Time Series Recovery Using Adjacent Channel Data Based on LSTM: A Case Study of Subway Vibrations
APPLIED SCIENCES-BASEL(2022)
摘要
Multi-sensor technology has been widely applied in the condition monitoring of rail transit. In practice, the data of some channels in the high channel counts are often abnormal or lost due to the abnormality and damage of the sensors, thus resulting in a large amount of data waste. A method for the data recovery of lost channels by using adjacent channel data is proposed to solve this problem. Based on the LSTM network algorithm, a data recovery model is established based on the "sequence-to-sequence" regression analysis of adjacent channel data. Taking the measured vibration data of a subway as an example, the network is trained with multi-channel measured data to recover the lost channel data of time-series characteristics. The results show that this multi-channel data recovery model is feasible, and the accuracy is up to 98%. This method can also further reduce the number of channels that need to be collected.
更多查看译文
关键词
multi-channel data, time-series recovery, neural network, regression analysis, data recovery, time domain, frequency domain
AI 理解论文
溯源树
样例
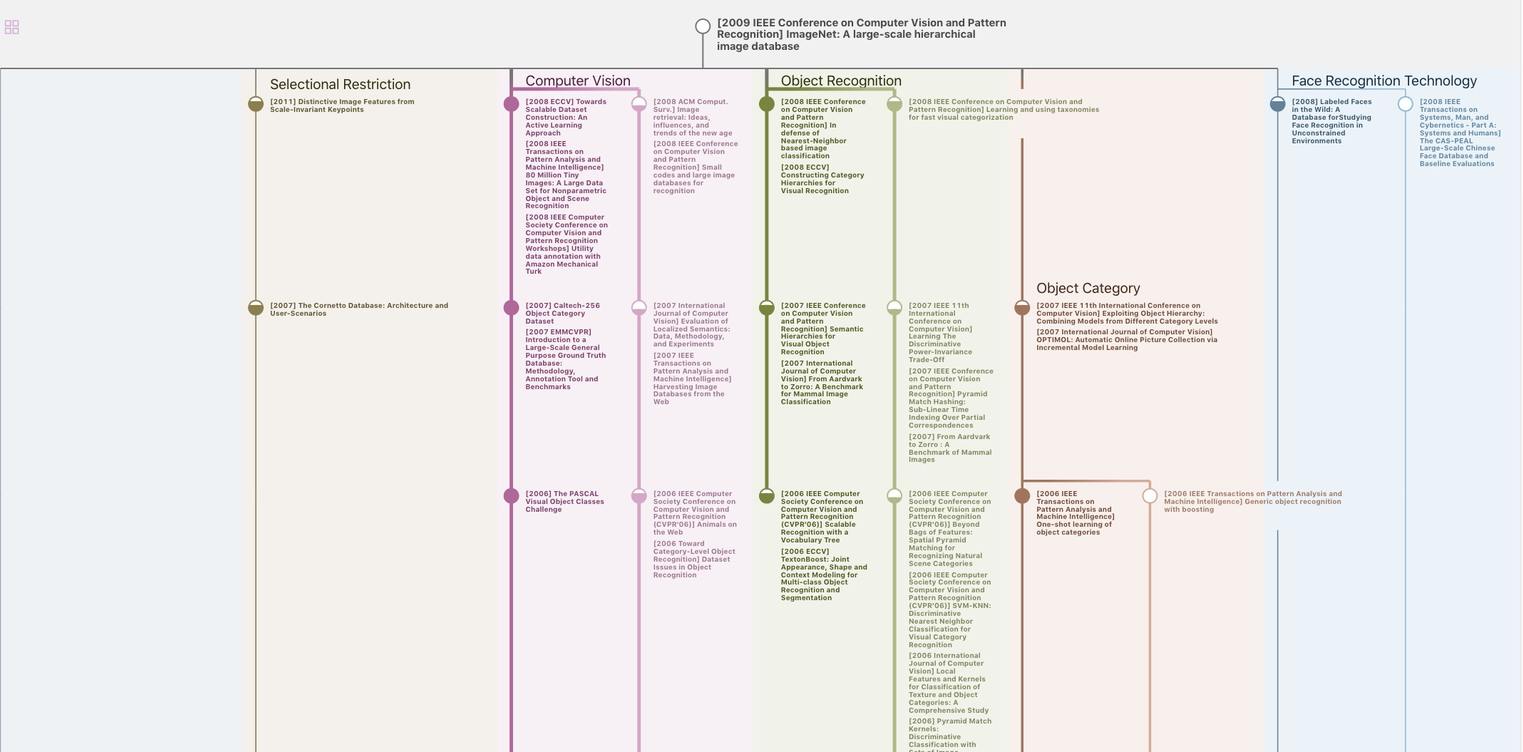
生成溯源树,研究论文发展脉络
Chat Paper
正在生成论文摘要