Dynamic and Interpretable State Representation for Deep Reinforcement Learning in Automated Driving
IFAC PAPERSONLINE(2022)
摘要
Understanding the causal relationship between an autonomous vehicle's input state and its output action is important for safety mitigation and explainable automated driving. However, reinforcement learning approaches have the drawback of being black box models. This work proposes an interpretable state representation that can capture state-action causalities for an automated driving agent, while also allowing for the underlying formulation to be general enough to be adapted to different driving scenarios. It also proposes encoding temporally-extended information in the state representation for better driving performance. We test this approach on a reinforcement learning agent in a highway simulation environment and demonstrate that the proposed state representation can capture state-action causalities in an interpretable manner. Experimental results show that the formulation and interpretation can be used to adapt the behavior of the driving agent to achieve desired, even unseen, driving behaviors after training. Copyright (c) 2022 The Authors. This is an open access article under the CC BY-NC-ND license
更多查看译文
关键词
autonomous vehicles,reinforcement learning control,state representation,interpretability,generalization,deep learning
AI 理解论文
溯源树
样例
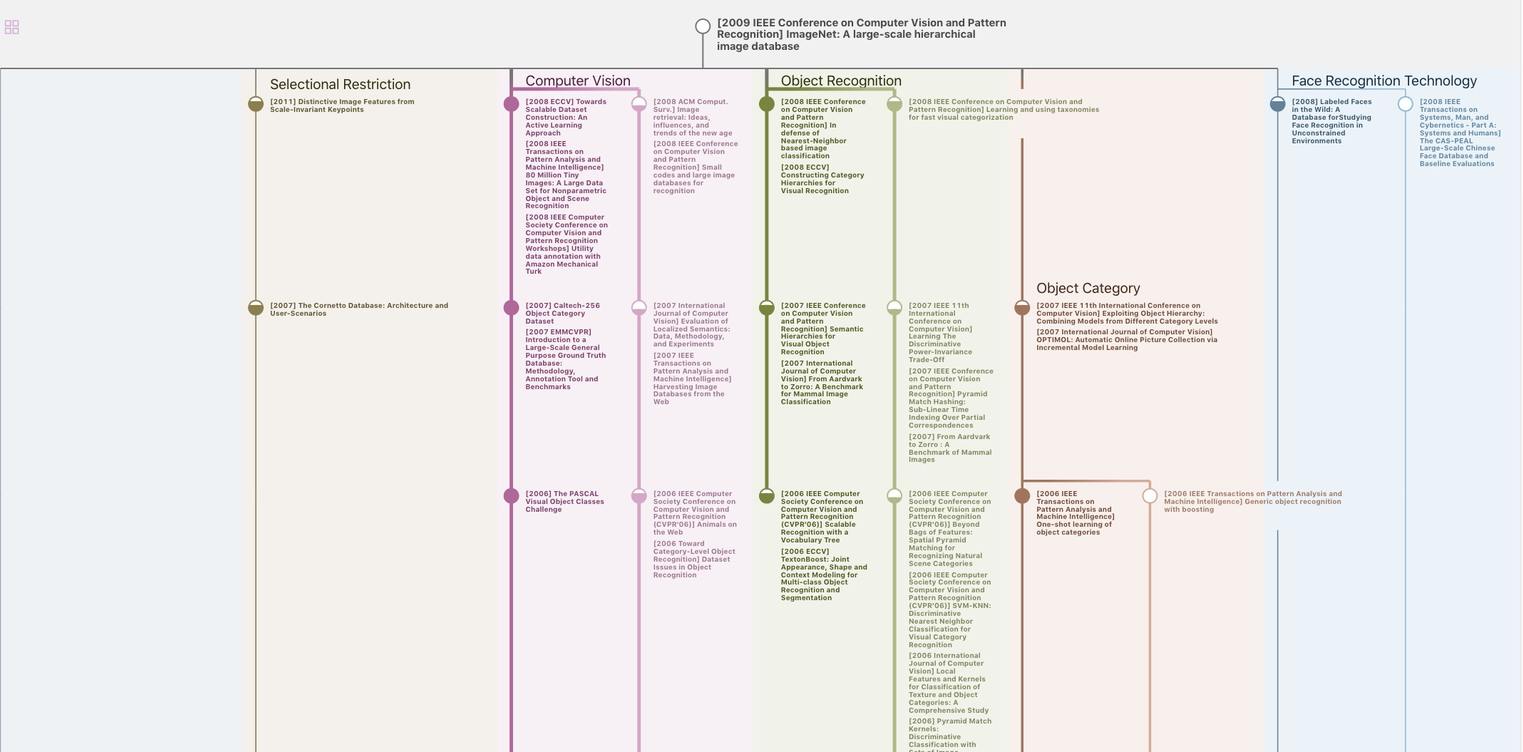
生成溯源树,研究论文发展脉络
Chat Paper
正在生成论文摘要