Tool wear recognition and signal labeling with small cross-labeled samples in impeller machining
INTERNATIONAL JOURNAL OF ADVANCED MANUFACTURING TECHNOLOGY(2022)
摘要
Data-driven deep learning method is the main way to study the condition monitoring of mechanical equipment, in which sufficient labeled signals to train the model parameters is a typical problem. The existing methods to obtain the labeled signals mainly focus on manual marking. For the non-batch impeller processing with variable working conditions, manually marking signals is not the wisest move. To solve this problem, this manuscript puts forward a deep conditional random field neural network (CRFNN) method. This framework fully utilizes the sensitivity of the conditional probability model to adjacent data marker information, and small cross-labeled samples are used to predict the labels of unknown signals. At the same time, the variational autoencoder is used to convert the one-dimensional time series signal into a three-dimensional image, which solves the problem that the empty tool signals have a great impact on the tool wear condition monitoring in the process of impeller blade machining. Experimental results on a CNC machining center demonstrate the effectiveness and feasibility of the proposed method and outperform the existing works under industrial small labeled samples.
更多查看译文
关键词
Deep conditional random field neural network,Signal labeling,Small cross-labeled samples,Tool wear recognition
AI 理解论文
溯源树
样例
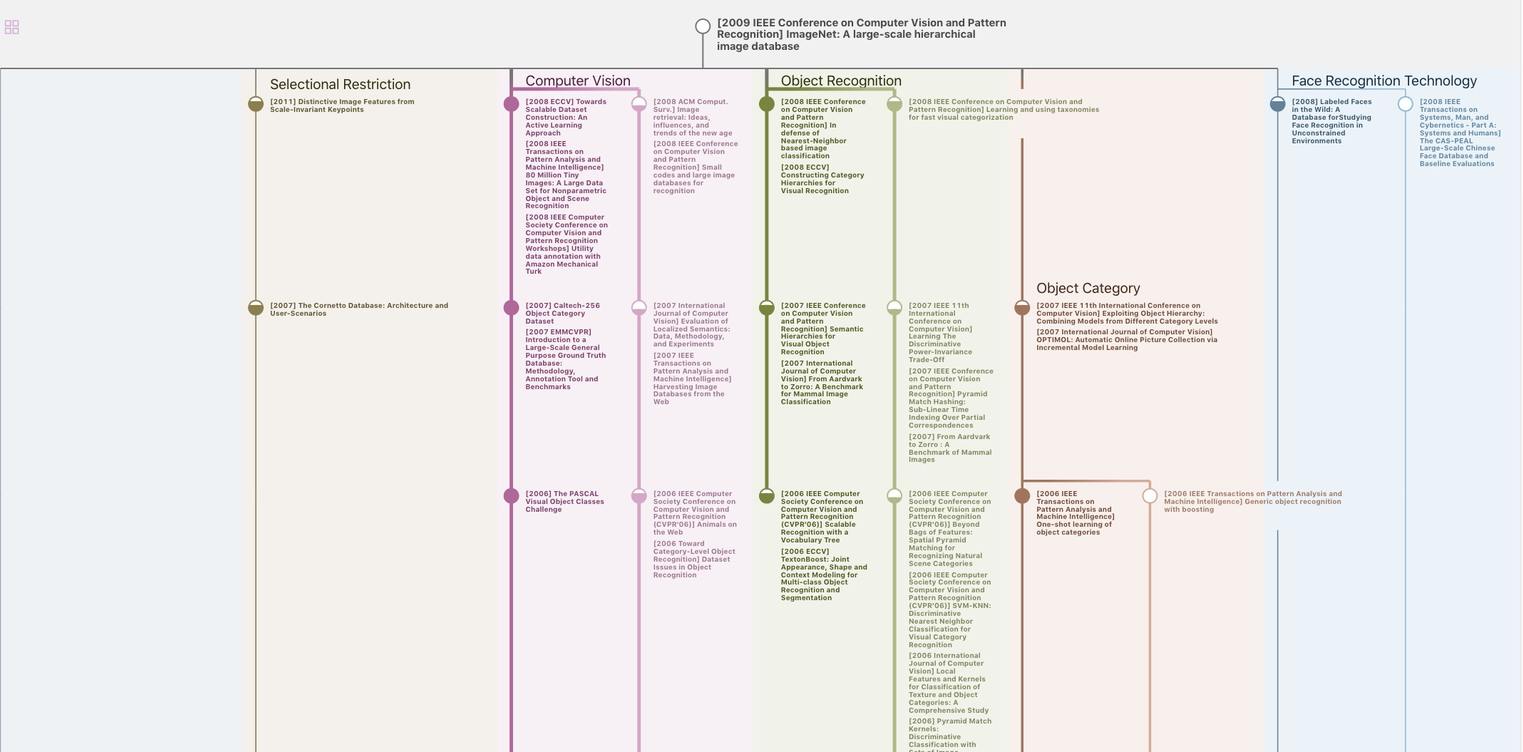
生成溯源树,研究论文发展脉络
Chat Paper
正在生成论文摘要