Deep and shallow fast embedded capsule networks: going faster with capsules
ANALOG INTEGRATED CIRCUITS AND SIGNAL PROCESSING(2022)
摘要
Capsule Networks (CapsNets) is a great approach for understanding data in the field of computer vision. CapsNets allow a deeper understanding of images compared to the traditional Convolutional Neural Networks. The first test for CapsNet was in digits recognition on the ‘MNIST’ dataset, where it successfully achieved high accuracy. CapsNets are reliable at deciphering overlapping digits. Deep Capsule Networks achieved state-of-the-art accuracy in CIFAR10 which isn’t achieved by shallow capsule networks. Despite all these accomplishments, Deep Capsule Networks are very slow due to the ‘Dynamic Routing’ algorithm. In this paper, Fast Embedded Capsule Network and Deep Fast Embedded Capsule Network are introduced, representing novel capsule network architectures that uses 1D convolution based dynamic routing with a fast element-wise multiplication transformation process. These architectures not only compete with the state-of-the-art methods in terms of accuracy in the capsule domain, but also excels in terms of speed, and reduced complexity. This is shown by the 58% reduction in the number of trainable parameters and 64% reduction in the average epoch time in the training process. Experimental results shows excellent and verified properties.
更多查看译文
关键词
1D convolutional kernels,CapsNets,Fashion MNIST,CIFAR10,Facial expressions recognition,MNIST,CK+
AI 理解论文
溯源树
样例
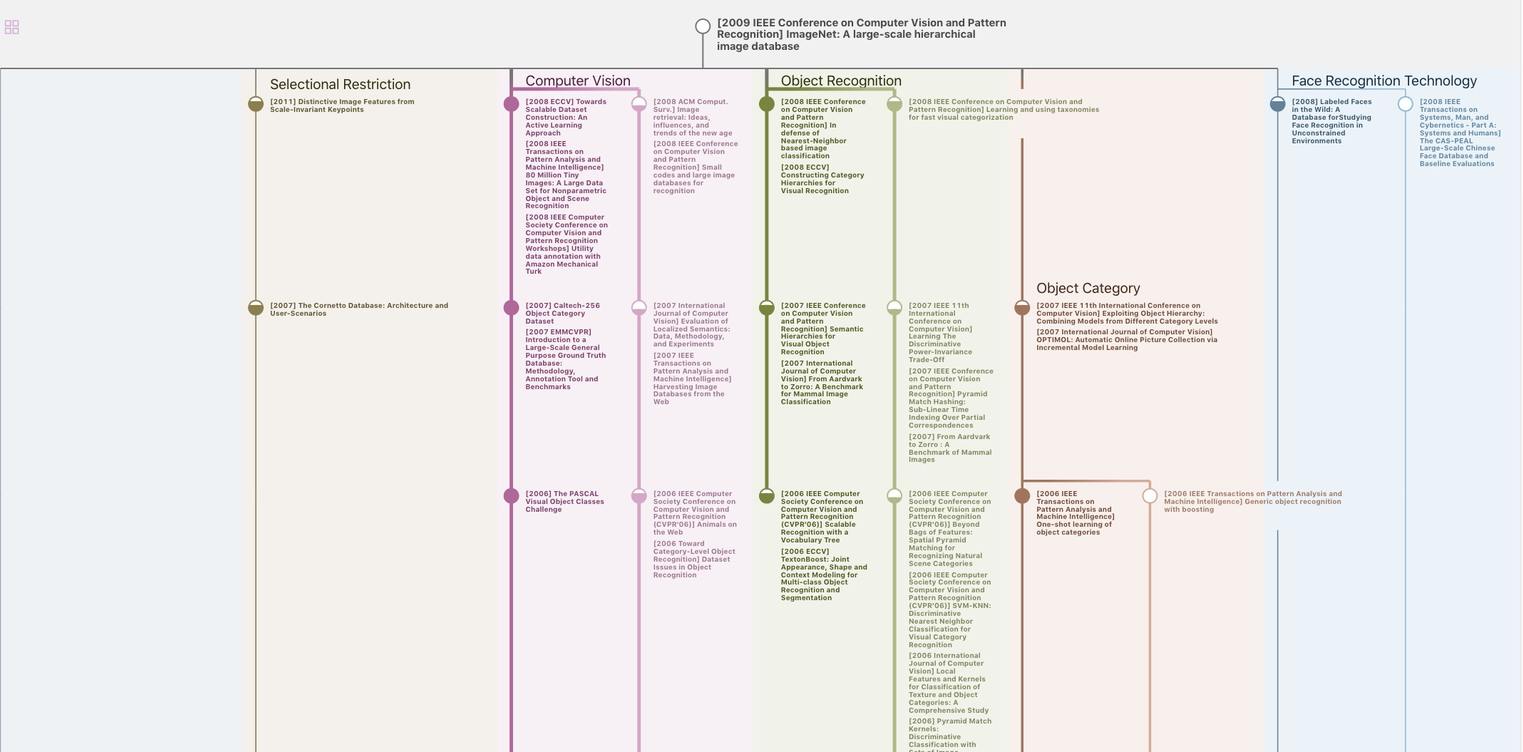
生成溯源树,研究论文发展脉络
Chat Paper
正在生成论文摘要