A 4 μ W Low-Power Audio Processor System for Real-Time Jaw Movements Recognition in Grazing Cattle
Journal of Signal Processing Systems(2022)
摘要
Precision livestock farming consists of technological tools and techniques to improve livestock management. Proper detection and classification of jaw movement (JM) events are indispensable for the estimation of dry matter intake, detection of health problems, and flag the onset of estrus, among other information. The analysis of acoustic signals is one of the most accepted ways to monitor the feeding behavior of free-grazing cattle. Different acoustic methods have been developed for recognizing JM-events in recent years. However, their operation is limited to off-line analysis on a personal computer. The lack of on-line acoustic monitoring systems is associated with the challenging operation requirements (low-power consumption, autonomy, portability, robustness and non-intrusive on the animal). In this paper, a fixed-point variant of the chew-bite energy-based algorithm is presented. This algorithm is implemented on a new low-power audio processor system for real-time recognition of JM-events. The system includes a Nanocontroller processor, which is always-on and detects JM-events; and a second transport-triggered architecture (TTA) based processor, which is mainly in power-down and classifies JM-events. The results demonstrate that the proposed fixed-point JM-events recognizer achieves a recognition rate of 91.4% and 90.2% in noiseless and noisy conditions, respectively. The recognition rate increases by 6.1% regarding a previous reference on-line system. Moreover, the proposed audio processor system chip consumes 4 μW on average, i.e., only 2.3% of the power of an always-on TTA-based processor system for the same audio sequence. An exemplary implementation of the proposed system in a 65 nm low-leakage CMOS technology is given.
更多查看译文
关键词
Acoustic monitoring, Machine learning, Transport-triggered architecture, Nanocontroller, Pattern recognition, Processor architecture
AI 理解论文
溯源树
样例
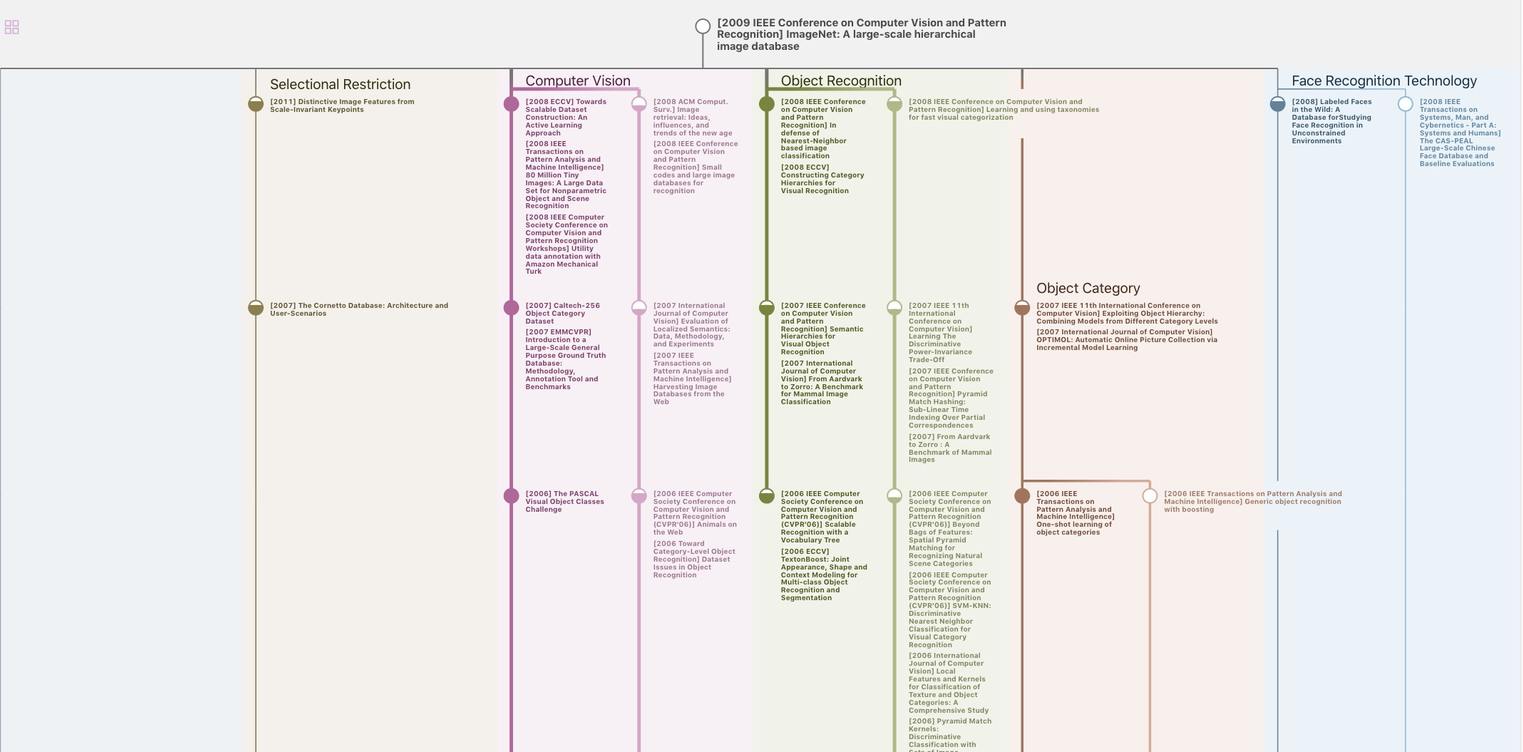
生成溯源树,研究论文发展脉络
Chat Paper
正在生成论文摘要