Spatial-Temporal Position-Aware Graph Convolution Networks for Traffic Flow Forecasting
IEEE TRANSACTIONS ON INTELLIGENT TRANSPORTATION SYSTEMS(2023)
摘要
Recent works demonstrate that capturing correlations between road network nodes is crucial to improving traffic flow forecasting accuracy. In general, there are spatial, temporal, and joint spatial-temporal correlations between two nodes, whose strength is related to spatial and temporal position factors. For example, traffic congestion that occurs at a traffic hub has a wider and stronger impact than that at a branch road. Moreover, the above impacts can vary with temporal position. Although spatial-temporal graph convolution networks have become a popular paradigm for modeling those correlations, there are still three problems with existing models: (i) failing to effectively model joint spatial-temporal correlations; (ii) ignoring spatial and temporal position factors when modeling the aforementioned correlations; and (iii) failing to capture distinct spatial-temporal patterns of each node. To cope with the above issues, this paper proposes a novel
S
patial-
T
emporal
P
osition-aware
G
raph
C
onvolution
N
etwork (STPGCN) for traffic flow forecasting. Specifically, a trainable embedding module is constructed to represent the spatial and temporal positions of the nodes. Subsequently, a spatial-temporal position-aware relation inference module is proposed to adaptively infer the correlation weights of the three important spatial-temporal relations. Based on this, the generated spatial-temporal relations are integrated into a graph convolution layer for aggregating and updating node features. Finally, we design a spatial-temporal position-aware gated activation unit in the graph convolution, to capture the node-specific pattern features under the guidance of position embedding. Extensive experiments on six real-world datasets demonstrate the superiority of our model in terms of prediction performance and computational efficiency.
更多查看译文
关键词
Correlation, Convolution, Forecasting, Predictive models, Feature extraction, Roads, Data models, Traffic flow forecasting, spatial-temporal correlations, spatial-temporal position, graph convolution networks
AI 理解论文
溯源树
样例
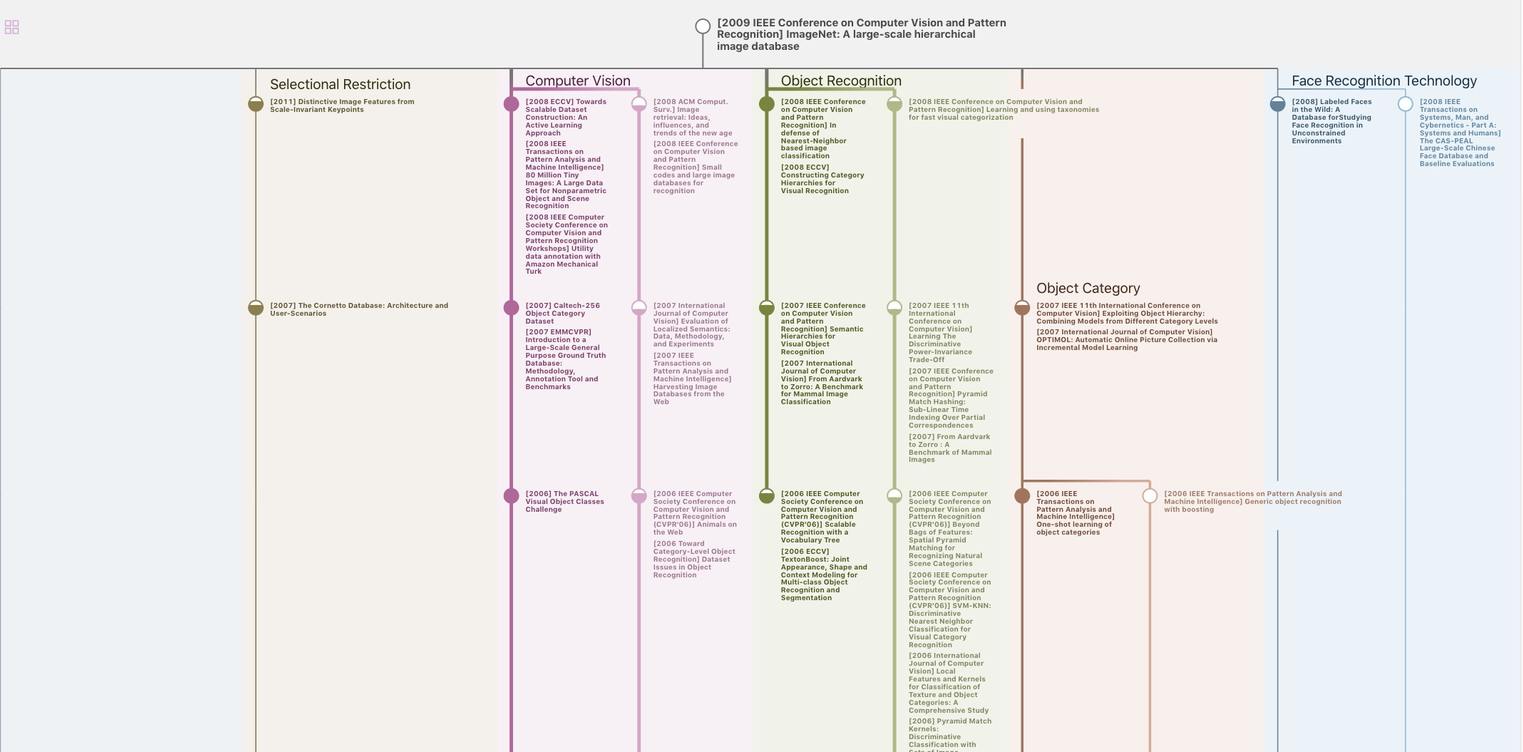
生成溯源树,研究论文发展脉络
Chat Paper
正在生成论文摘要