FPT: Fine-Grained Detection of Driver Distraction Based on the Feature Pyramid Vision Transformer
IEEE Transactions on Intelligent Transportation Systems(2023)
摘要
According to the surveys of the World Health Organization, distracted driving is one of main causes of road traffic accidents. To improve road traffic safety, real-time detection of drivers’ driving behavior is very important for the development of highly reliable Advanced Driver Assistance System (ADAS). At present, the deep learning architecture based on a Convolutional Neural Network (CNN) has disadvantages such as large number of parameters and weak global feature extraction ability. Therefore, this paper proposes an innovative driver distraction detection model based on the fusion of a transformer and a CNN, referred to as FPT, which is the first exploration in the field of driver distraction detection. First, we introduce the latest Twins transformer as a benchmark. Then, we design residual embedding to replace block embedding, which can further integrate the convolutional neural network with Transformer and improve the feature extraction ability. In addition, the Multilayer Perceptron (MLP) module with a large parameter occupancy rate in the original transformer structure is replaced with a lightweight group convolution module to reduce computational complexity. Finally, a cross-entropy loss function for label smoothing is designed to guide network learning with significantly differentiated features. Comparison results on two large-scale driver distraction detection datasets show that the proposed FPT offers a better compromise between computational cost and performance compared to the state-of-the-art CNN and Transformer architectures.
更多查看译文
关键词
Vision transformer,distraction detection,deep learning,residual embedding,driving safety
AI 理解论文
溯源树
样例
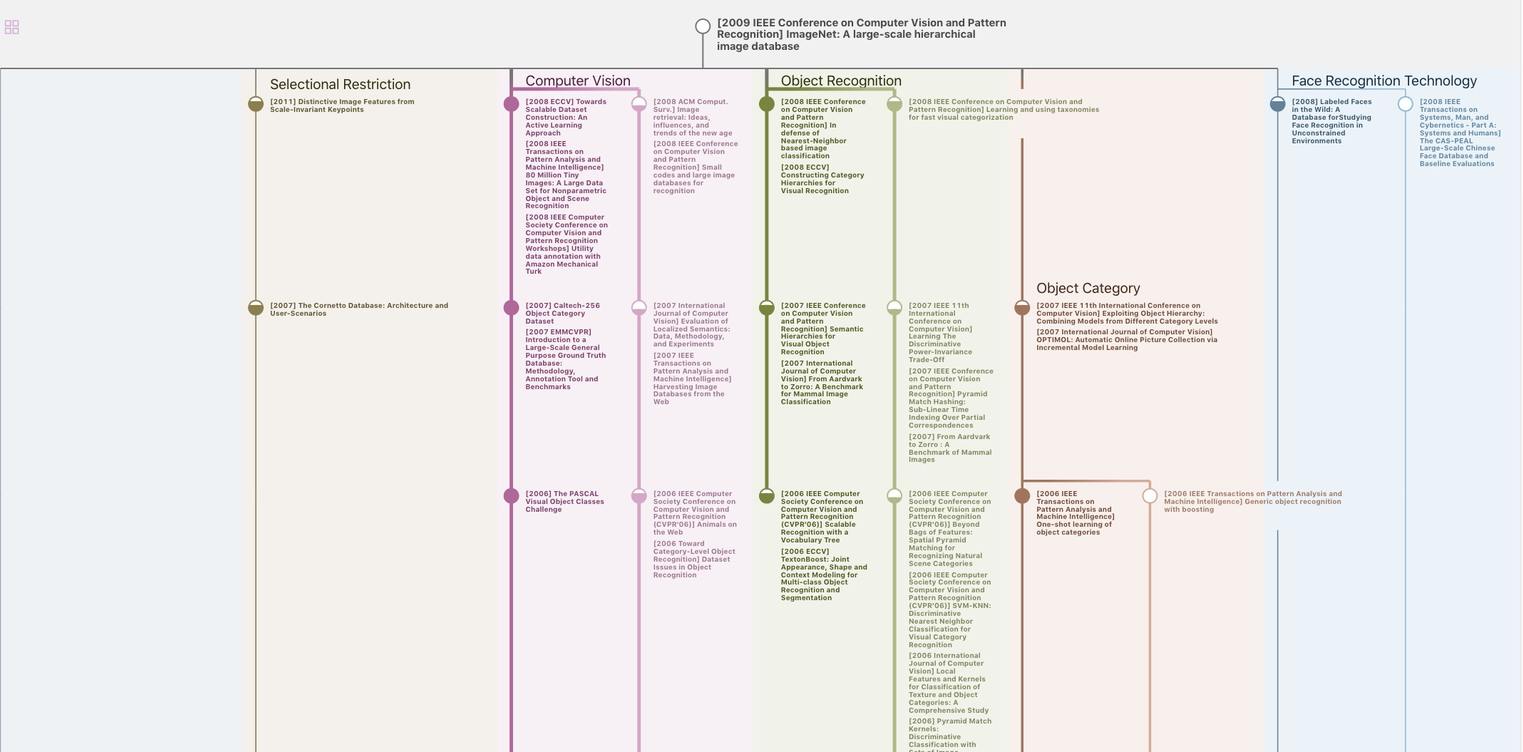
生成溯源树,研究论文发展脉络
Chat Paper
正在生成论文摘要