展脉络
Chat Paper
正在生成论文摘要