P ^2 CG: a privacy preserving collaborative graph neural network training framework
VLDB JOURNAL(2022)
摘要
Graph neural networks (GNNs) and their variants have generalized deep learning methods into non-Euclidean graph data, bringing substantial improvement in many graph mining tasks. In practice, the large graph could be isolated by different databases. Recently, user privacy protection has become a crucial concern in practical machine learning, which motivates us to explore a GNN framework with data sharing and without violating user privacy leakage in the meanwhile. However, it is challenging to scale GNN training to edge partitioned distributed graph databases while preserving data privacy and model quality. In this paper, we propose a privacy preserving collaborative GNN training framework, P ^2 CG, aiming to obtain competitive model performance as the centralized setting. We present the clustering-based differential privacy algorithm to reduce the model degradation caused by the noisy edges generation. Moreover, we propose a novel interaction-based secure multi-layer graph convolution algorithm to alleviate the noise diffusion problem. Experimental results on the benchmark datasets and the production dataset in Tencent Inc. show that P ^2 CG can significantly increase the model performance and obtain competitive results as a centralized setting.
更多查看译文
关键词
Graph neural networks, Data privacy protection, Differential privacy, Edge partitioned distributed graph database
AI 理解论文
溯源树
样例
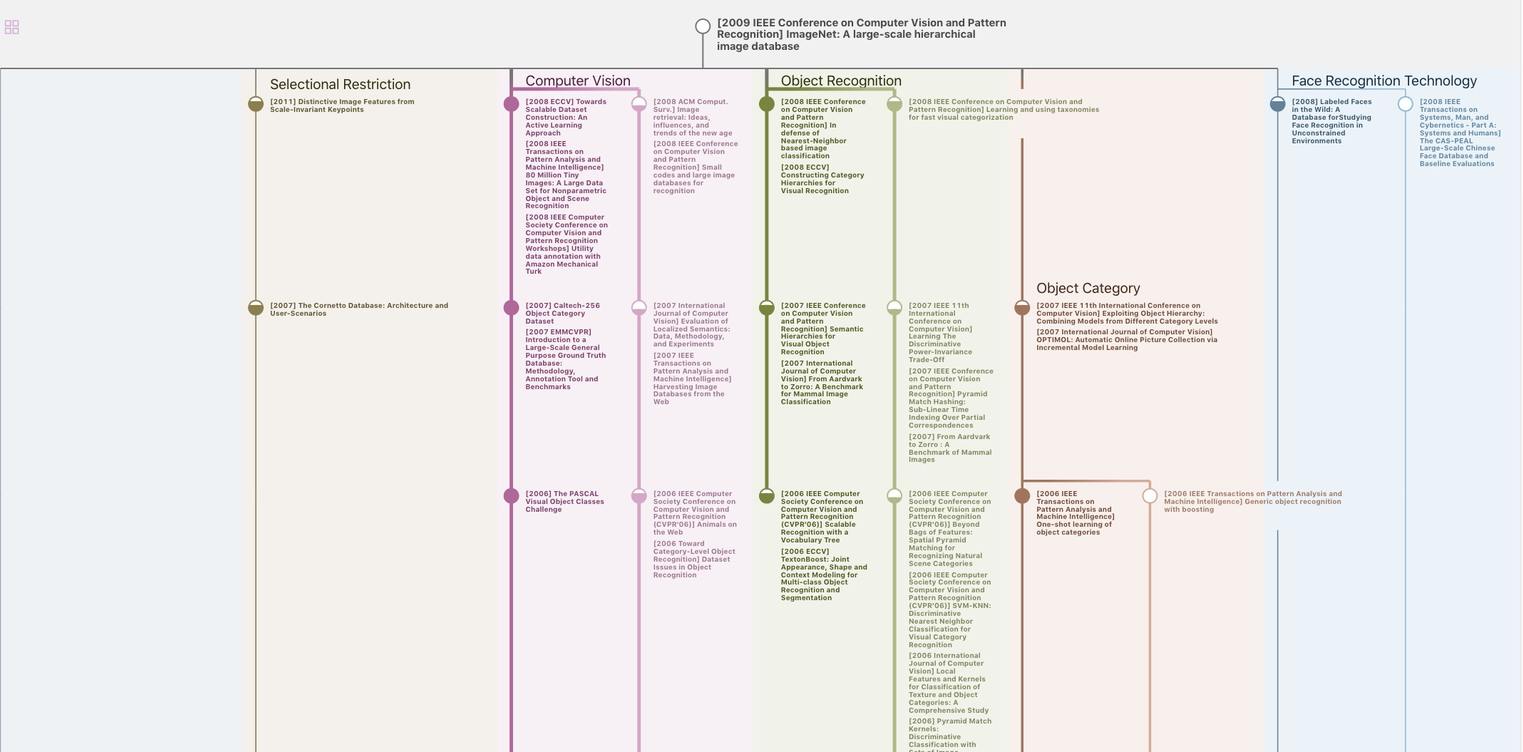
生成溯源树,研究论文发展脉络
Chat Paper
正在生成论文摘要